How Well Does Kohn-Sham Regularizer Work for Weakly Correlated Systems?
JOURNAL OF PHYSICAL CHEMISTRY LETTERS(2022)
摘要
Kohn-Sham regularizer (KSR) is a differentiable machine learning approach to finding the exchange-correlation functional in Kohn-Sham density functional theory that works for strongly correlated systems. Here we test KSR for a weak correlation. We propose spin-adapted KSR (sKSR) with trainable local, semilocal, and nonlocal approximations found by minimizing density and total energy loss. We assess the atoms-to-molecules generalizability by training on one-dimensional (1D) H, He, Li, Be, and Be2+ and testing on 1D hydrogen chains, LiH, BeH2, and helium hydride complexes. The generalization error from our semilocal approximation is comparable to other differentiable approaches, but our nonlocal functional outperforms any existing machine learning functionals, predicting ground-state energies of test systems with a mean absolute error of 2.7 mH.
更多查看译文
AI 理解论文
溯源树
样例
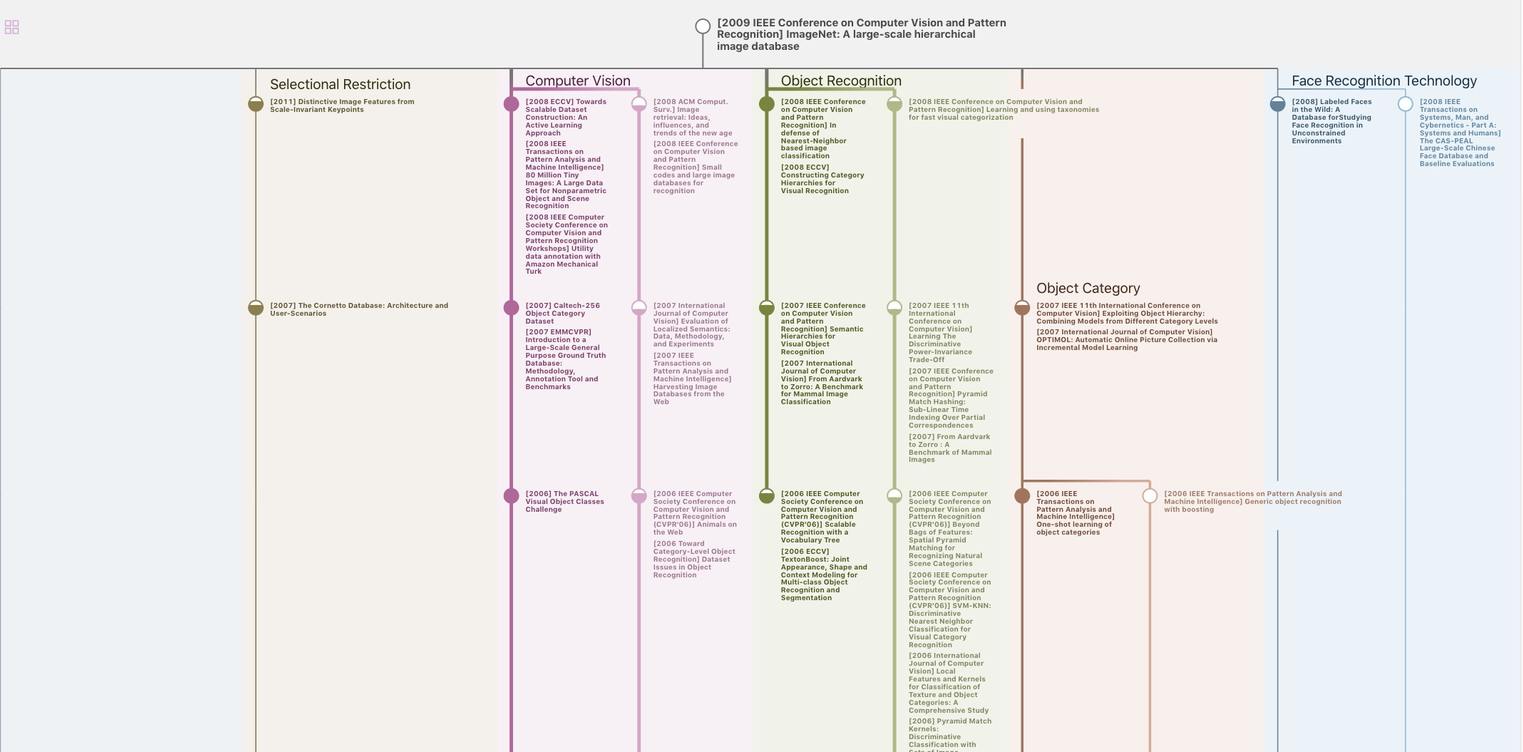
生成溯源树,研究论文发展脉络
Chat Paper
正在生成论文摘要