Scalable Bayesian Network Structure Learning with Splines.
European Workshop on Probabilistic Graphical Models (PGM)(2022)
摘要
A Bayesian Network (BN) is a probabilistic graphical model consisting of a directed acyclic graph (DAG), where each node is a random variable represented as a function of its parents. We present a novel approach capable of learning the global DAG structure of a BN and modelling linear and non-linear local relationships between variables. We achieve this by a combination of feature selection to reduce the search space for local relationships, and extending the widely used score-and-search approach to support modelling relationships between variables as Multivariate Adaptive Regression Splines (MARS). MARS are polynomial regression models represented as piecewise spline functions - this lets us model non-linear relationships without the risk of overfitting that a single polynomial regression model would bring. The combination allows us to learn relationships in all bnlearn benchmark instances within minutes and enables us to scale to networks of over a thousand nodes
更多查看译文
关键词
structure,network,learning
AI 理解论文
溯源树
样例
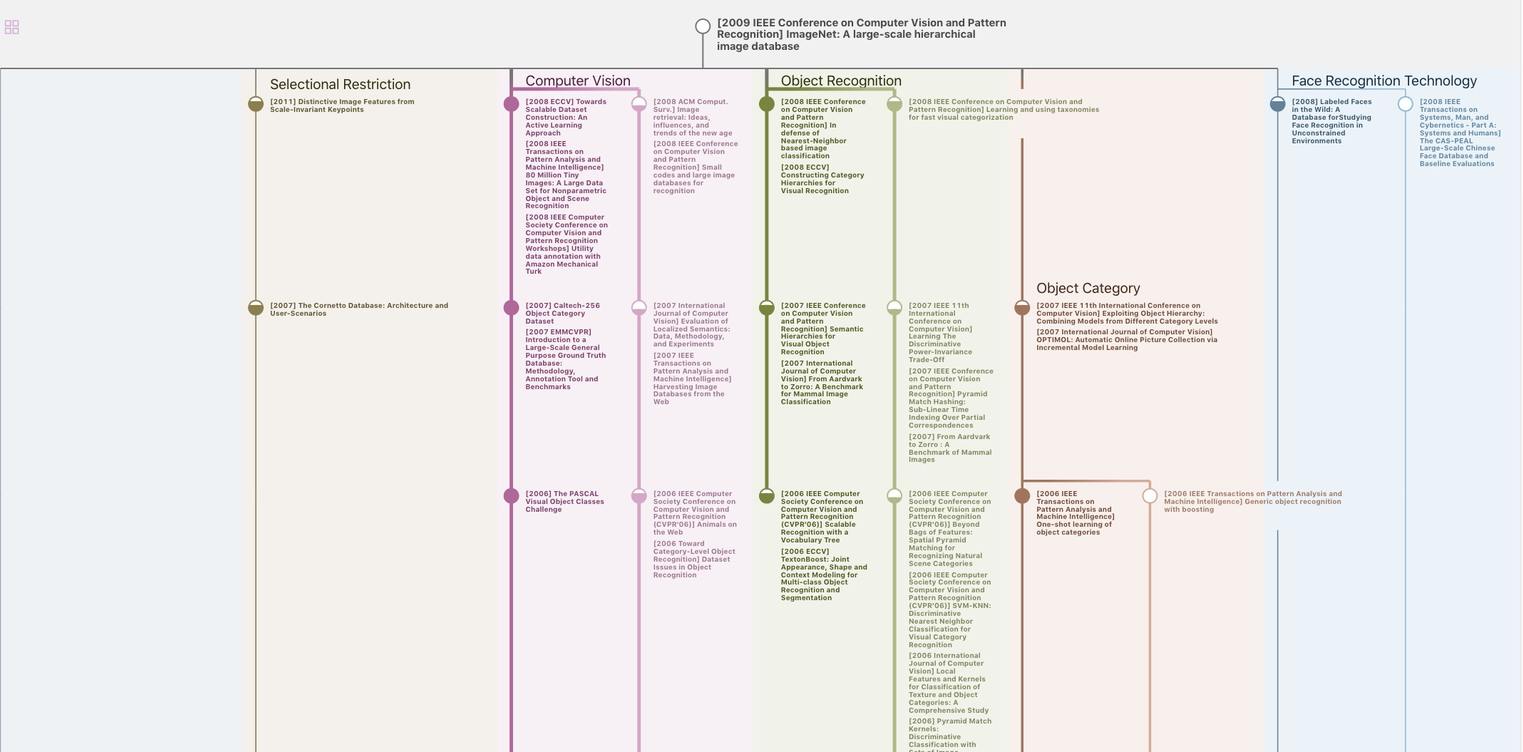
生成溯源树,研究论文发展脉络
Chat Paper
正在生成论文摘要