A spiking network model for semantic representation and replay-based association acquisition.
ICONS(2021)
摘要
The ability to form and store several types of associations between representations of natural images is an area of ongoing research in artificial deep neural networks, which may be informed by biologically-inspired computational models. It is hypothesized that replay of sensory stimuli through cortical-hippocampal connections is responsible for training associations between events, as a powerful form of associative learning. While models of associative memories and sensory processing have been studied extensively, there is a potential for spiking models encompassing sensory processing, reasoning over associations, and learning representations which has not been previously demonstrated. Such networks would be suitable for reasoning and learning from visual data on neuromorphic hardware. In this work, we demonstrate a novel visual reasoning network capable of representing semantic relationships and learning new associations through replay-based association with spiking models using natural images. This is demonstrated through associations of natural images from Tiny Imagenet with a knowledge graph derived from WordNet, and we show that relations in the knowledge graph can be accurately traversed for multiple sequential queries. We also demonstrate learning of a novel association after replayed presentations of natural images. This represents a novel capability for machine learning and reasoning with spiking neural networks which may be amenable to neuromorphic hardware.
更多查看译文
AI 理解论文
溯源树
样例
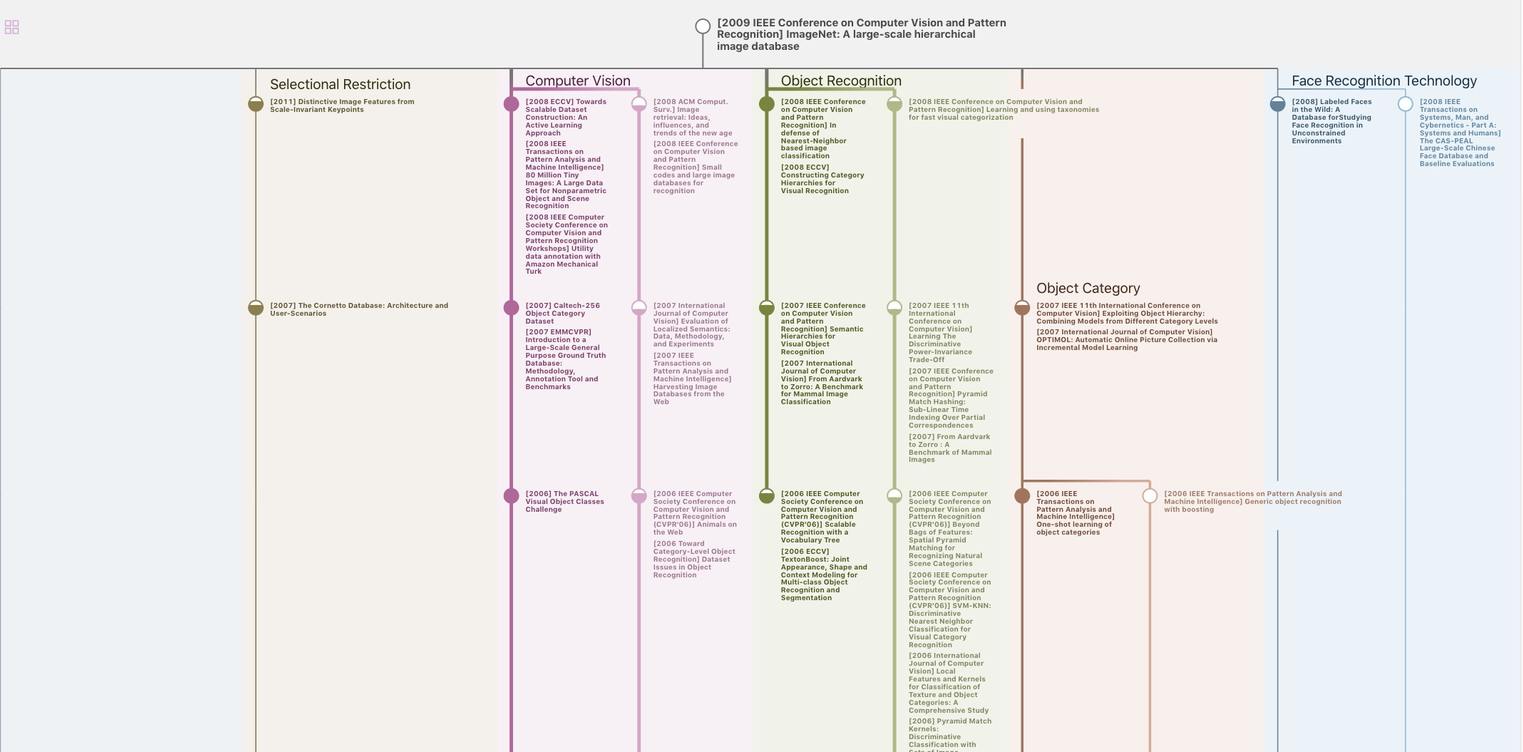
生成溯源树,研究论文发展脉络
Chat Paper
正在生成论文摘要