Replay Overshooting: Learning Stochastic Latent Dynamics with the Extended Kalman Filter
2021 IEEE INTERNATIONAL CONFERENCE ON ROBOTICS AND AUTOMATION (ICRA 2021)(2021)
摘要
This paper presents replay overshooting (RO), an algorithm that uses properties of the extended Kalman filter (EKF) to learn nonlinear stochastic latent dynamics models suitable for long-horizon prediction. We build upon overshooting methods used to train other prediction models and recover a novel variational learning objective. Further, we use RO to extend another objective that acts as a surrogate for the true log-likelihood, and show that this objective empirically yields better models than the variational one. We evaluate RO on two tasks: prediction of synthetic video frames of a swinging motorized pendulum and prediction of the planar position of various objects being pushed by a real manipulator (MIT Push Dataset). Our model outperforms several other prediction models on both quantitative and qualitative metrics.
更多查看译文
关键词
replay overshooting,extended Kalman filter,nonlinear stochastic latent dynamics models,long-horizon prediction,overshooting methods,variational learning objective,RO,swinging motorized pendulum,planar position prediction,manipulator,MIT Push Dataset,quantitative metric,qualitative metric
AI 理解论文
溯源树
样例
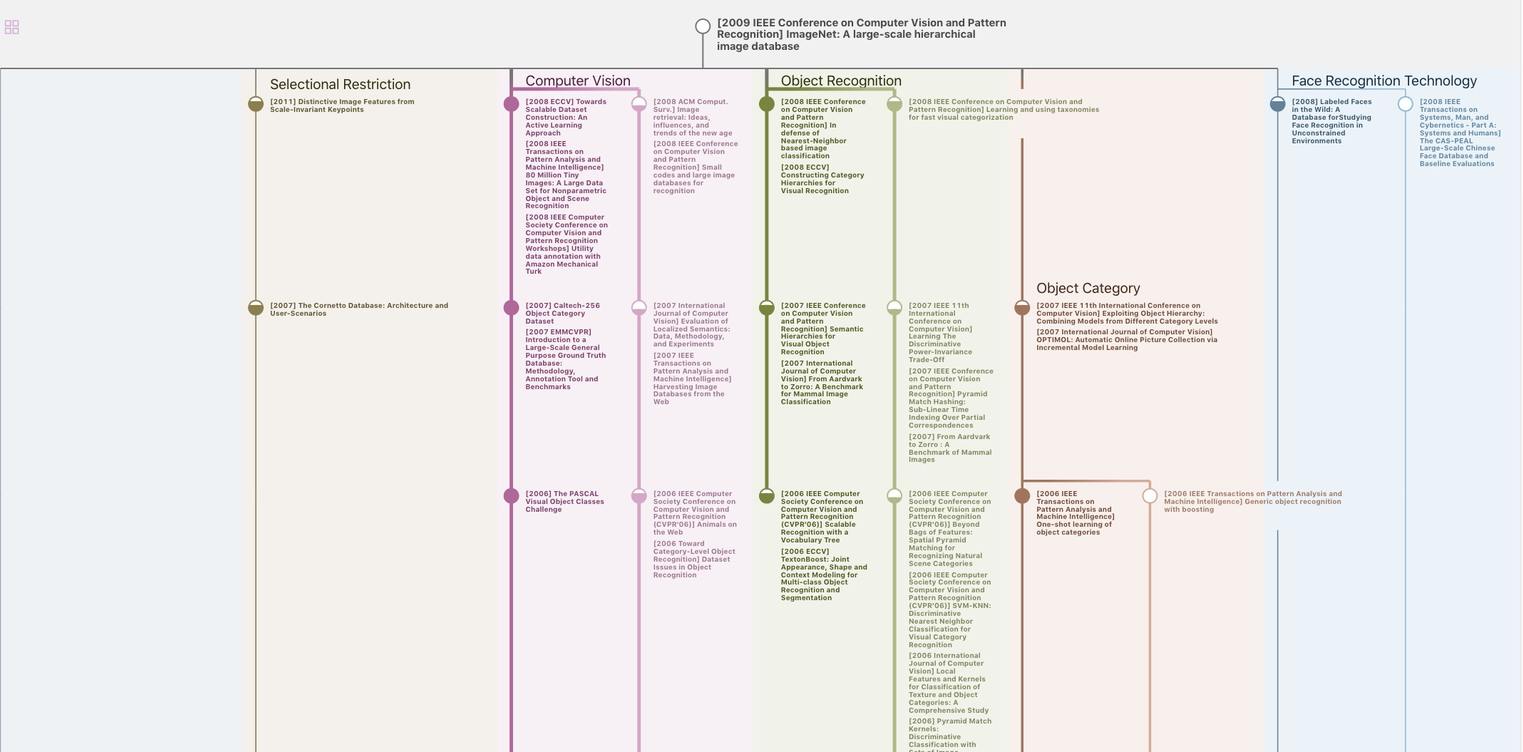
生成溯源树,研究论文发展脉络
Chat Paper
正在生成论文摘要