{\epsilon}-weakened Robustness of Deep Neural Networks
arXiv (Cornell University)(2021)
摘要
This paper introduces a notation of $\varepsilon$-weakened robustness for analyzing the reliability and stability of deep neural networks (DNNs). Unlike the conventional robustness, which focuses on the "perfect" safe region in the absence of adversarial examples, $\varepsilon$-weakened robustness focuses on the region where the proportion of adversarial examples is bounded by user-specified $\varepsilon$. Smaller $\varepsilon$ means a smaller chance of failure. Under such robustness definition, we can give conclusive results for the regions where conventional robustness ignores. We prove that the $\varepsilon$-weakened robustness decision problem is PP-complete and give a statistical decision algorithm with user-controllable error bound. Furthermore, we derive an algorithm to find the maximum $\varepsilon$-weakened robustness radius. The time complexity of our algorithms is polynomial in the dimension and size of the network. So, they are scalable to large real-world networks. Besides, We also show its potential application in analyzing quality issues.
更多查看译文
关键词
robustness,neural networks
AI 理解论文
溯源树
样例
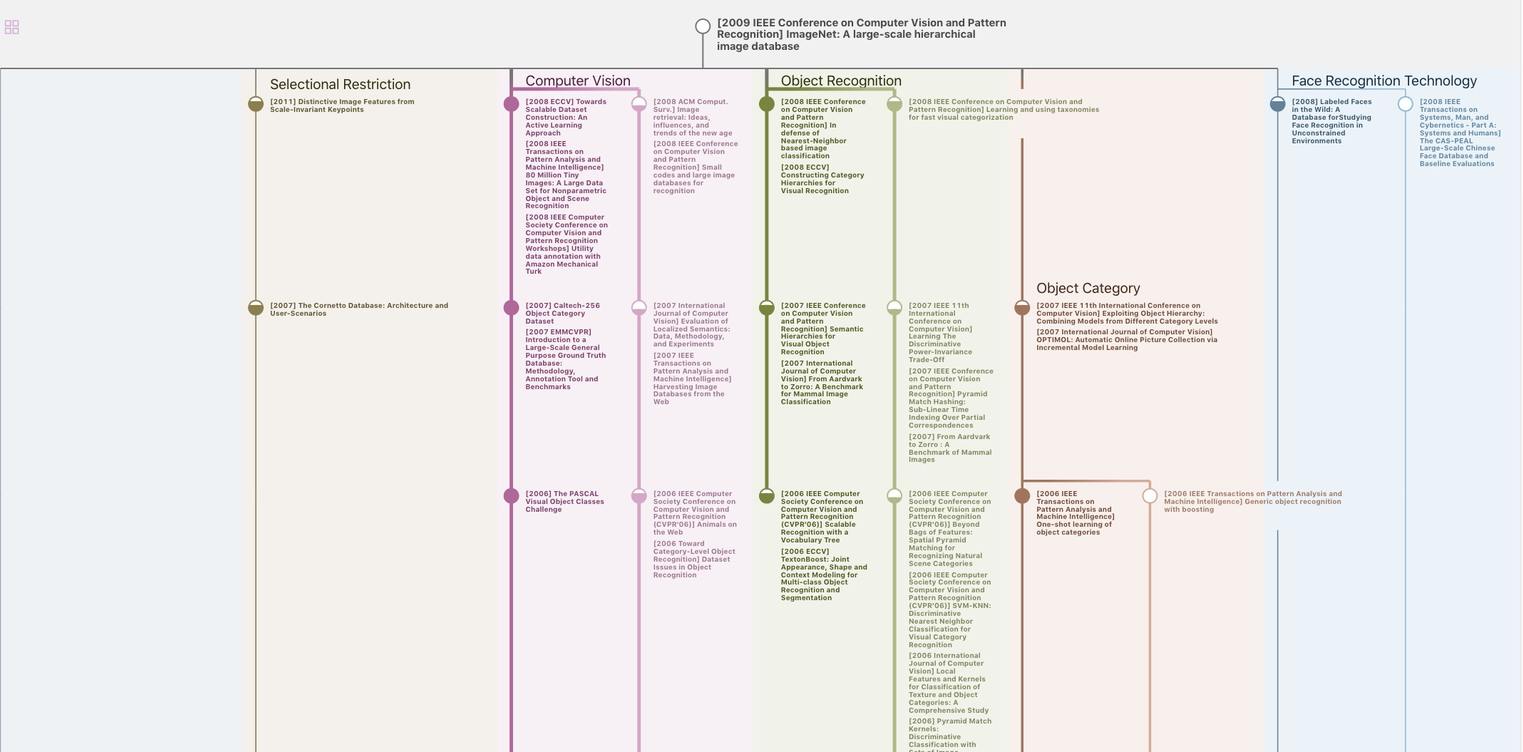
生成溯源树,研究论文发展脉络
Chat Paper
正在生成论文摘要