Geometric Heuristics for Transfer Learning in Decision Trees
Conference on Information and Knowledge Management(2021)
摘要
ABSTRACTMotivated by a network fault detection problem, we study how recall can be boosted in a decision tree classifier, without sacrificing too much precision. This problem is relevant and novel in the context of transfer learning(TL), in which few target domain training samples are available. We define a geometric optimization problem for boosting the recall of a decision tree classifier, and show it is NP-hard. To solve it efficiently, we propose several near-linear time heuristics, and experimentally validate these heuristics in the context of TL. Our evaluation includes 7 public datasets, as well as 6 network fault datasets, and we compare our heuristics with several existing TL algorithms, as well as exact mixed integer linear programming(MILP) solutions to our optimization problem. We find that our heuristics boost recall in a manner similar to optimal MILP solutions, yet require several orders of magnitude less compute time. In many cases the F1 score of our approach is competitive, and often better, than other TL algorithms. Moreover, our approach can be used as a building block to apply transfer learning to more powerful ensemble methods, such as random forests.
更多查看译文
AI 理解论文
溯源树
样例
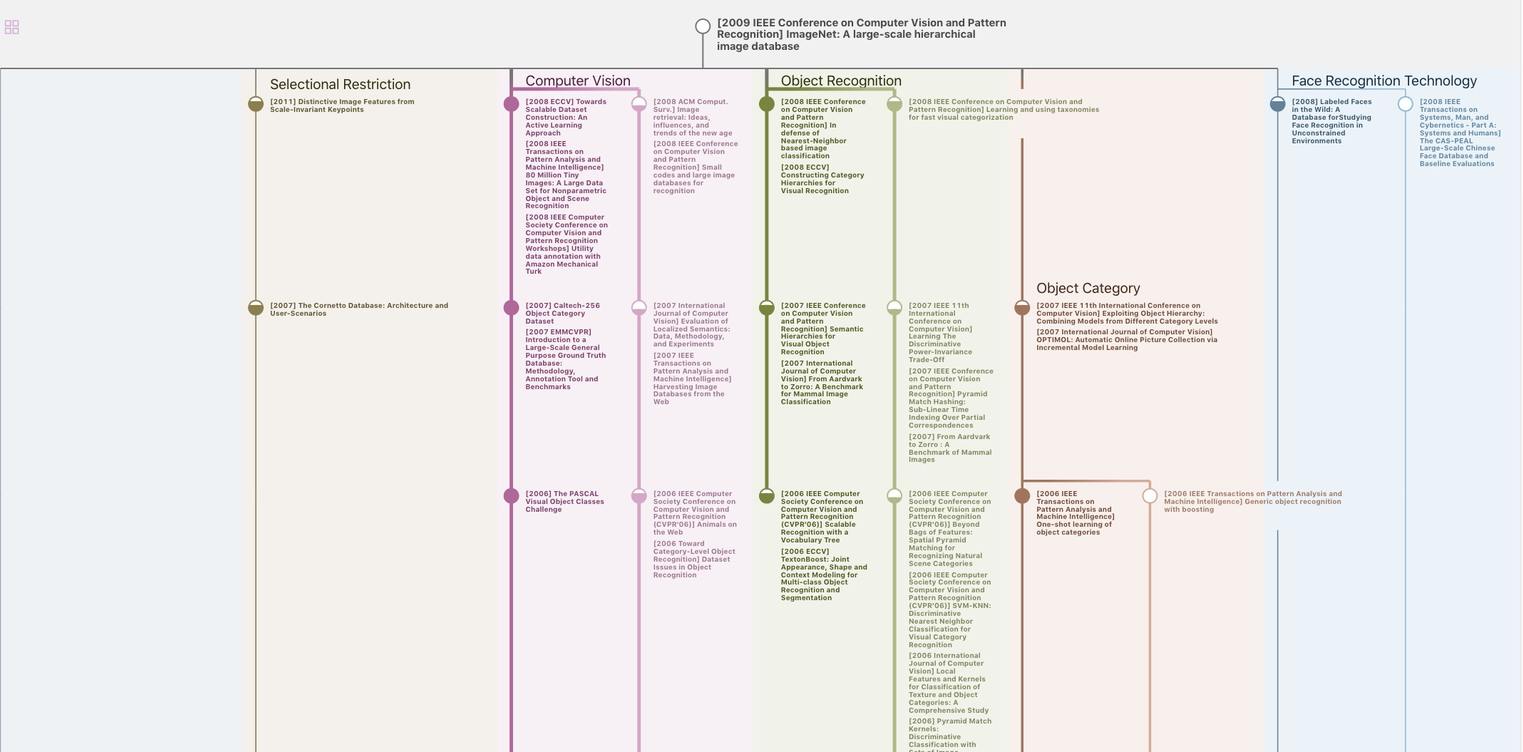
生成溯源树,研究论文发展脉络
Chat Paper
正在生成论文摘要