Visual Explanations for Convolutional Neural Networks via Latent Traversal
arxiv(2021)
摘要
Lack of explainability in artificial intelligence, specifically deep neural networks, remains a bottleneck for implementing models in practice. Popular techniques such as Gradient-weighted Class Activation Mapping (Grad-CAM) provide a coarse map of salient features in an image, which rarely tells the whole story of what a convolutional neural network (CNN) learned. Using COVID-19 chest X-rays, we present a method for interpreting what a CNN has learned by utilizing Generative Adversarial Networks (GANs). Our GAN framework disentangles lung structure from COVID-19 features. Using this GAN, we can visualize the transition of a pair of COVID negative lungs in a chest radiograph to a COVID positive pair by interpolating in the latent space of the GAN, which provides fine-grained visualization of how the CNN responds to varying features within the lungs.
更多查看译文
关键词
visual explanations,convolutional neural networks,latent traversal
AI 理解论文
溯源树
样例
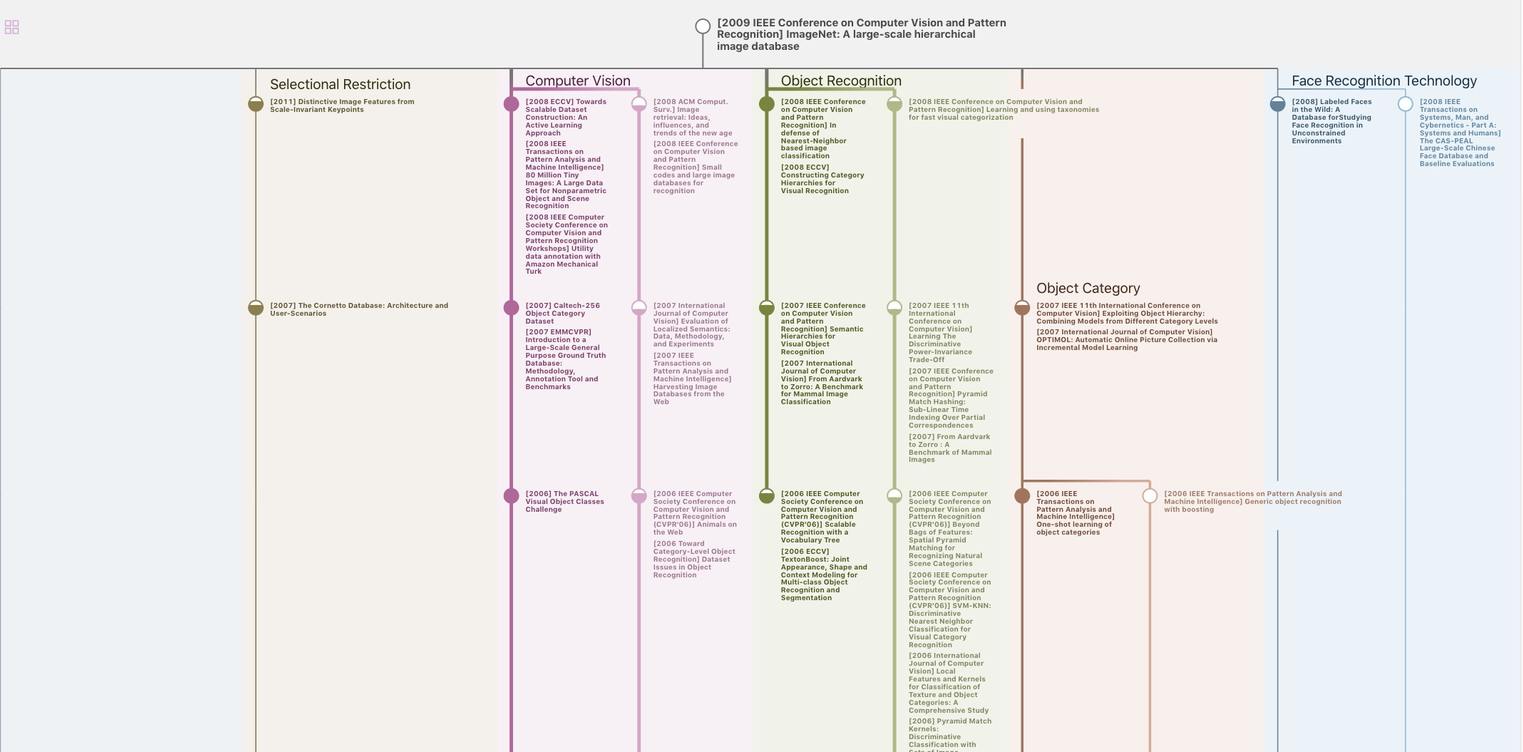
生成溯源树,研究论文发展脉络
Chat Paper
正在生成论文摘要