Mining Bursty Groups from Interaction Data
Conference on Information and Knowledge Management(2021)
摘要
ABSTRACTEmpirical studies and theoretical models both highlight burstinessas a common temporal pattern in online behavior. A key driver for burstiness is the self-exciting nature of online interactions. For example, posts in online groups often incite posts in response. Such temporal dependencies are easily lost when interaction data is aggregated in snapshots which are subsequently analyzed independently. An alternative is to model individual interactions as a multi-dimensional self-exciting process, thus, enforcing both temporal and network dependencies. Point processes, however, are challenging to employ for large real-world datasets as fitting them incurs super-linear cost in the number of events. How can we efficiently detect online groups exhibiting bursty self-exciting temporal behavior in large real-world datasets? We propose a bursty group detection framework, called MYRON, which explicitly models self-exciting behavior within groups while also accounting for network-wide baseline activity. MYRON imposes bursty temporal structure within a scalable tensor factorization framework to decouple within-group interactions as interpretable factors. Our framework can incorporate different "shapes"of temporal burstiness via wavelet decomposition or kernels forself-exciting behavior. Our evaluation on both synthetic and real-world data demonstrates MYRON's utility in community detection.It is up to 40% more effective in detecting ground truth groups compared to state-of-the-art baselines. In addition, MYRON is able to uncover interpretable bursty patterns of behavior from user-photo interactions in Flickr.
更多查看译文
AI 理解论文
溯源树
样例
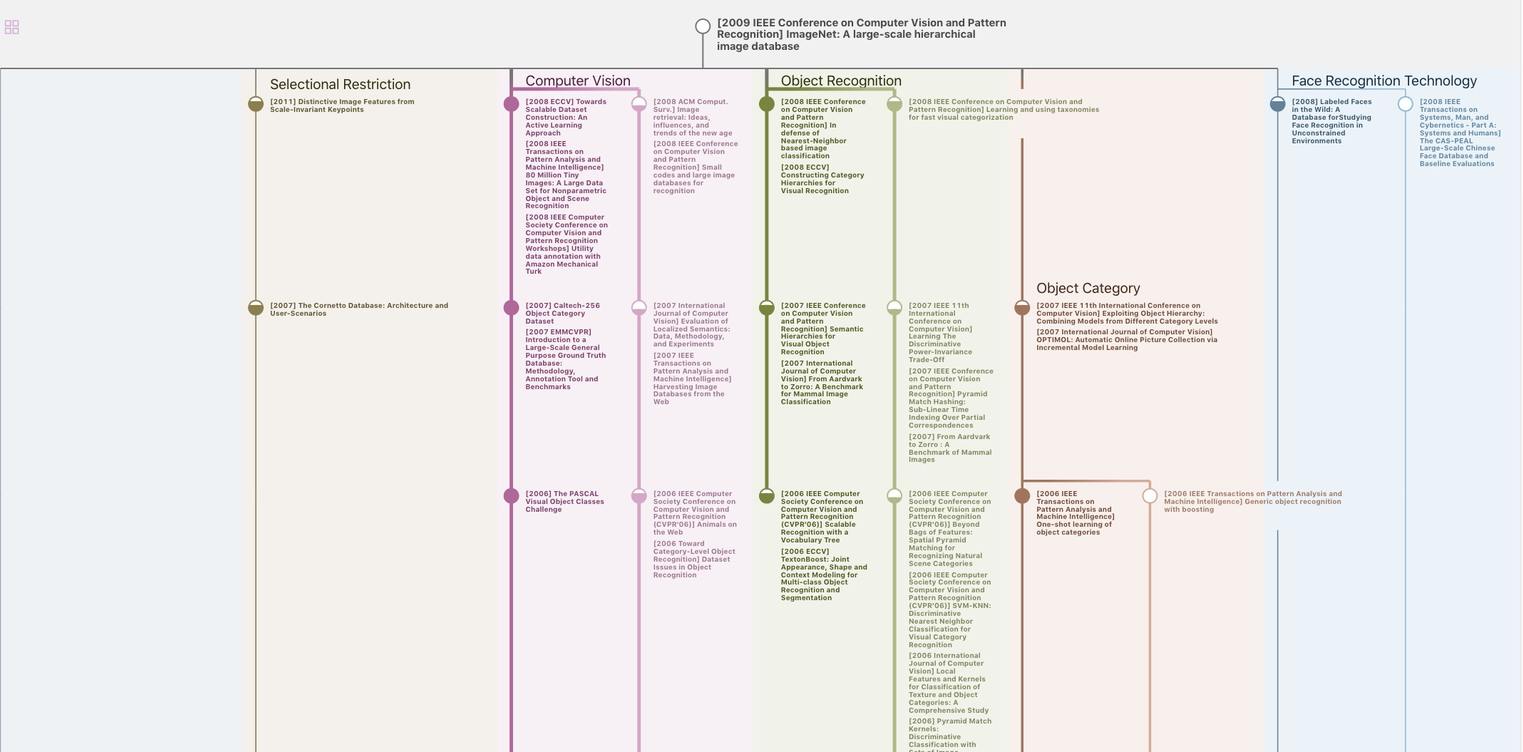
生成溯源树,研究论文发展脉络
Chat Paper
正在生成论文摘要