Flexible Projection Search Using Optimal Re-weighted Adjacency for Unsupervised Manifold Learning.
PRCV(2021)
摘要
Graph-based manifold learning plays an important role in clustering and classification tasks. Regarding the unsupervised case, the local structure of each sample is vital to the quality of clustering results. Many state-of-the-art methods seek the low-dimensional projection matrix for graph embedding, ignoring the contortion of original local structure in the projected space, which impairs the quality of clustering. To remedy this shortcoming, we propose an iterative leaning approach in this paper, aiming at preserving the original locality in each iteration. During iterative steps, adjacency weights of each currently projected sample are optimally defined by quadratic programming with equality constraints, and in return the upcoming projection matrix that attempts to keep the original locality is flexibly determined based on the current weights. Such iteration proceeds until the objective function value converges. In particular, the proposed approach requires very few parameters, leading to simple operation in experiments. Further, local distances of projected samples could be directly obtained from their inner products, with no explicit calculation of projection matrix in each iteration. We repeat k-means clustering many times w.r.t these samples after convergence, and experimental results reveal the obvious better clustering quality of the proposed approach on average, compared to the state-of the-art ones.
更多查看译文
关键词
Unsupervised manifold leaning,Quadratic programming,Flexible projection,Re-weighted adjacency,k-means clustering
AI 理解论文
溯源树
样例
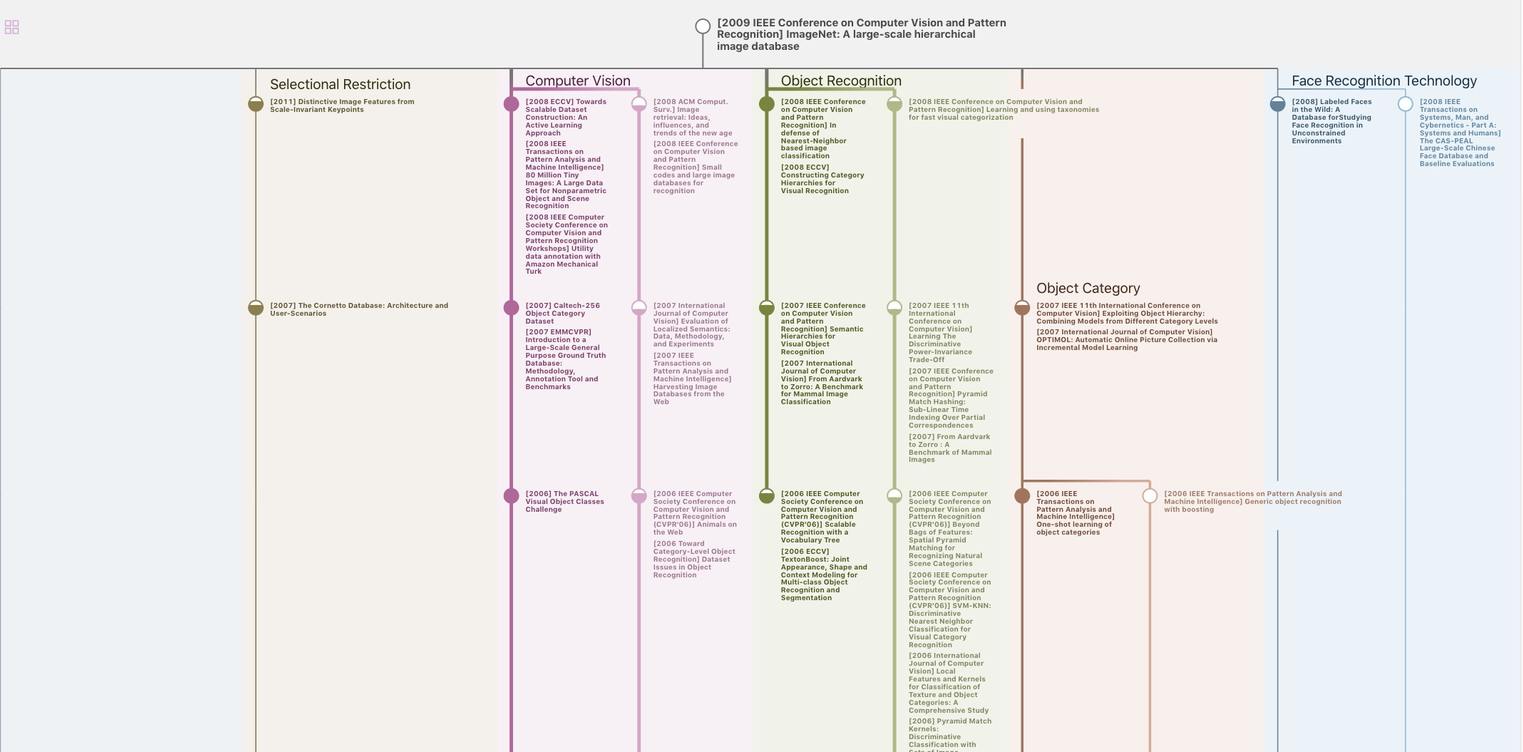
生成溯源树,研究论文发展脉络
Chat Paper
正在生成论文摘要