Temporal Knowledge Distillation for on-device Audio Classification
IEEE International Conference on Acoustics, Speech, and Signal Processing (ICASSP)(2022)
摘要
Improving the performance of on-device audio classification models remains a challenge given the computational limits of the mobile environment. Many studies leverage knowledge distillation to boost predictive performance by transferring the knowledge from large models to on-device models. However, most lack the essence of the temporal information which is crucial to audio classification tasks, or similar architecture is often required. In this paper, we propose a new knowledge distillation method designed to incorporate the temporal knowledge embedded in attention weights of large models to on-device models. Our distillation method is applicable to various types of architectures, including the non-attention-based architectures such as CNNs or RNNs, without any architectural change during inference. Through extensive experiments on both an audio event detection dataset and a noisy keyword spotting dataset, we show that our proposed method improves the predictive performance across diverse on-device architectures.
更多查看译文
关键词
On-device,Audio Classification,Knowledge Distillation,Transformer
AI 理解论文
溯源树
样例
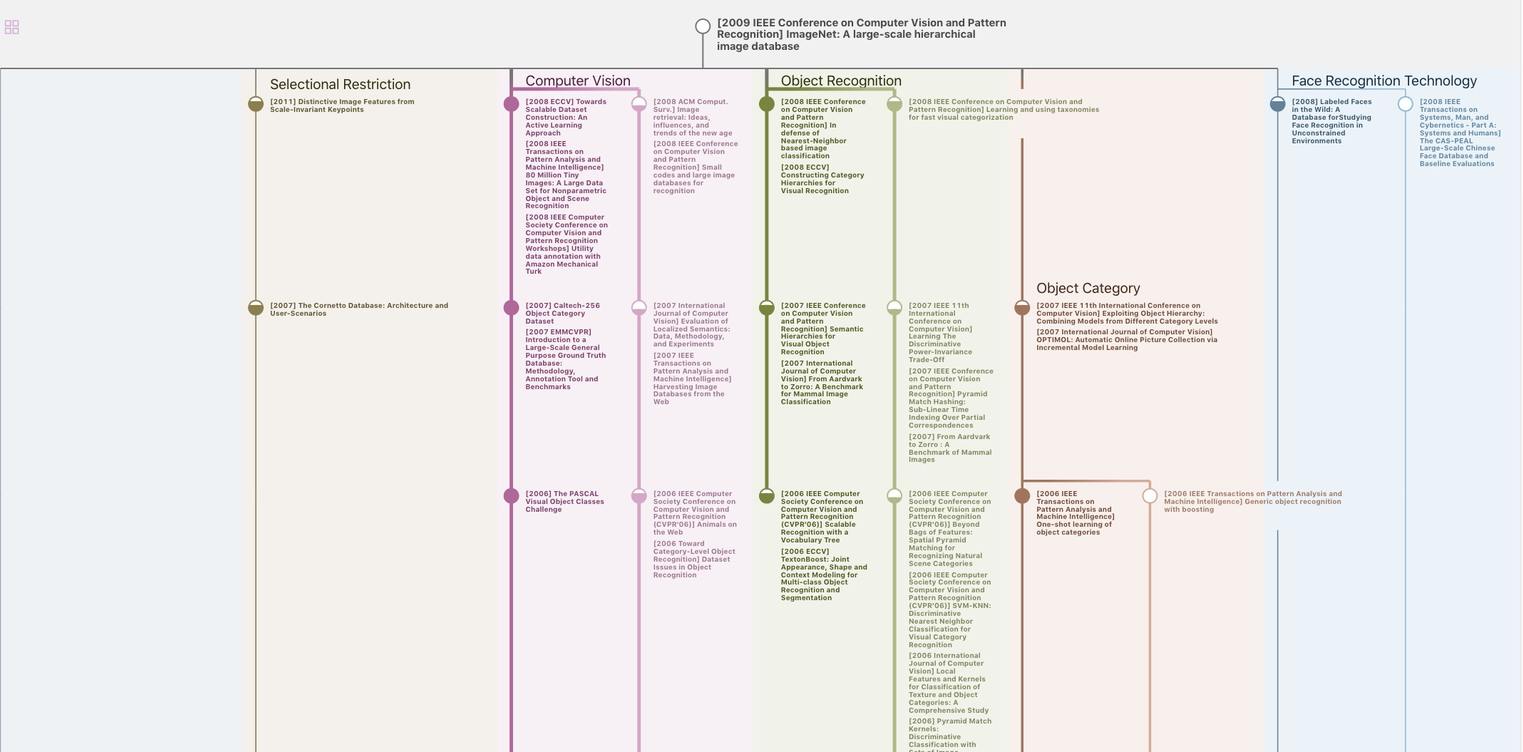
生成溯源树,研究论文发展脉络
Chat Paper
正在生成论文摘要