Learning Circular Hidden Quantum Markov Models: A Tensor Network Approach
arxiv(2021)
摘要
In this paper, we propose circular Hidden Quantum Markov Models (c-HQMMs), which can be applied for modeling temporal data in quantum datasets (with classical datasets as a special case). We show that c-HQMMs are equivalent to a constrained tensor network (more precisely, circular Local Purified State with positive-semidefinite decomposition) model. This equivalence enables us to provide an efficient learning model for c-HQMMs. The proposed learning approach is evaluated on six real datasets and demonstrates the advantage of c-HQMMs on multiple datasets as compared to HQMMs, circular HMMs, and HMMs.
更多查看译文
关键词
quantum,models,learning
AI 理解论文
溯源树
样例
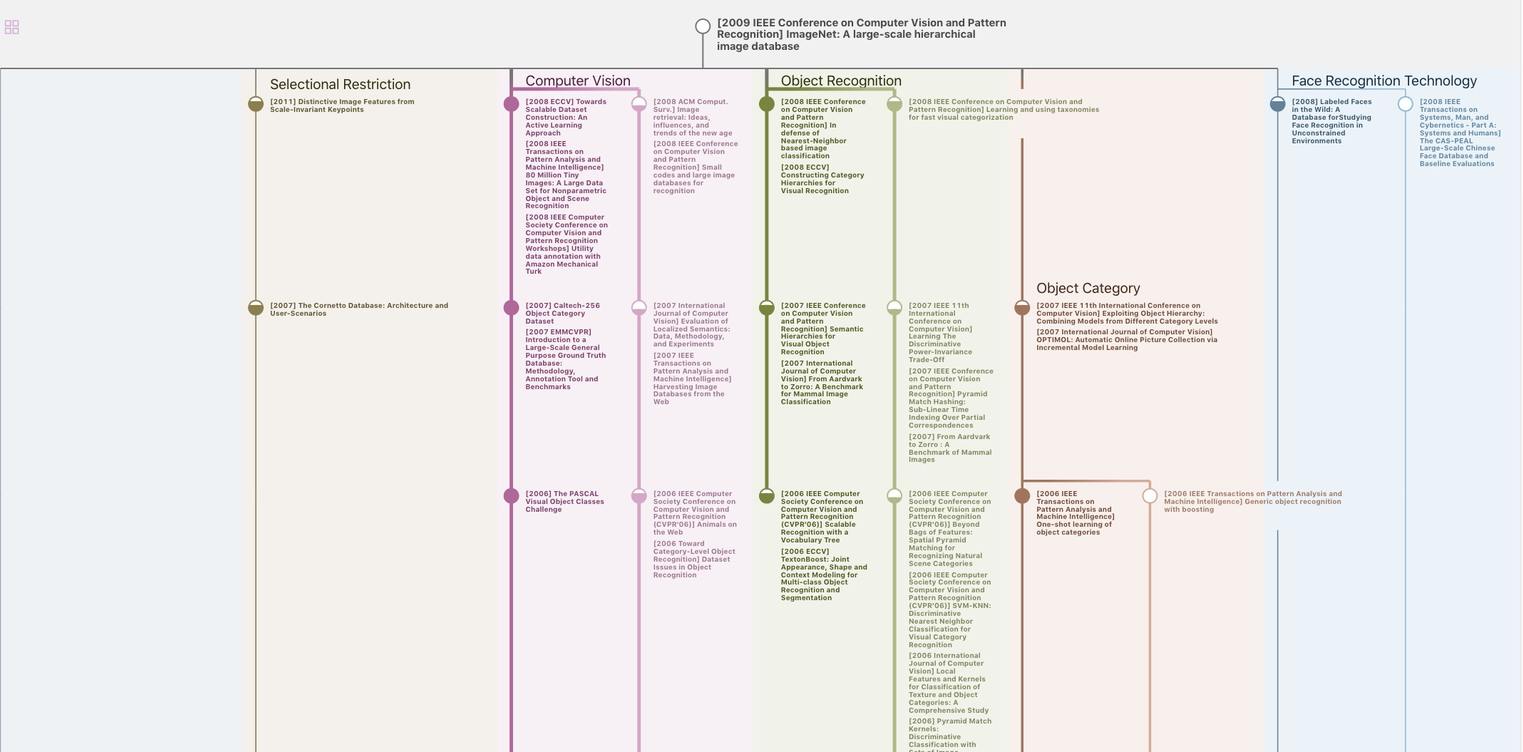
生成溯源树,研究论文发展脉络
Chat Paper
正在生成论文摘要