Using weak supervision to generate training datasets from social media data: a proof of concept to identify drug mentions
Neural computing & applications(2021)
摘要
Twitter has been a remarkable resource for research in pharmacovigilance in the last decade. Traditionally, rule- or lexicon-based methods have been utilized for automatically extracting drug tweets for human annotation. The process of human annotation to create labeled sets for machine learning models is laborious, time consuming and not scalable. In this work, we demonstrate the feasibility of applying weak supervision (noisy labeling) to select drug data, and build machine learning models using large amounts of noisy labeled data instead of limited gold standard labelled sets. Our results demonstrate the models built with large amounts of noisy data achieve similar performance than models trained on limited gold standard datasets, hence demonstrating that weak supervision helps reduce the need to rely on manual annotation, allowing more data to be easily labeled and useful for downstream machine learning applications, in this case drug mention identification.
更多查看译文
关键词
Weak supervision,Noisy learning,Pharmacovigilance,Twitter
AI 理解论文
溯源树
样例
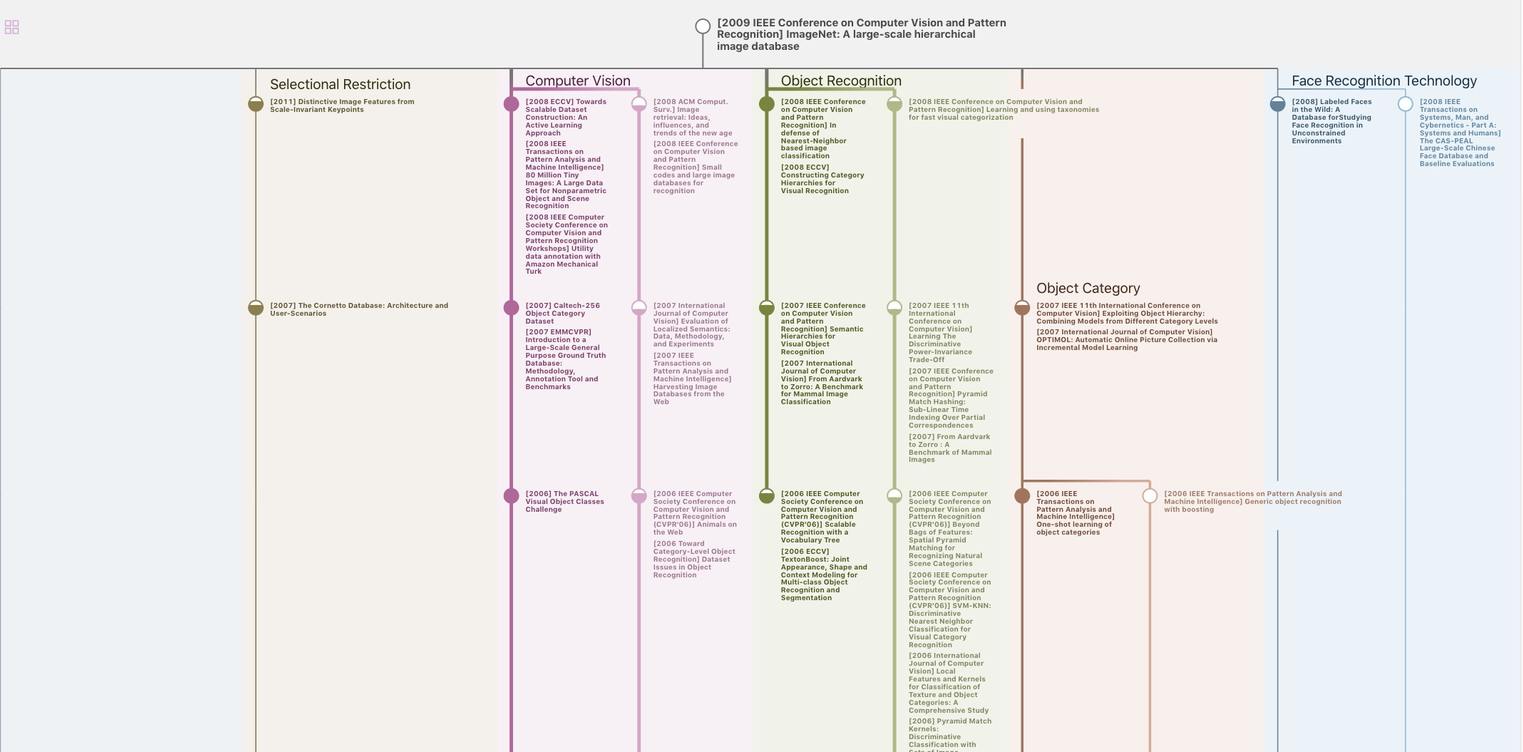
生成溯源树,研究论文发展脉络
Chat Paper
正在生成论文摘要