Multi-session Underwater Pose-graph SLAM using Inter-session Opti-acoustic Two-view Factor
2021 IEEE INTERNATIONAL CONFERENCE ON ROBOTICS AND AUTOMATION (ICRA 2021)(2021)
摘要
Concurrent mapping necessitates data association among vehicles to overcome temporal and sensor modality differences. In this work, we focus on an underwater multi-vehicle mapping scenario in which vehicles have various sensor modalities, namely sonar and camera. This inter-session sonar-optical image matching poses two main challenges. First, ensuring covisibility for the opti-acoustic pair is complex due to their projection models and field of view (FOV) difference. Second, even with secured covisible frames, feature matching over various sensor modalities is not trivial. To overcome these challenges, we complete multi-session simultaneous localization and mapping (SLAM) by introducing an opti-acoustic pairwise factor. We alleviate the covisibility requirement by introducing inter-session measurements. We achieved opti-acoustic feature matching by applying a style-transfer and integration with SuperGlue. The proposed method is validated via simulation and real underwater tank tests.
更多查看译文
关键词
inter-session opti-acoustic two-view factor,concurrent mapping,temporal modality differences,sensor modality differences,multivehicle mapping scenario,sensor modalities,inter-session sonar-optical image matching,opti-acoustic pair,opti-acoustic pairwise factor,inter-session measurements,opti-acoustic feature,multisession underwater pose-graph SLAM,data association,field of view difference,FOV difference,FOV difference,SuperGlue
AI 理解论文
溯源树
样例
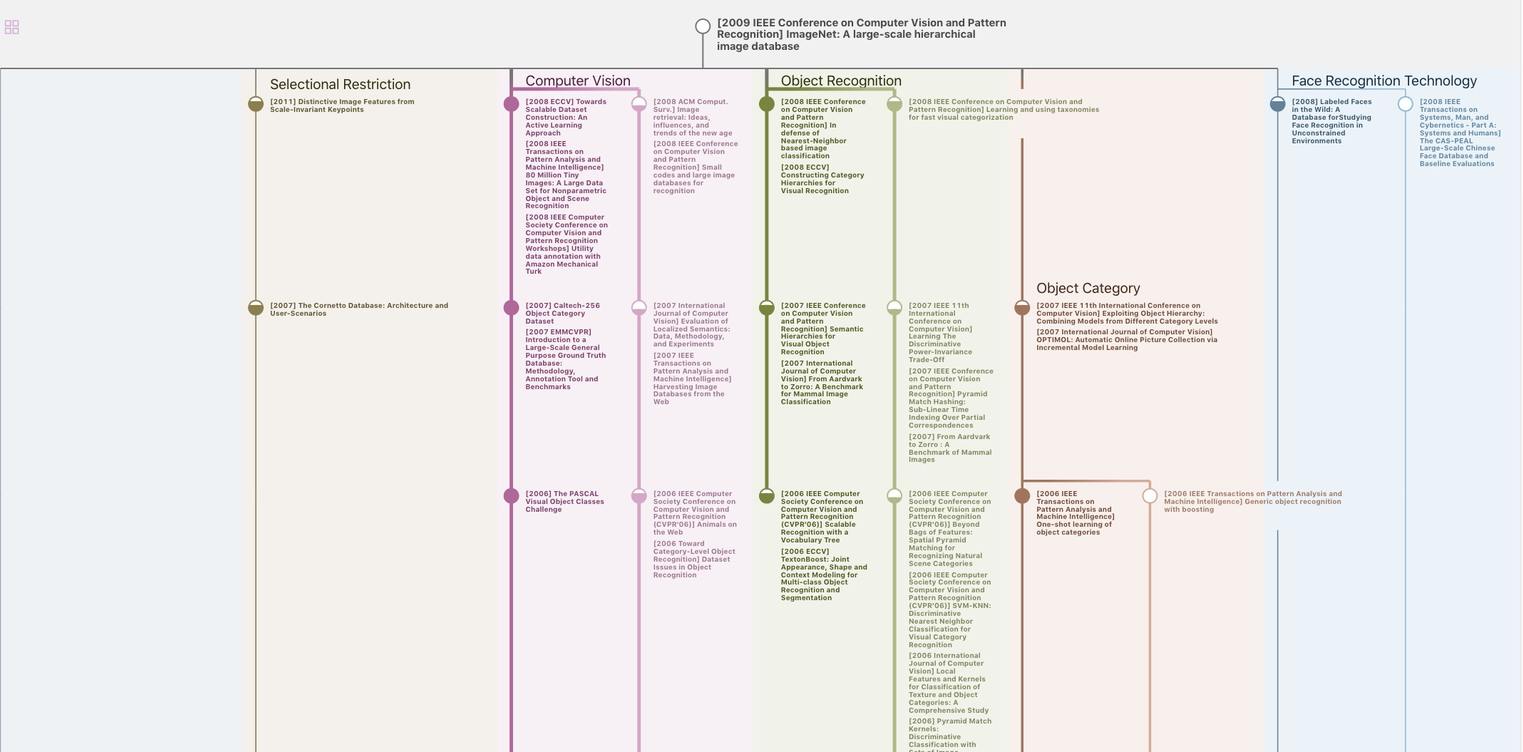
生成溯源树,研究论文发展脉络
Chat Paper
正在生成论文摘要