Average-Reward Learning and Planning with Options.
Annual Conference on Neural Information Processing Systems(2021)
摘要
We extend the options framework for temporal abstraction in reinforcement learning from discounted Markov decision processes (MDPs) to average-reward MDPs. Our contributions include general convergent off-policy inter-option learning algorithms, intra-option algorithms for learning values and models, as well as sample-based planning variants of our learning algorithms. Our algorithms and convergence proofs extend those recently developed by Wan, Naik, and Sutton. We also extend the notion of option-interrupting behaviour from the discounted to the average-reward formulation. We show the efficacy of the proposed algorithms with experiments on a continuing version of the Four-Room domain.
更多查看译文
关键词
planning,learning,average-reward
AI 理解论文
溯源树
样例
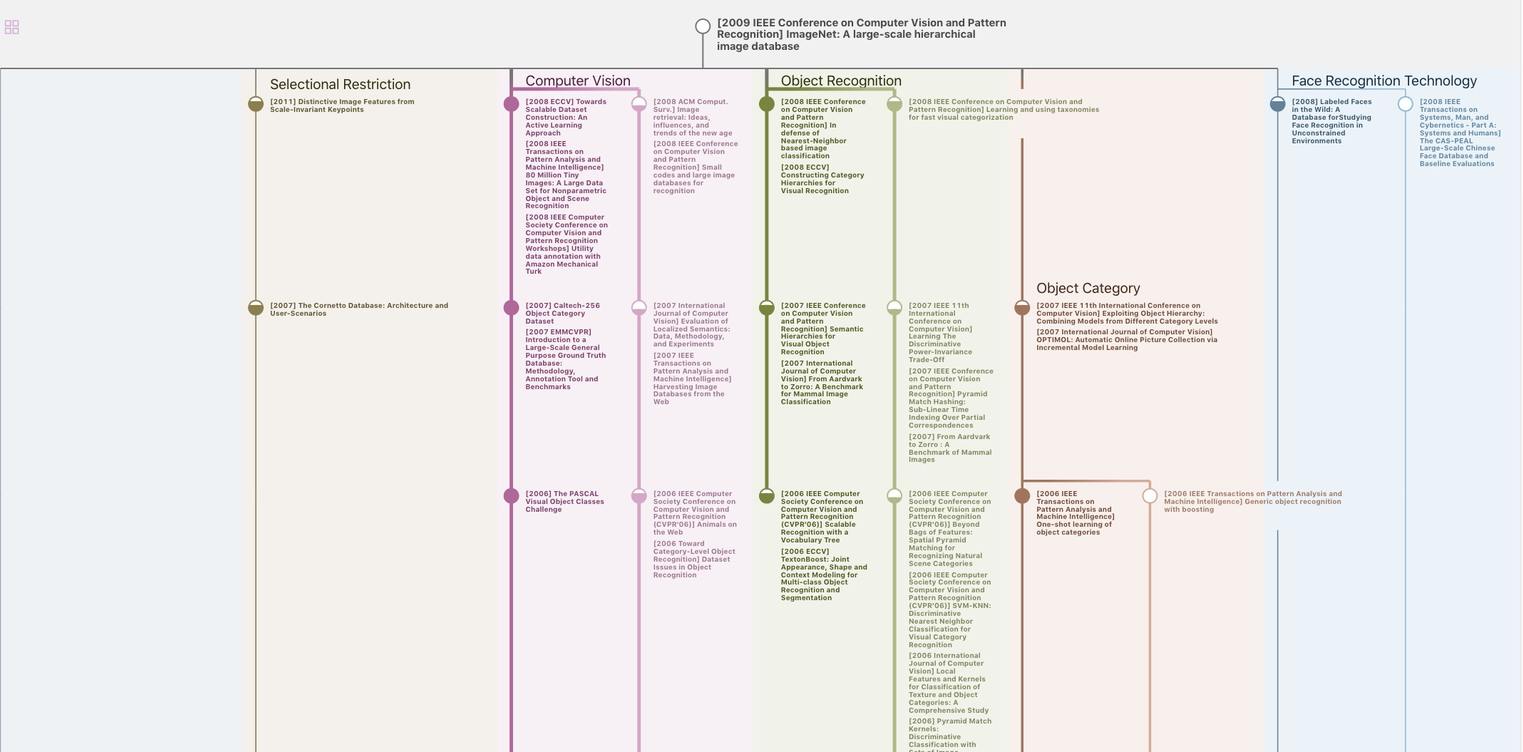
生成溯源树,研究论文发展脉络
Chat Paper
正在生成论文摘要