CoCoPRED: coiled-coil protein structural feature prediction from amino acid sequence using deep neural networks
BIOINFORMATICS(2022)
摘要
Motivation: Coiled-coil is composed of two or more helices that are wound around each other. It widely exists in proteins and has been discovered to play a variety of critical roles in biology processes. Generally, there are three types of structural features in coiled-coil: coiled-coil domain (CCD), oligomeric state and register. However, most of the existing computational tools only focus on one of them. Results: Here, we describe a new deep learning model, CoCoPRED, which is based on convolutional layers, bidirectional long short-term memory, and attention mechanism. It has three networks, i.e. CCD network, oligomeric state network, and register network, corresponding to the three types of structural features in coiled-coil. This means CoCoPRED has the ability of fulfilling comprehensive prediction for coiled-coil proteins. Through the 5-fold crossvalidation experiment, we demonstrate that CoCoPRED can achieve better performance than the state-of-the-art models on both CCD prediction and oligomeric state prediction. Further analysis suggests the CCD prediction may be a performance indicator of the oligomeric state prediction in CoCoPRED. The attention heads in CoCoPRED indicate that registers a, b and e are more crucial for the oligomeric state prediction.
更多查看译文
AI 理解论文
溯源树
样例
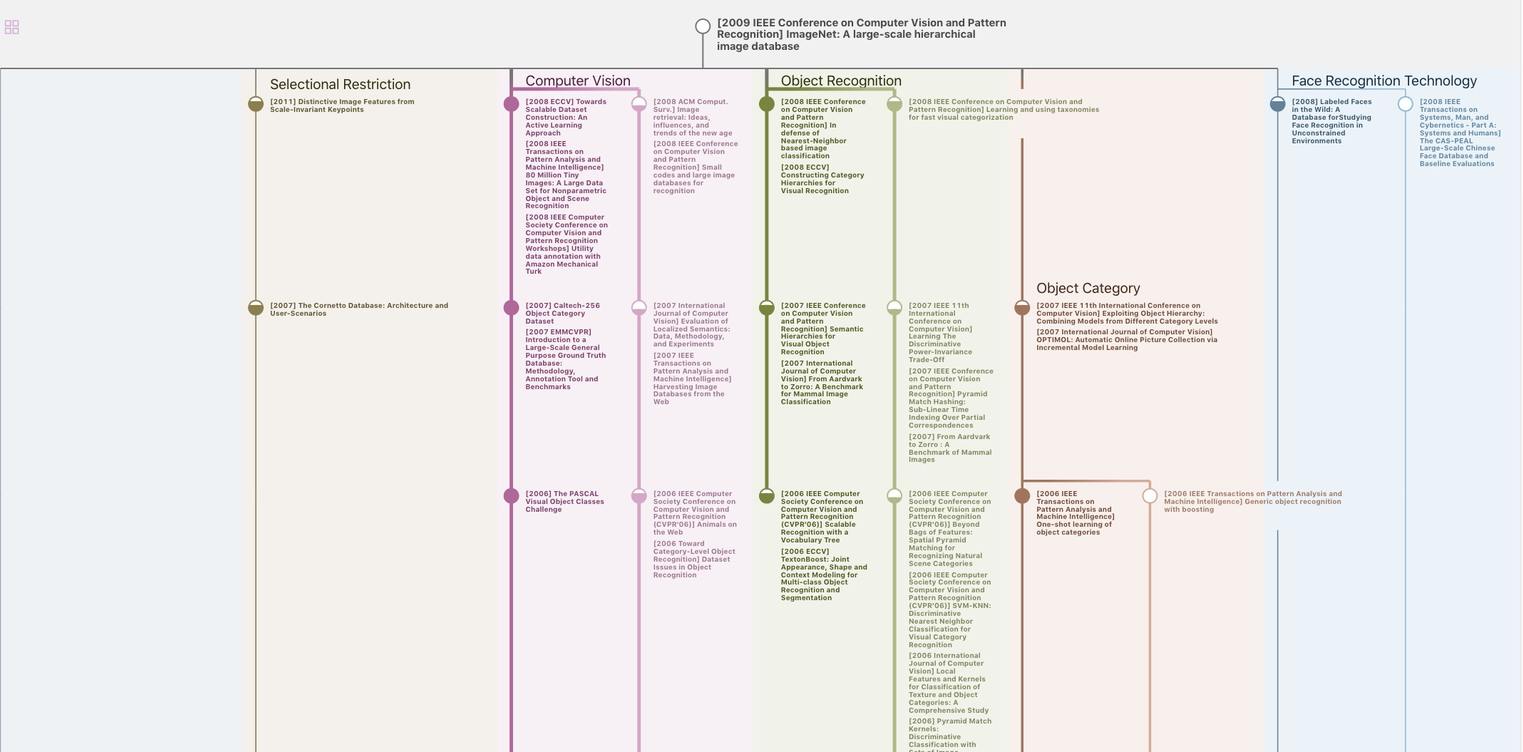
生成溯源树,研究论文发展脉络
Chat Paper
正在生成论文摘要