Deep learning via message passing algorithms based on belief propagation
MACHINE LEARNING-SCIENCE AND TECHNOLOGY(2022)
摘要
Message-passing algorithms based on the belief propagation (BP) equations constitute a well-known distributed computational scheme. They yield exact marginals on tree-like graphical models and have also proven to be effective in many problems defined on loopy graphs, from inference to optimization, from signal processing to clustering. The BP-based schemes are fundamentally different from stochastic gradient descent (SGD), on which the current success of deep networks is based. In this paper, we present and adapt to mini-batch training on GPUs a family of BP-based message-passing algorithms with a reinforcement term that biases distributions towards locally entropic solutions. These algorithms are capable of training multi-layer neural networks with performance comparable to SGD heuristics in a diverse set of experiments on natural datasets including multi-class image classification and continual learning, while being capable of yielding improved performances on sparse networks. Furthermore, they allow to make approximate Bayesian predictions that have higher accuracy than point-wise ones.
更多查看译文
关键词
artificial neural networks, deep learning, approximate message passing, belief propagation, bayesian neural networks
AI 理解论文
溯源树
样例
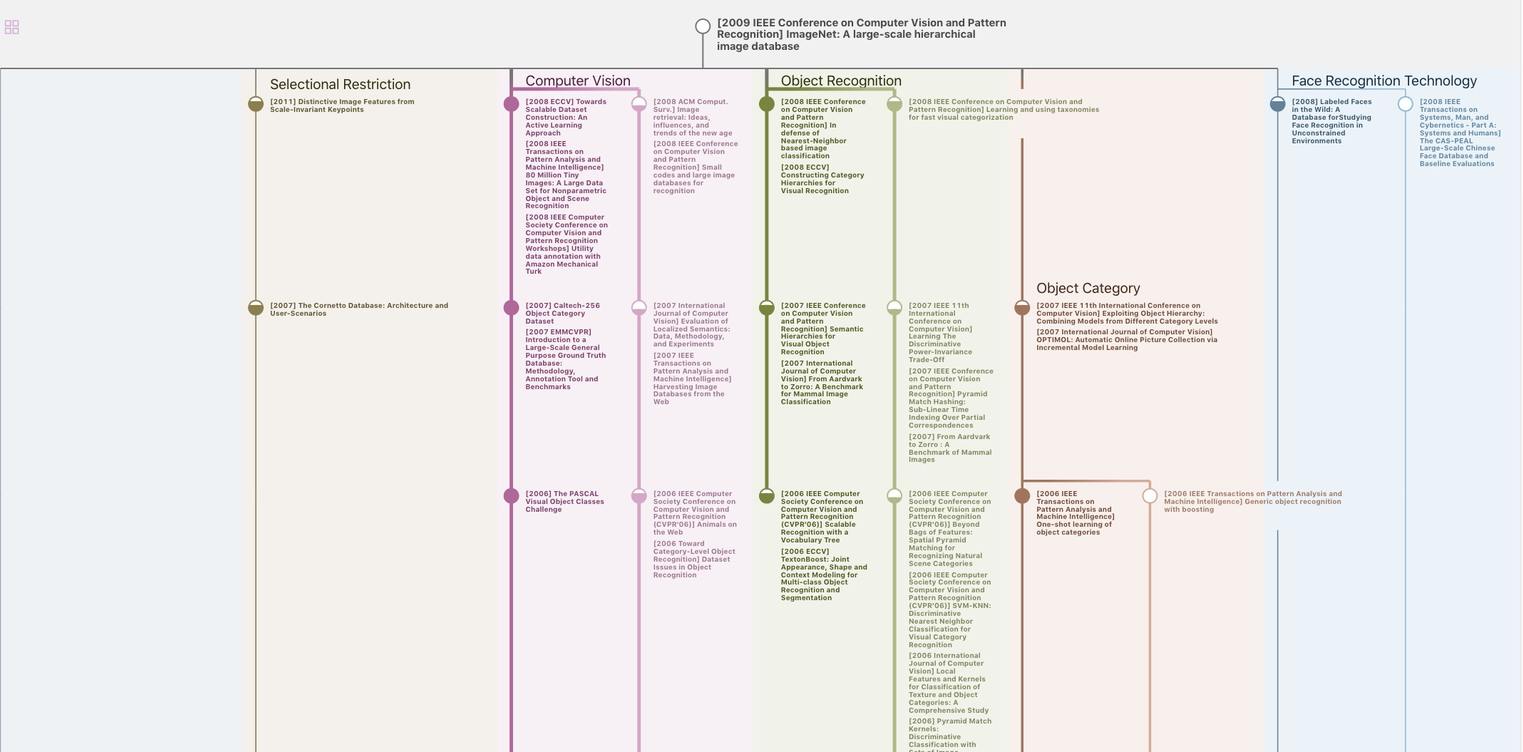
生成溯源树,研究论文发展脉络
Chat Paper
正在生成论文摘要