Performance reserves in brain-imaging-based phenotype prediction
biorxiv(2024)
摘要
Machine learning studies have shown that various phenotypes can be predicted from structural and functional brain images. However, in most such studies, prediction performance ranged from moderate to disappointing. It is unclear whether prediction performance will substantially improve with larger sample sizes or whether insufficient predictive information in brain images impedes further progress. Here, we systematically assess the effect of sample size on prediction performance using sample sizes far beyond what is possible in common neuroimaging studies. We project 3-9 fold improvements in prediction performance for behavioral and mental health phenotypes when moving from one thousand to one million samples. Moreover, we find that moving from single imaging modalities to multimodal input data can lead to further improvements in prediction performance, often on par with doubling the sample size. Our analyses reveal considerable performance reserves for neuroimaging-based phenotype prediction. Machine learning models may benefit much more from extremely large neuroimaging datasets than currently believed.
### Competing Interest Statement
The authors have declared no competing interest.
更多查看译文
关键词
prediction,performance reserves,brain-imaging-based
AI 理解论文
溯源树
样例
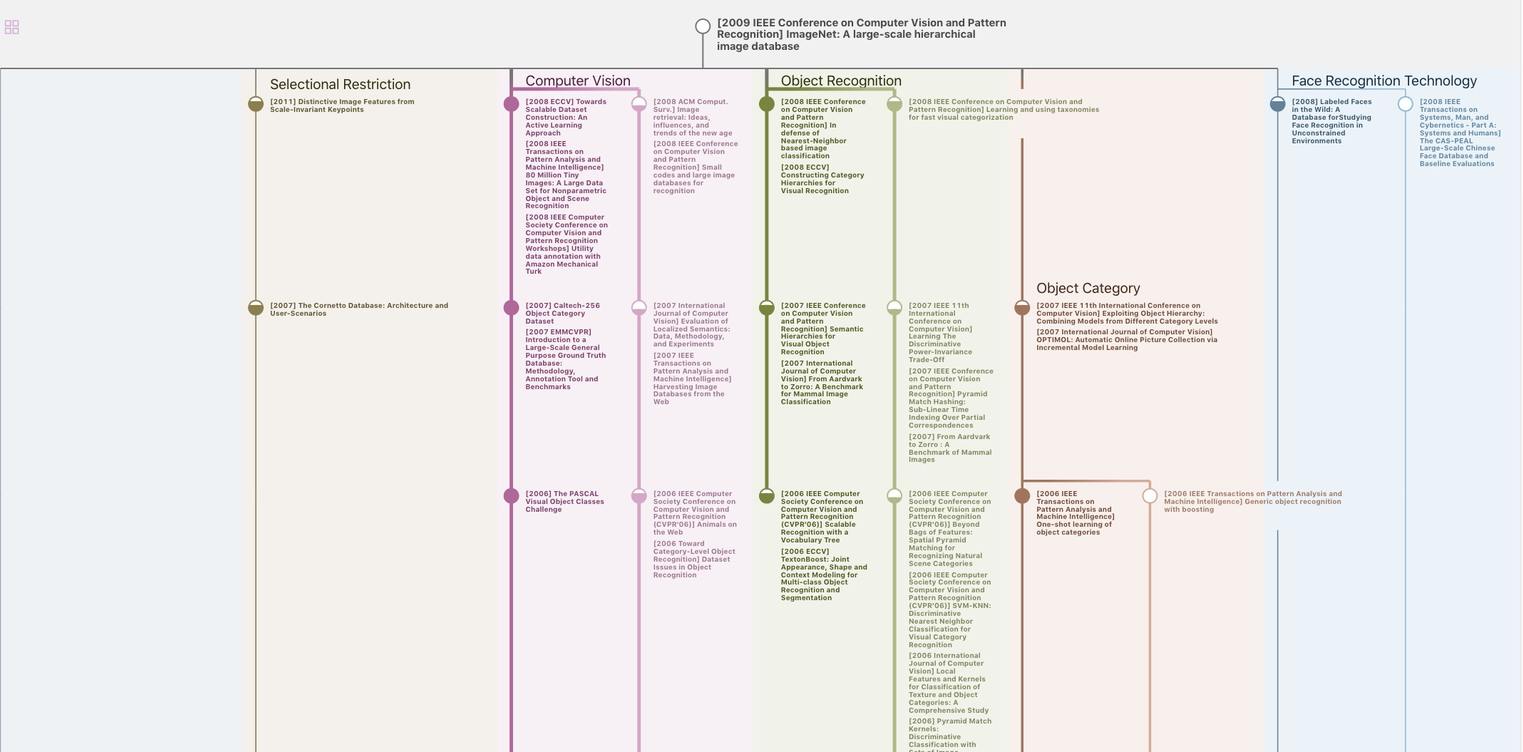
生成溯源树,研究论文发展脉络
Chat Paper
正在生成论文摘要