Wireless Federated Learning over MIMO Networks: Joint Device Scheduling and Beamforming Design.
2022 IEEE International Conference on Communications Workshops (ICC Workshops)(2022)
摘要
Federated learning (FL) is recognized as a key enabling technology to support distributed artificial intelligence (AI) services in future 6G. By supporting decentralized data training and collaborative model training among devices, FL inherently tames privacy leakage and reduces transmission costs. Whereas, the performance of the wireless FL is typically restricted by the communication latency. Multiple-input multiple-output (MIMO) technique is one promising solution to build up a communication-efficient edge FL system with limited radio resources. In this paper, we propose a novel joint device scheduling and receive beamforming design approach to reduce the FL convergence gap over shared wireless MIMO networks. Specifically, we theoretically establish the convergence analysis of the FL process, and then apply the proposed device scheduling policy to maximize the number of weighted devices under the FL system latency and sum power constraints. Numerical results verify the theoretical analysis of the FL convergence and exhibit the appealing learning performance of the proposed approach.
更多查看译文
关键词
FL convergence gap,shared wireless MIMO networks,convergence analysis,FL process,device scheduling policy,weighted devices,appealing learning performance,wireless federated learning,distributed artificial intelligence services,decentralized data training,collaborative model training,privacy leakage,transmission costs,wireless FL,multiple-input multiple-output technique,communication-efficient edge FL system,radio resources,joint device scheduling,beamforming design approach,distributed artificial intelligence,future 6G,limited radio resources
AI 理解论文
溯源树
样例
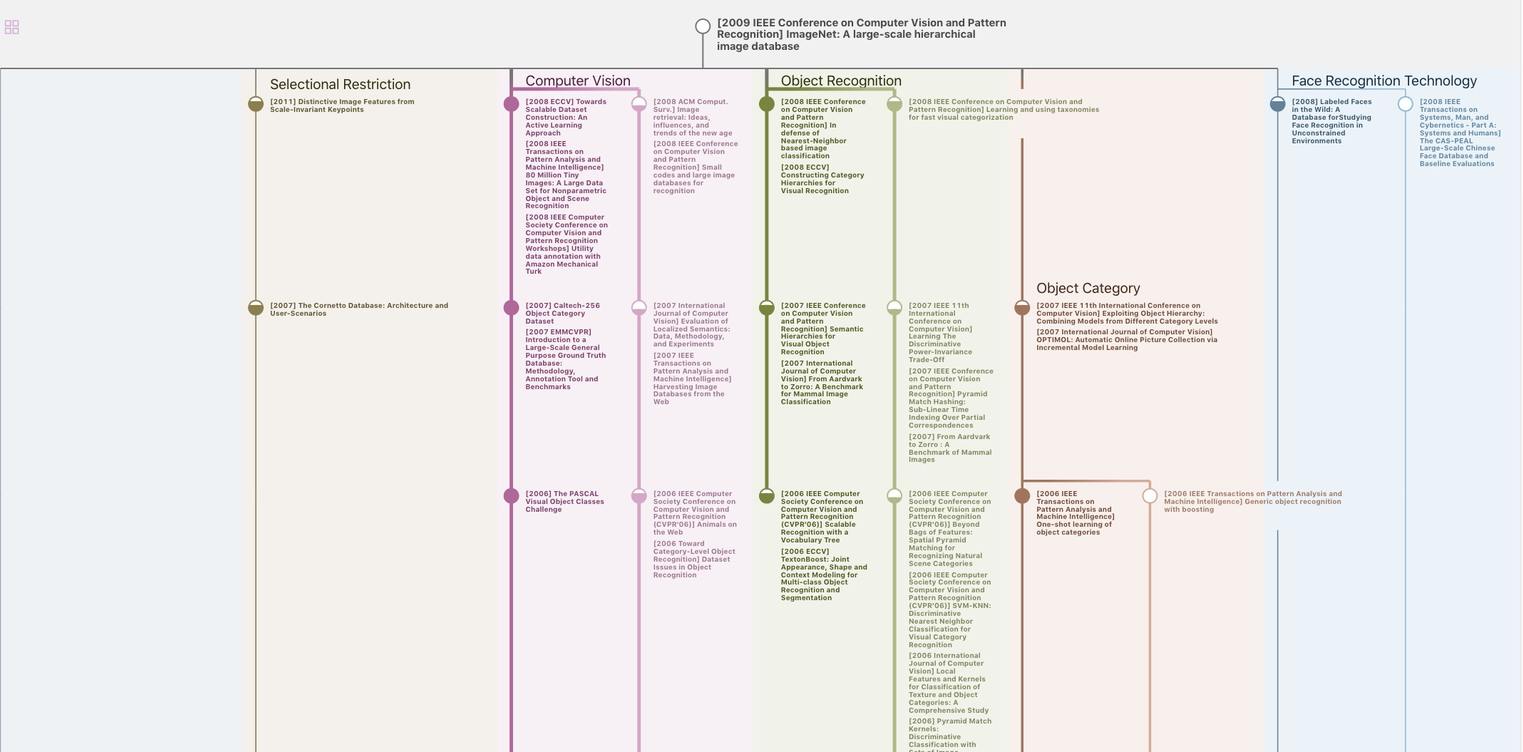
生成溯源树,研究论文发展脉络
Chat Paper
正在生成论文摘要