To Talk or to Work: Delay Efficient Federated Learning over Mobile Edge Devices
2021 IEEE GLOBAL COMMUNICATIONS CONFERENCE (GLOBECOM)(2021)
摘要
Federated learning (FL), an emerging distributed machine learning paradigm, in conflux with edge computing is a promising area with novel applications over mobile edge devices. In FL, since mobile devices collaborate to train a model based on their own data under the coordination of a central server by sharing just the model updates, training data is maintained private. However, without the central availability of data, computing nodes need to communicate the model updates often to attain convergence. Hence, the local computation time to create local model updates along with the time taken for transmitting them to and from the server result in a delay in the overall time. Furthermore, unreliable network connections may obstruct an efficient communication of these updates. To address these, in this paper, we propose a delay-efficient FL mechanism that reduces the overall time (consisting of both the computation and communication latencies) and communication rounds required for the model to converge. Exploring the impact of various parameters contributing to delay, we seek to balance the trade-off between wireless communication (to talk) and local computation (to work). We formulate a relation with overall time as an optimization problem and demonstrate the efficacy of our approach through extensive simulations.
更多查看译文
关键词
delay efficient federated learning,mobile edge
AI 理解论文
溯源树
样例
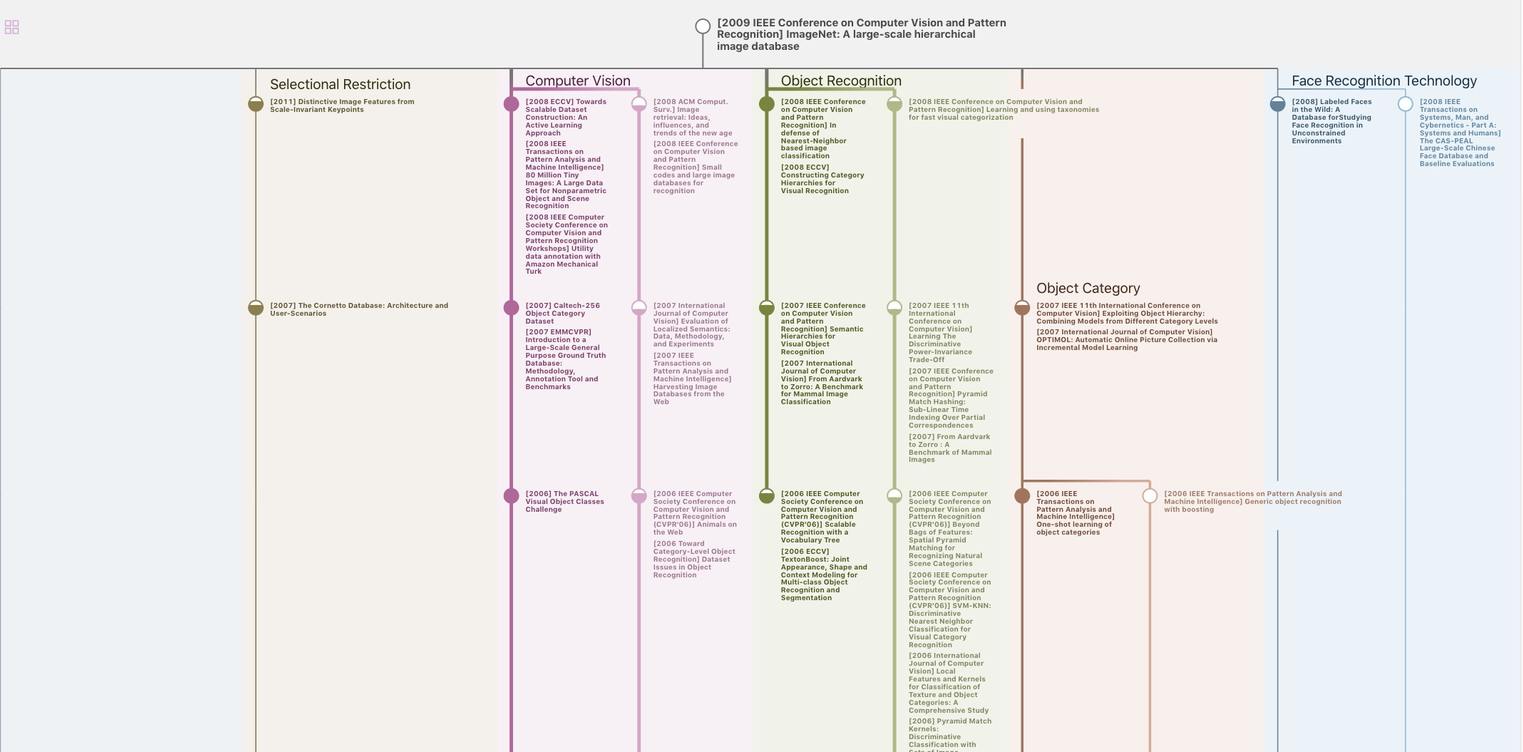
生成溯源树,研究论文发展脉络
Chat Paper
正在生成论文摘要