Causal Effect Estimation using Variational Information Bottleneck
arxiv(2022)
摘要
Causal inference is to estimate the causal effect in a causal relationship when intervention is applied. Precisely, in a causal model with binary interventions, i.e., control and treatment, the causal effect is simply the difference between the factual and counterfactual. The difficulty is that the counterfactual may never been obtained which has to be estimated and so the causal effect could only be an estimate. The key challenge for estimating the counterfactual is to identify confounders which effect both outcomes and treatments. A typical approach is to formulate causal inference as a supervised learning problem and so counterfactual could be predicted. Including linear regression and deep learning models, recent machine learning methods have been adapted to causal inference. In this paper, we propose a method to estimate Causal Effect by using Variational Information Bottleneck (CEVIB). The promising point is that VIB is able to naturally distill confounding variables from the data, which enables estimating causal effect by using observational data. We have compared CEVIB to other methods by applying them to three data sets showing that our approach achieved the best performance. We also experimentally showed the robustness of our method.
更多查看译文
关键词
estimation,information,effect
AI 理解论文
溯源树
样例
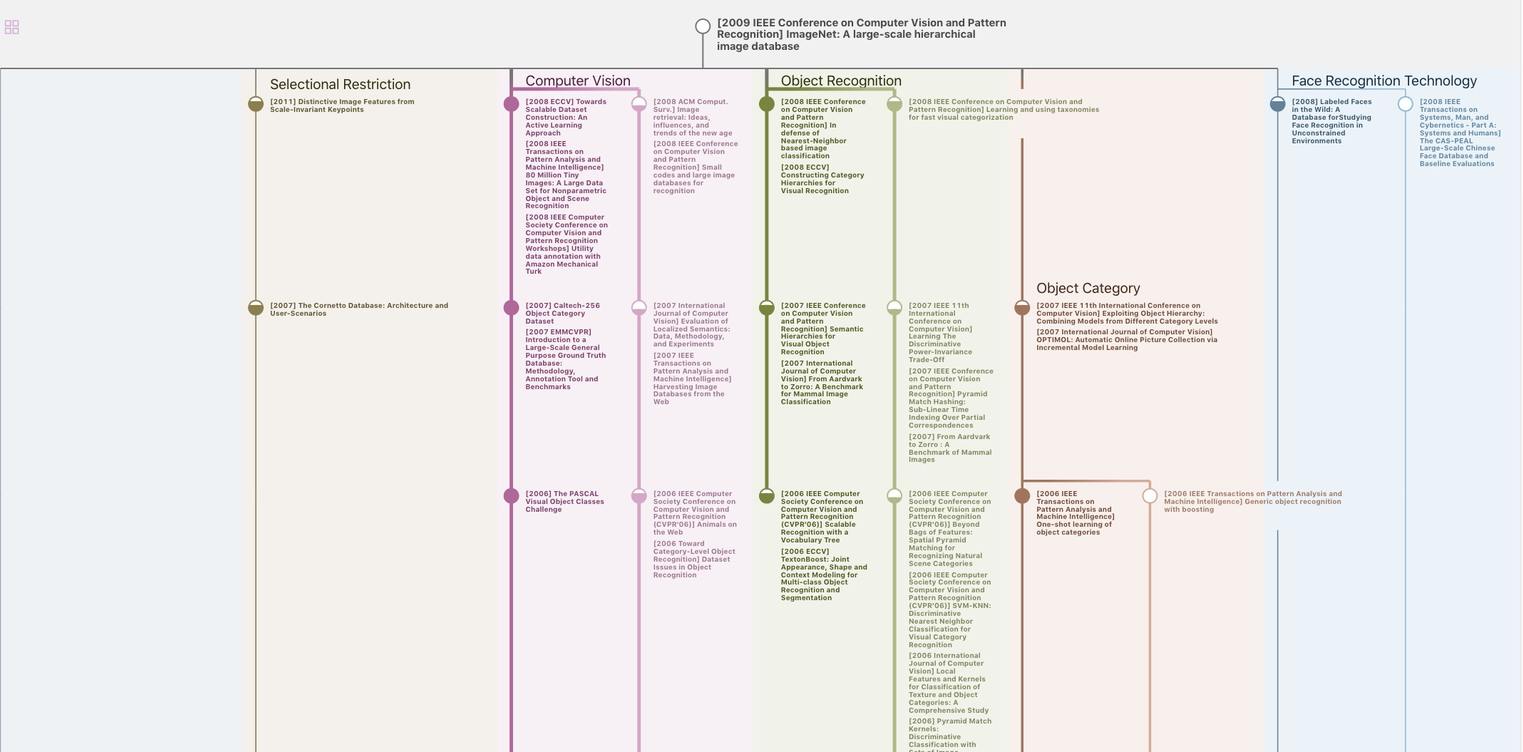
生成溯源树,研究论文发展脉络
Chat Paper
正在生成论文摘要