Segmentation of Intracellular Structures in Fluorescence Microscopy Images by Fusing Low-Level Features.
PRCV(2021)
摘要
Intracellular organelles such as the endoplasmic reticulum, mitochondria, and the cell nucleus exhibit complex structures and diverse morphologies. These intracellular structures (ICSs) play important roles in serving essential physiological functions of cells. Their accurate segmentation is crucial to many important life sciences and clinical applications. When using deep learning-based segmentation models to extract ICSs in fluorescence microscopy images (FLMIs), we find that U-Net provides superior performance, while other well-designed models such as DeepLabv3+ and SegNet perform poorly. By investigating the relative importance of the features learned by U-Net, we find that low-level features play a dominant role. Therefore, we develop a simple strategy that modifies general-purpose segmentation models by fusing low-level features via a decoder architecture to improve their performance in segmenting ICSs from FLMIs. For a given segmentation model, we first use a group of convolutions at the original image scale as the input layer to obtain low-level features. We then use a decoder to fuse the multi-scale features, which directly passes information of low-level features to the prediction layer. Experimental results on two custom datasets, ER and MITO, and a public dataset NUCLEUS, show that the strategy substantially improves the performance of all the general-purpose models tested in segmentation of ICSs from FLMIs. Data and code of this study are available at https://git hub.com/cbmi-group/icss-segmentation.
更多查看译文
关键词
fluorescence microscopy images,intracellular structures,segmentation,low-level
AI 理解论文
溯源树
样例
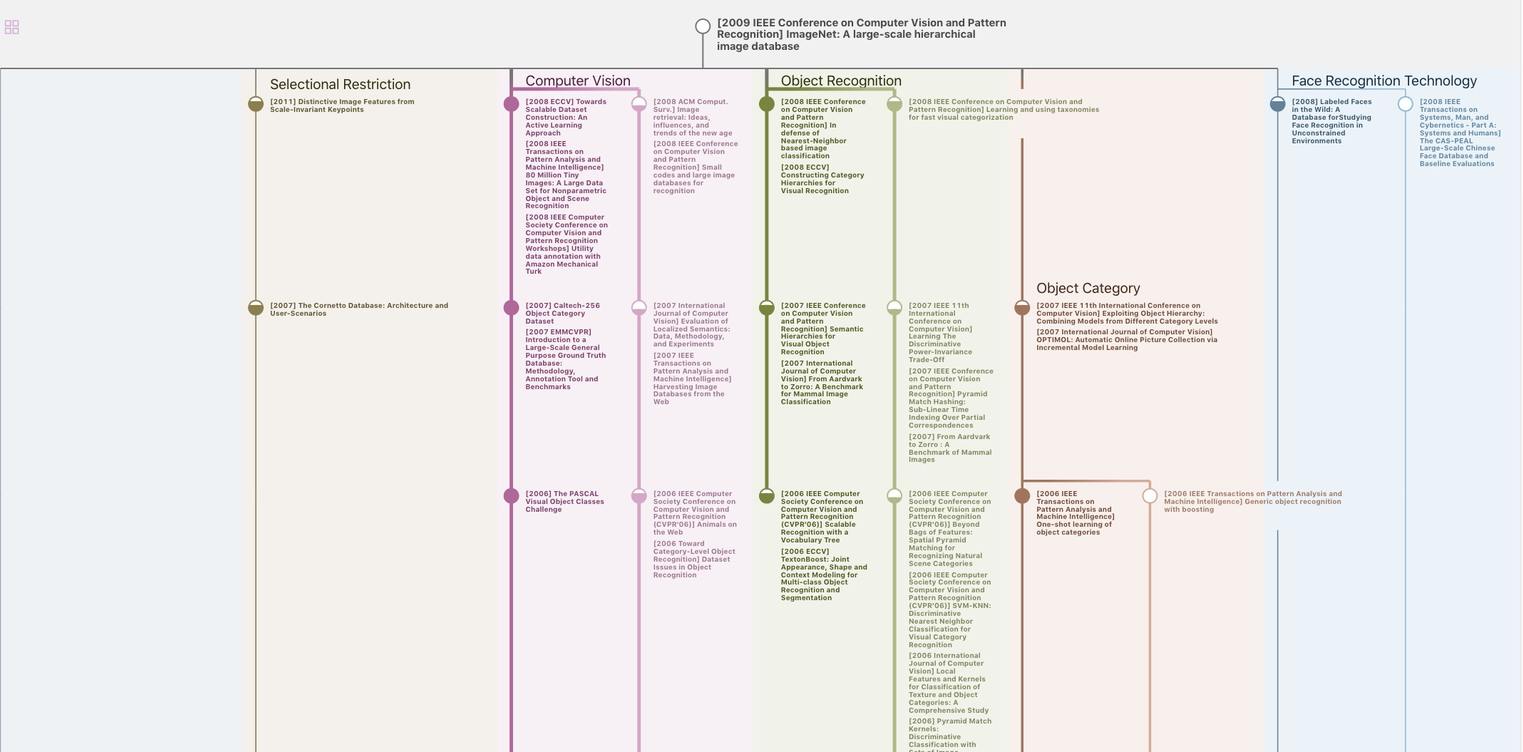
生成溯源树,研究论文发展脉络
Chat Paper
正在生成论文摘要