Automating Control of Overestimation Bias for Continuous Reinforcement Learning
arxiv(2021)
摘要
Bias correction techniques are used by most of the high-performing methods for off-policy reinforcement learning. However, these techniques rely on a pre-defined bias correction policy that is either not flexible enough or requires environment-specific tuning of hyperparameters. In this work, we present a simple data-driven approach for guiding bias correction. We demonstrate its effectiveness on the Truncated Quantile Critics -- a state-of-the-art continuous control algorithm. The proposed technique can adjust the bias correction across environments automatically. As a result, it eliminates the need for an extensive hyperparameter search, significantly reducing the actual number of interactions and computation.
更多查看译文
关键词
continuous reinforcement learning,overestimation bias,reinforcement learning
AI 理解论文
溯源树
样例
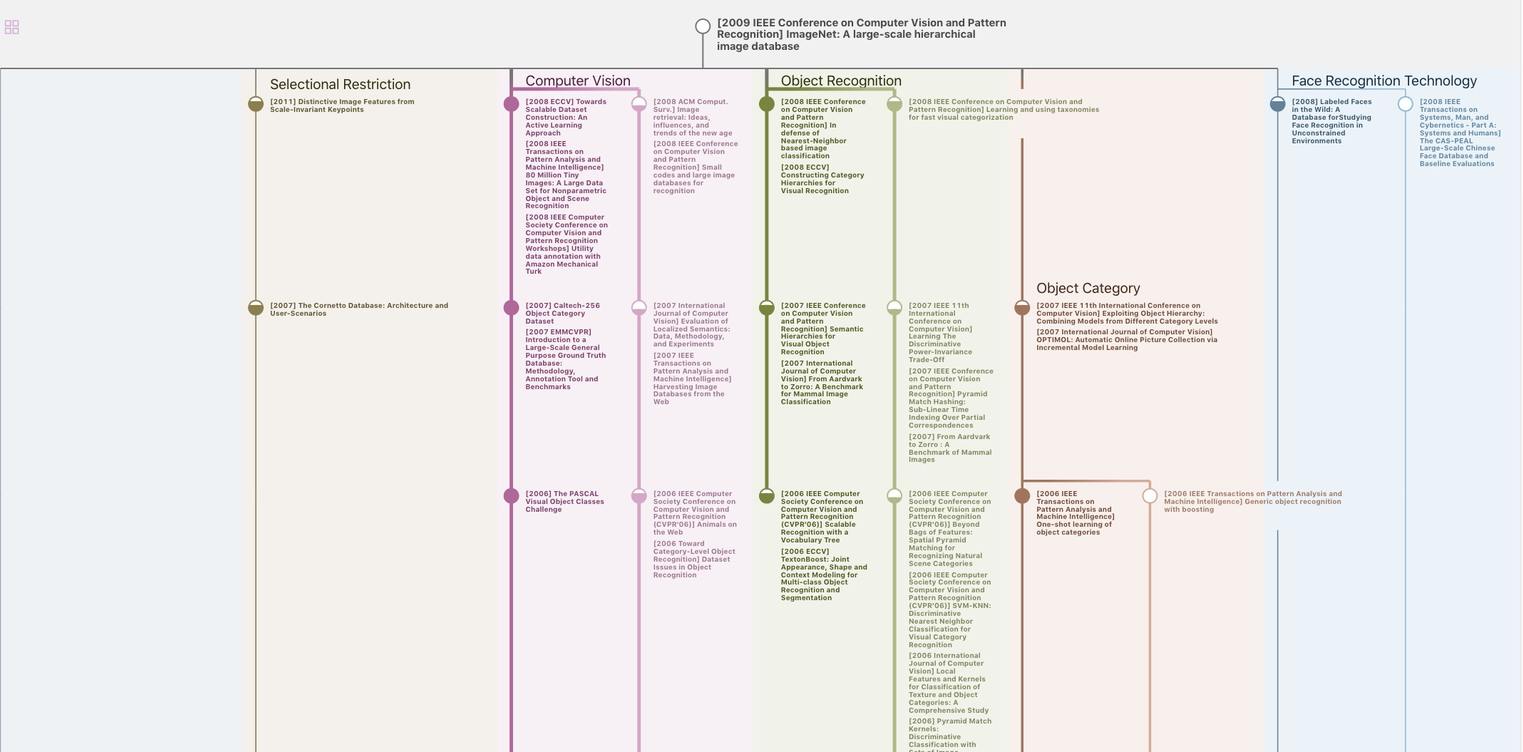
生成溯源树,研究论文发展脉络
Chat Paper
正在生成论文摘要