On quadrature rules for solving Partial Differential Equations using Neural Networks
COMPUTER METHODS IN APPLIED MECHANICS AND ENGINEERING(2022)
摘要
Neural Networks have been widely used to solve Partial Differential Equations. These methods require to approximate definite integrals using quadrature rules. Here, we illustrate via 1D numerical examples the quadrature problems that may arise in these applications and propose several alternatives to overcome them, namely: Monte Carlo methods, adaptive integration, polynomial approximations of the Neural Network output, and the inclusion of regularization terms in the loss. We also discuss the advantages and limitations of each proposed numerical integration scheme. We advocate the use of Monte Carlo methods for high dimensions (above 3 or 4), and adaptive integration or polynomial approximations for low dimensions (3 or below). The use of regularization terms is a mathematically elegant alternative that is valid for any spatial dimension; however, it requires certain regularity assumptions on the solution and complex mathematical analysis when dealing with sophisticated Neural Networks. (C) 2022 The Author(s). Published by Elsevier B.V.
更多查看译文
关键词
Deep learning, Neural Networks, Ritz method, Least-Squares method, Quadrature rules
AI 理解论文
溯源树
样例
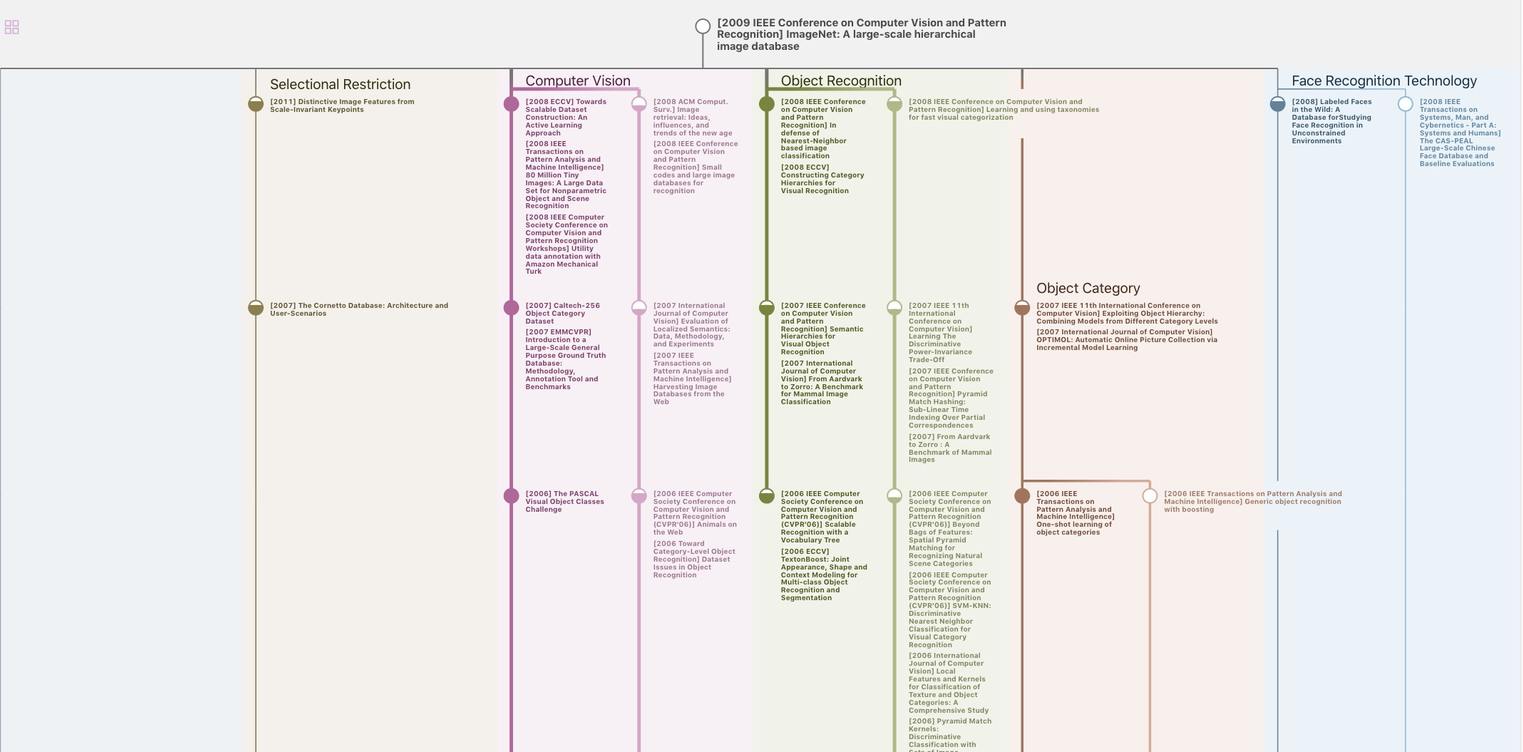
生成溯源树,研究论文发展脉络
Chat Paper
正在生成论文摘要