Antagonistic Procedural Content Generation Of Sparse Reward Game.
FDG(2021)
摘要
With the development of the game industry, procedural content generation technology has been widely used in game automatic content generation. And with the help of this technology, designers can quickly generate unlimited levels, implement real-time level generation, preset difficulty level generation, and so on. However, due to the difficulty of providing real-time and continuous feedback from game elements, sparse reward game is difficult to meet the expected results in content generation. This paper uses search-based procedural content generation combined with auxiliary task reinforcement learning to implement automatic content generation of sparse bonus games by means of confrontation generation. In this method, hierarchical reinforcement learning can smoothly evaluate the fitness of the generated candidate individuals, and screen individual populations according to the obtained fitness. This generation method is based on agent simulation, and generates runnable levels through free exploration of agents, which is more novel than traditional rule-based generation. In the end, we successfully implemented the procedural content generation of a typical sparse reward game, proving that our method is feasible. Different sparse reward games have different task complexity. By modifying the levels and tasks of hierarchical reinforcement learning, we can extend this method to other sparse reward games.
更多查看译文
AI 理解论文
溯源树
样例
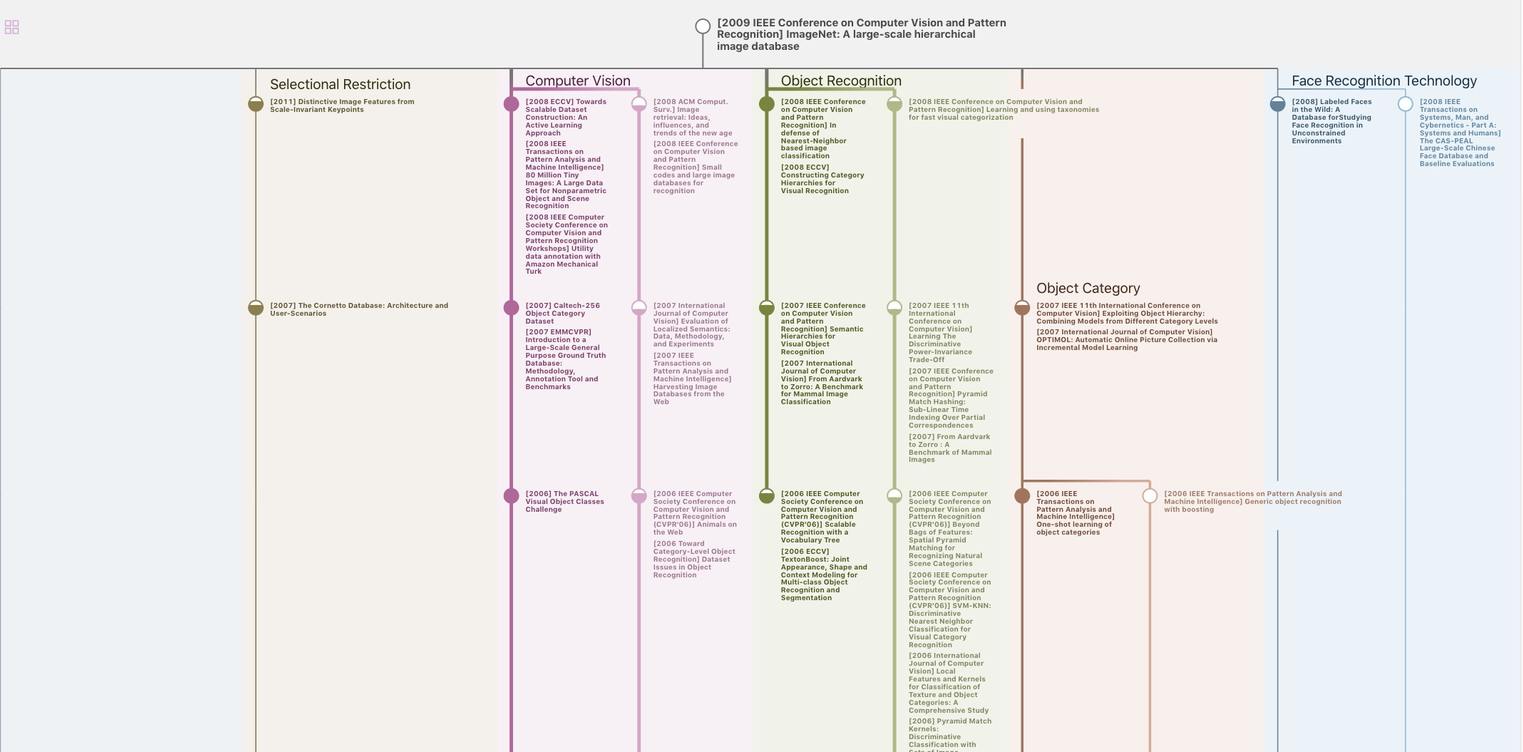
生成溯源树,研究论文发展脉络
Chat Paper
正在生成论文摘要