Robust Road Network Representation Learning: When Traffic Patterns Meet Traveling Semantics
Conference on Information and Knowledge Management(2021)
摘要
ABSTRACTIn this work, we propose a robust road network representation learning framework called Toast, which comes to be a cornerstone to boost the performance of numerous demanding transport planning tasks. Specifically, we first propose a traffic context aware skip-gram module to incorporate auxiliary tasks of predicting the traffic context of a target road segment. Furthermore, we propose a trajectory-enhanced Transformer module that utilizes trajectory data to extract traveling semantics on road networks. Apart from obtaining effective road segment representations, this module also enables us to obtain the route representations. With these two modules, we can learn representations which can capture multi-faceted characteristics of road networks to be applied in both road segment based applications and trajectory based applications. Last, we design a benchmark containing four typical transport planning tasks to evaluate the usefulness of Toast and comprehensive experiments verify that Toast consistently outperforms the state-of-the-art baselines across all tasks.
更多查看译文
AI 理解论文
溯源树
样例
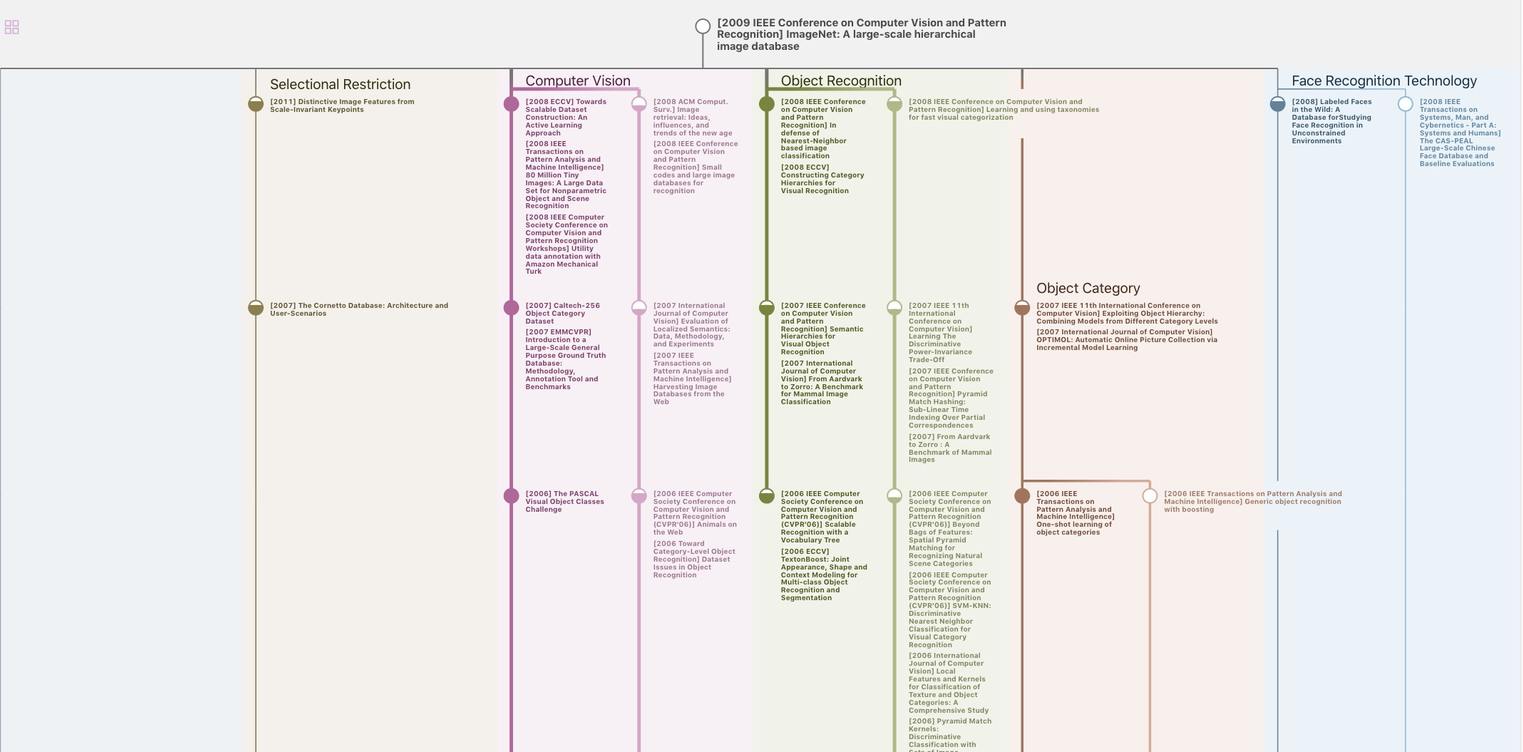
生成溯源树,研究论文发展脉络
Chat Paper
正在生成论文摘要