Optimal prediction for kernel-based semi-functional linear regression
ANALYSIS AND APPLICATIONS(2023)
摘要
This paper proposes a novel prediction approach for a semi-functional linear model comprising a functional and a nonparametric component. The study establishes the minimax optimal rates of convergence for this model, revealing that the functional component can be learned with the same minimax rate as if the nonparametric component were known and vice versa. This result can be achieved by using a double-penalized least squares method to estimate both the functional and nonparametric components within the framework of reproducing kernel Hilbert spaces. Thanks to the representer theorem, the approach also offers other desirable features, including the algorithm efficiency requiring no iterations. We also provide numerical studies to demonstrate the effectiveness of the method and validate the theoretical analysis.
更多查看译文
关键词
Learning theory,convergence rate,semi-functional linear regression,minimax optimality,reproducing kernel Hilbert spaces
AI 理解论文
溯源树
样例
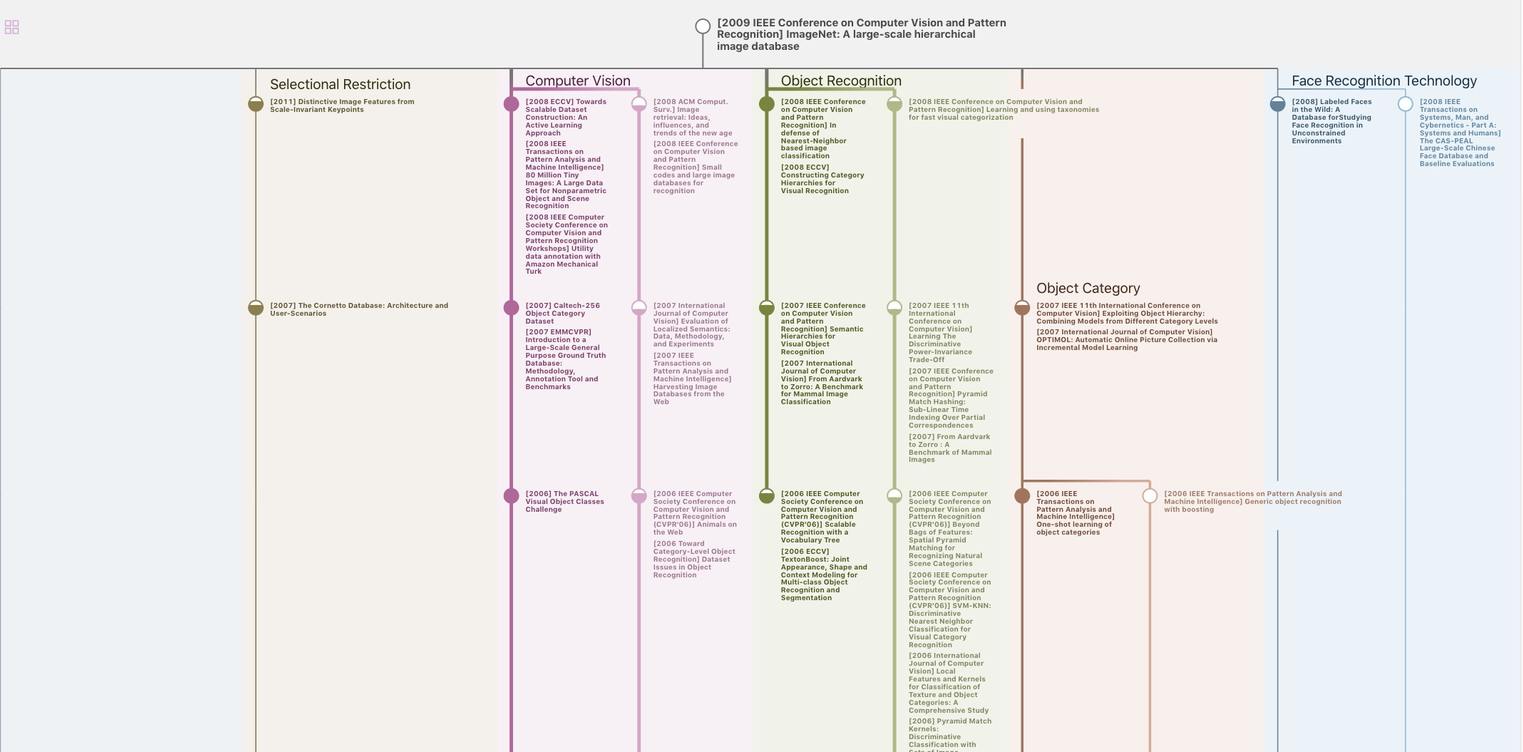
生成溯源树,研究论文发展脉络
Chat Paper
正在生成论文摘要