MARS-Net: Deep learning-based segmentation pipeline for profiling cellular morphodynamics from multiple types of live cell microscopy
biorxiv(2021)
摘要
Quantitative studies of cellular morphodynamics rely on extracting leading-edge velocity time-series based on accurate cell segmentation from live cell imaging. However, live cell imaging has numerous challenging issues about accurate edge localization. Here, we develop a deep learning-based pipeline, termed MARS-Net (Multiple-microscopy- type-based Accurate and Robust Segmentation Network), that utilizes transfer learning and the datasets from multiple types of microscopy to localize cell edges with high accuracy, allowing quantitative profiling of cellular morphodynamics. For effective training with the datasets from multiple types of live cell microscopy, we integrated the pretrained VGG-19 encoder with U-Net decoder and added dropout layers. Using this structure, we were able to train one neural network model that can accurately segment various live cell movies from phase contrast, spinning disk confocal, and total internal reflection fluorescence microscopes. Intriguingly, MARS-Net produced more accurate edge localization than the neural network models trained with single microscopy type datasets, whereas the standard U-Net could not increase the overall accuracy. We expect that MARS-Net can accelerate the studies of cellular morphodynamics by providing accurate segmentation of challenging live cell images.
### Competing Interest Statement
The authors have declared no competing interest.
更多查看译文
AI 理解论文
溯源树
样例
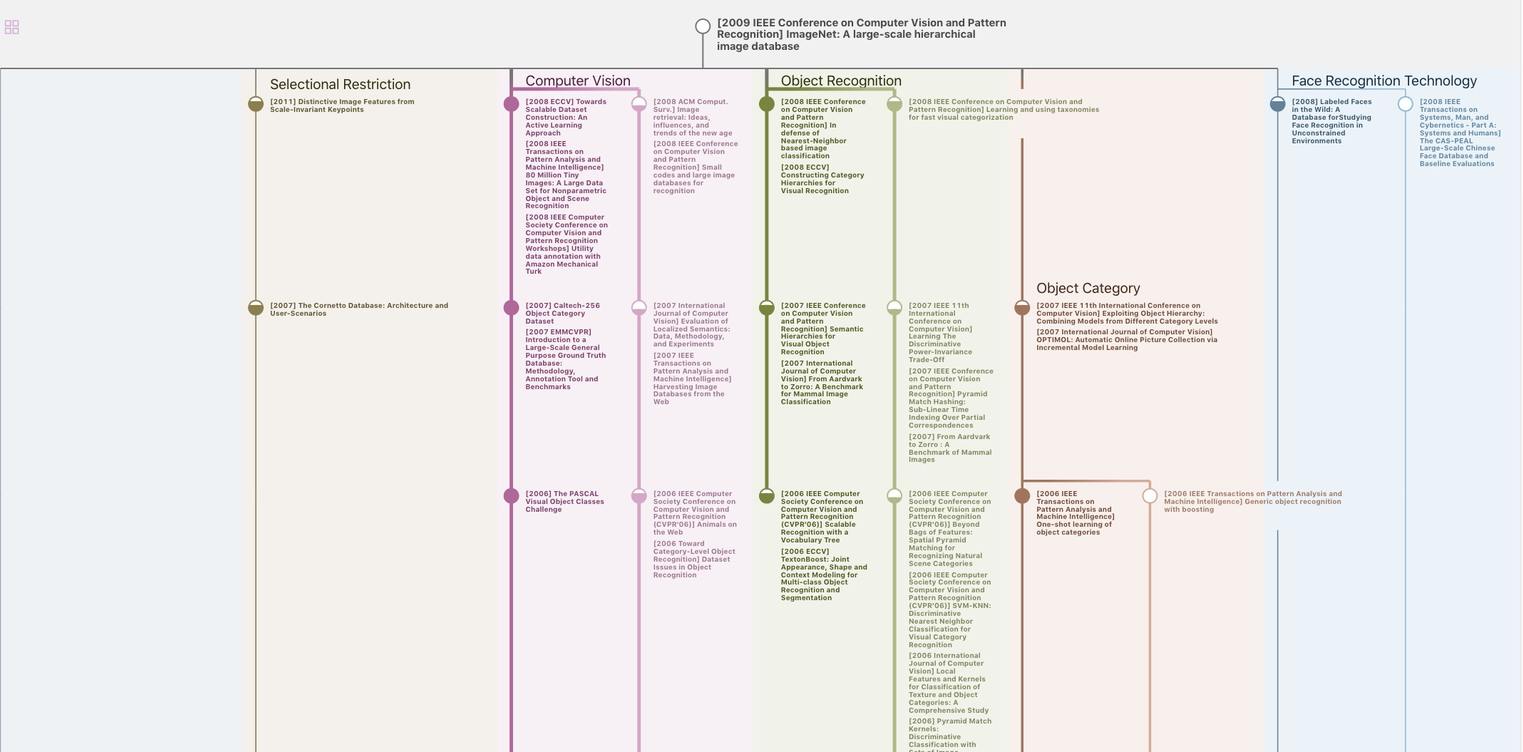
生成溯源树,研究论文发展脉络
Chat Paper
正在生成论文摘要