Model based Multi-agent Reinforcement Learning with Tensor Decompositions
CoRR(2021)
Abstract
A challenge in multi-agent reinforcement learning is to be able to generalize over intractable state-action spaces. Inspired from Tesseract [Mahajan et al., 2021], this position paper investigates generalisation in state-action space over unexplored state-action pairs by modelling the transition and reward functions as tensors of low CP-rank. Initial experiments on synthetic MDPs show that using tensor decompositions in a model-based reinforcement learning algorithm can lead to much faster convergence if the true transition and reward functions are indeed of low rank.
MoreTranslated text
Key words
tensor decompositions,reinforcement learning,multi-agent
AI Read Science
Must-Reading Tree
Example
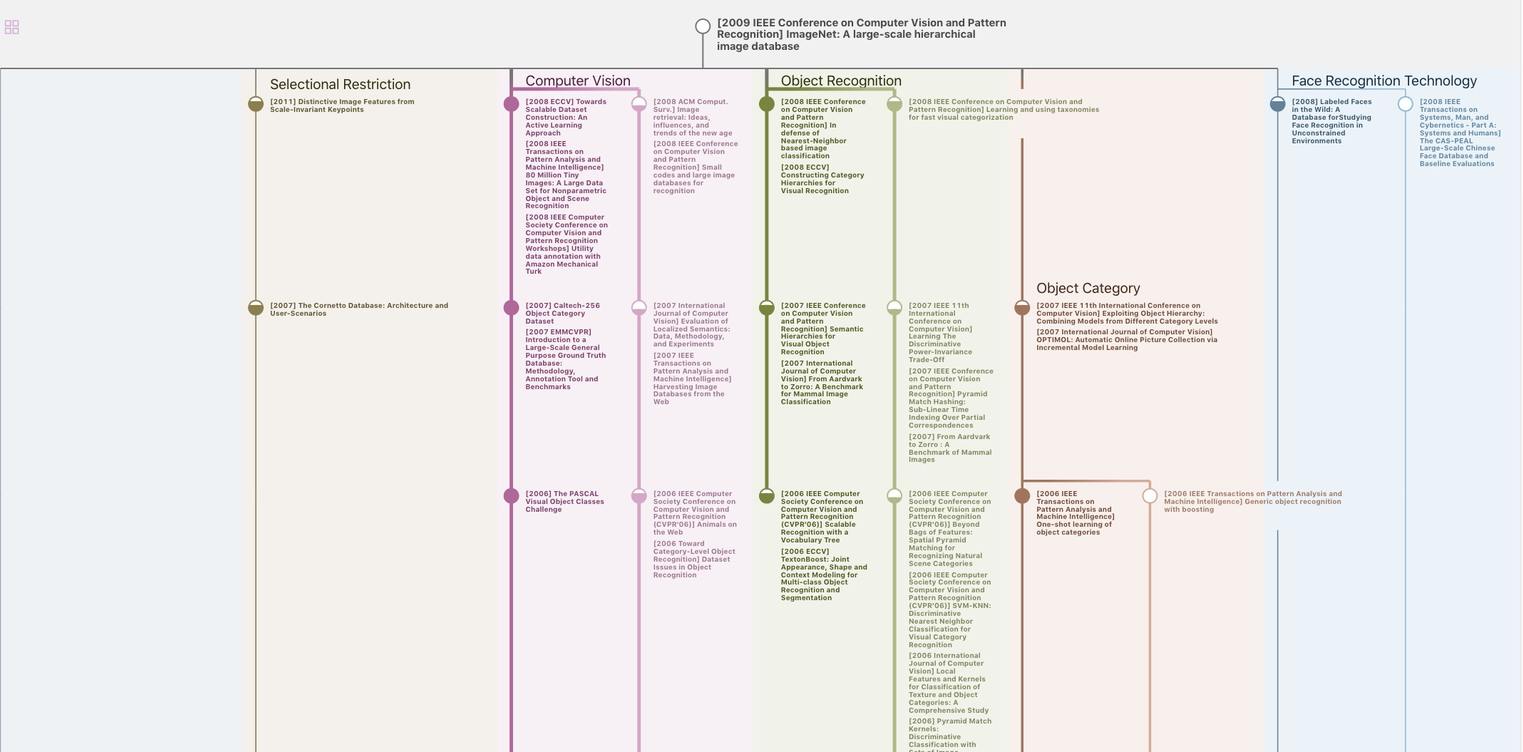
Generate MRT to find the research sequence of this paper
Chat Paper
Summary is being generated by the instructions you defined