Robust Trajectory Planning with Parametric Uncertainties
2021 IEEE INTERNATIONAL CONFERENCE ON ROBOTICS AND AUTOMATION (ICRA 2021)(2021)
摘要
In this paper we extend the previously introduced notion of closed-loop state sensitivity by introducing the concept of input sensitivity and by showing how to exploit it in a trajectory optimization framework. This allows to generate an optimal reference trajectory for a robot that minimizes the state and input sensitivities against uncertainties in the model parameters, thus producing inherently robust motion plans. We parametrize the reference trajectories with Beziers curves and discuss how to consider linear and nonlinear constraints in the optimization process (e.g., input saturations). The whole machinery is validated via an extensive statistical campaign that clearly shows the interest of the proposed methodology.
更多查看译文
关键词
reference trajectories,Béziers curves,nonlinear constraints,optimization process,robust trajectory planning,parametric uncertainties,closed-loop state sensitivity,trajectory optimization framework,optimal reference trajectory,input sensitivities,model parameters,inherently robust motion plans,extensive statistical campaign
AI 理解论文
溯源树
样例
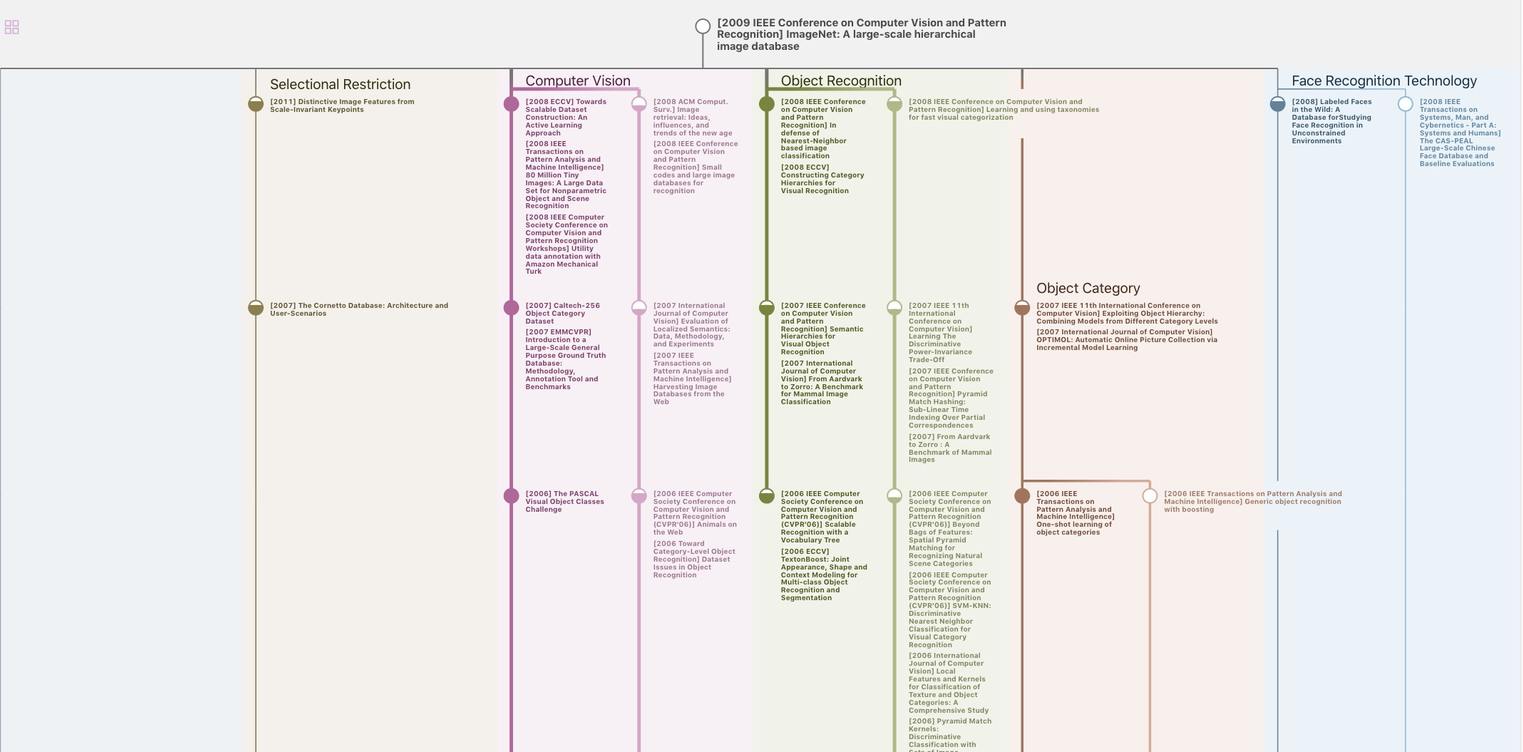
生成溯源树,研究论文发展脉络
Chat Paper
正在生成论文摘要