Holistic deep learning
arxiv(2024)
摘要
This paper presents a novel holistic deep learning framework that simultaneously addresses the challenges of vulnerability to input perturbations, overparametrization, and performance instability from different train-validation splits. The proposed framework holistically improves accuracy, robustness, sparsity, and stability over standard deep learning models, as demonstrated by extensive experiments on both tabular and image data sets. The results are further validated by ablation experiments and SHAP value analysis, which reveal the interactions and trade-offs between the different evaluation metrics. To support practitioners applying our framework, we provide a prescriptive approach that offers recommendations for selecting an appropriate training loss function based on their specific objectives. All the code to reproduce the results can be found at https://github.com/kimvc7/HDL .
更多查看译文
关键词
deep learning
AI 理解论文
溯源树
样例
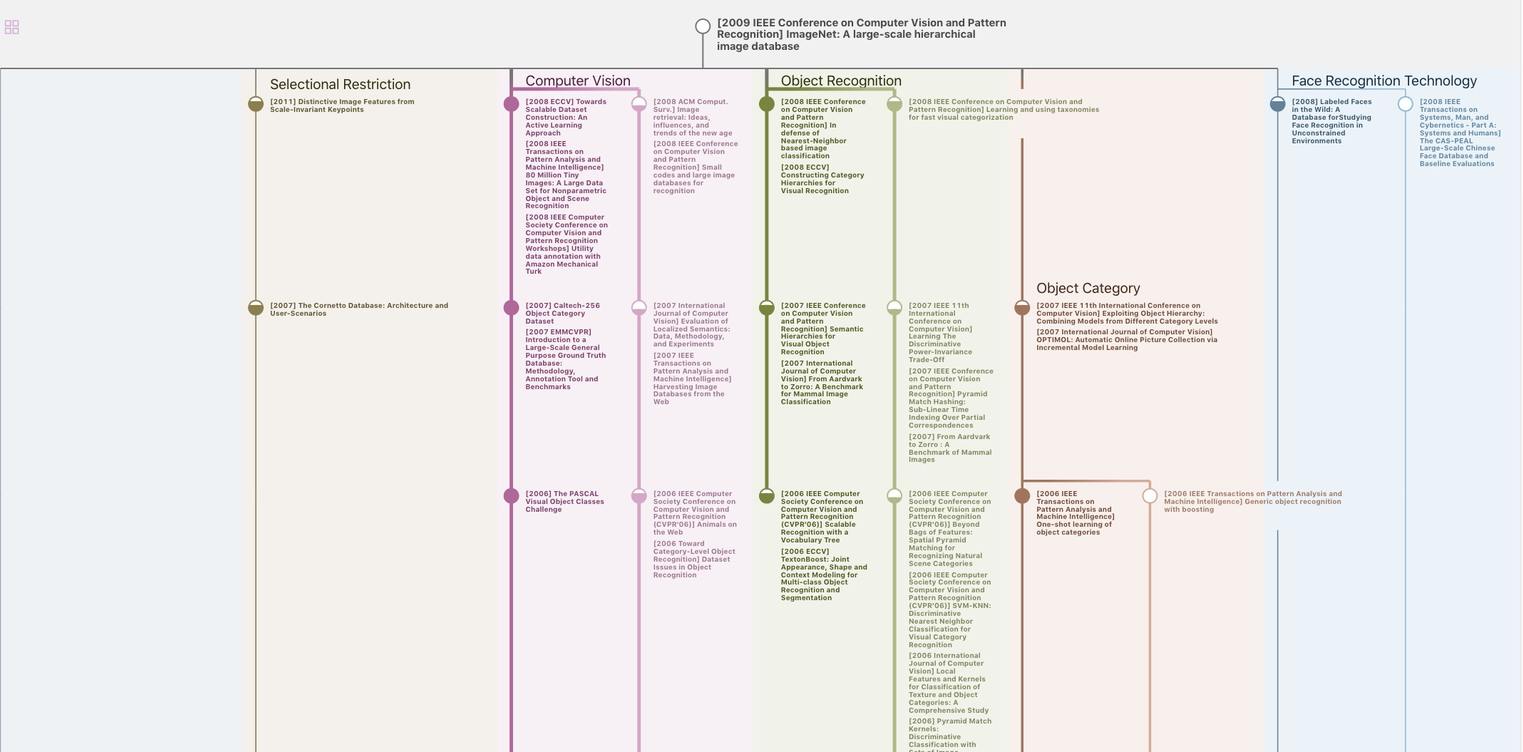
生成溯源树,研究论文发展脉络
Chat Paper
正在生成论文摘要