Practical and Light-weight Secure Aggregation for Federated Submodel Learning
arxiv(2021)
摘要
Recently, Niu, et. al. introduced a new variant of Federated Learning (FL), called Federated Submodel Learning (FSL). Different from traditional FL, each client locally trains the submodel (e.g., retrieved from the servers) based on its private data and uploads a submodel at its choice to the servers. Then all clients aggregate all their submodels and finish the iteration. Inevitably, FSL introduces two privacy-preserving computation tasks, i.e., Private Submodel Retrieval (PSR) and Secure Submodel Aggregation (SSA). Existing work fails to provide a loss-less scheme, or has impractical efficiency. In this work, we leverage Distributed Point Function (DPF) and cuckoo hashing to construct a practical and light-weight secure FSL scheme in the two-server setting. More specifically, we propose two basic protocols with few optimisation techniques, which ensures our protocol practicality on specific real-world FSL tasks. Our experiments show that our proposed protocols can finish in less than 1 minute when weight sizes $\leq 2^{15}$, we also demonstrate protocol efficiency by comparing with existing work and by handling a real-world FSL task.
更多查看译文
关键词
federated submodel
AI 理解论文
溯源树
样例
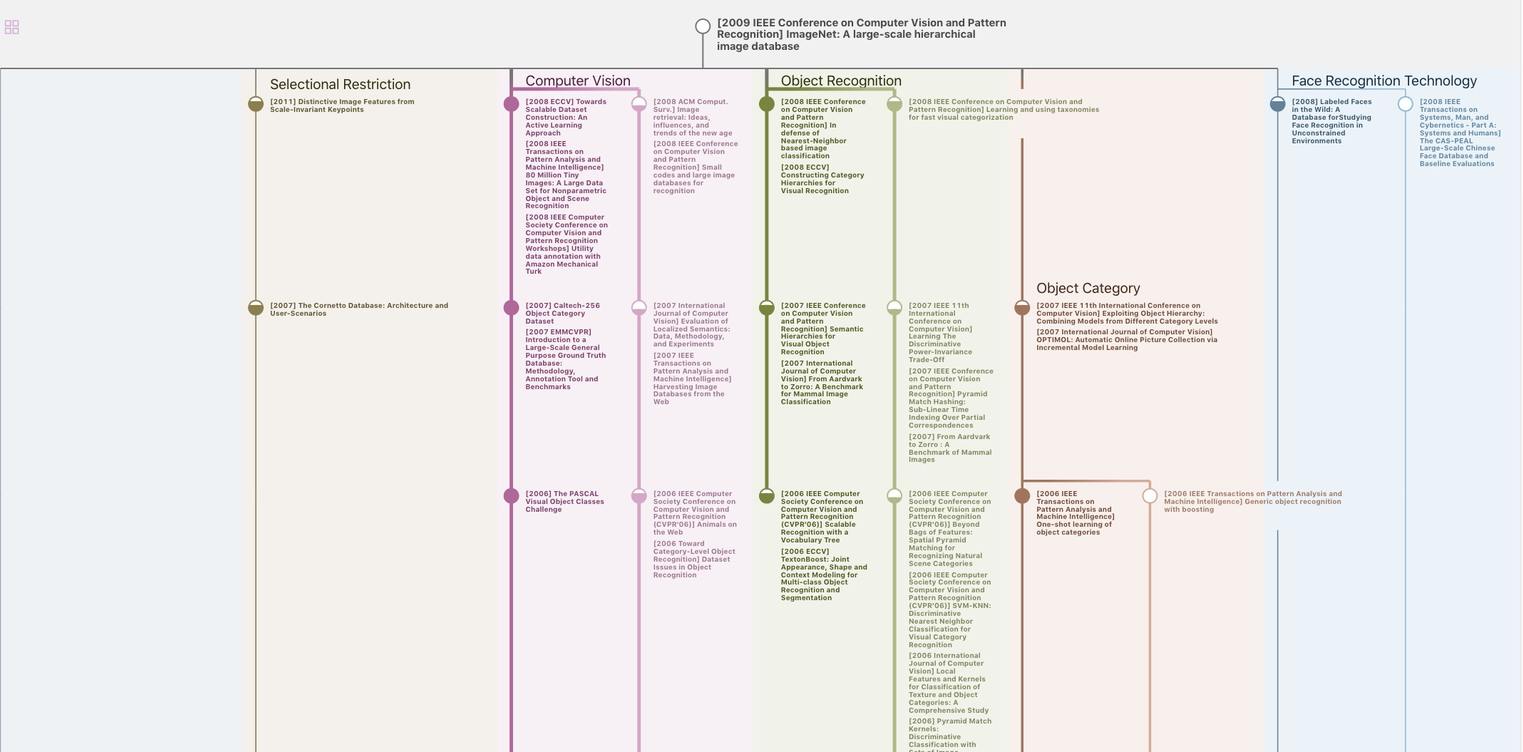
生成溯源树,研究论文发展脉络
Chat Paper
正在生成论文摘要