Mining Cross Features for Financial Credit Risk Assessment
Conference on Information and Knowledge Management(2021)
摘要
ABSTRACTFor reliability, machine learning models in some areas, e.g., finance and healthcare, require to be both accurate and globally interpretable. Among them, credit risk assessment is a major application of machine learning for financial institutions to evaluate credit of users and detect default or fraud. Simple white-box models, such as Logistic Regression (LR), are usually used for credit risk assessment, but not powerful enough to model complex nonlinear interactions among features. In contrast, complex black-box models are powerful at modeling, but lack of interpretability, especially global interpretability. Fortunately, automatic feature crossing is a promising way to find cross features to make simple classifiers to be more accurate without heavy handcrafted feature engineering. However, existing automatic feature crossing methods have problems in efficiency on credit risk assessment, for corresponding data usually contains hundreds of feature fields. In this work, we find local interpretations in Deep Neural Networks (DNNs) of a specific feature are usually inconsistent among different samples. We demonstrate this is caused by nonlinear feature interactions in the hidden layers of DNN. Thus, we can mine feature interactions in DNN, and use them as cross features in LR. This will result in mining cross features more efficiently. Accordingly, we propose a novel automatic feature crossing method called DNN2LR. The final model, which is a LR model empowered with cross features, generated by DNN2LR is a white-box model. We conduct experiments on both public and business datasets from real-world credit risk assessment applications, which show that, DNN2LR outperform both conventional models used for credit assessment and several feature crossing methods. Moreover, comparing with state-of-the-art feature crossing methods, i.e., AutoCross, the proposed DNN2LR method accelerates the speed by about 10 to 40 times on financial credit assessment datasets, which contain hundreds of feature fields.
更多查看译文
AI 理解论文
溯源树
样例
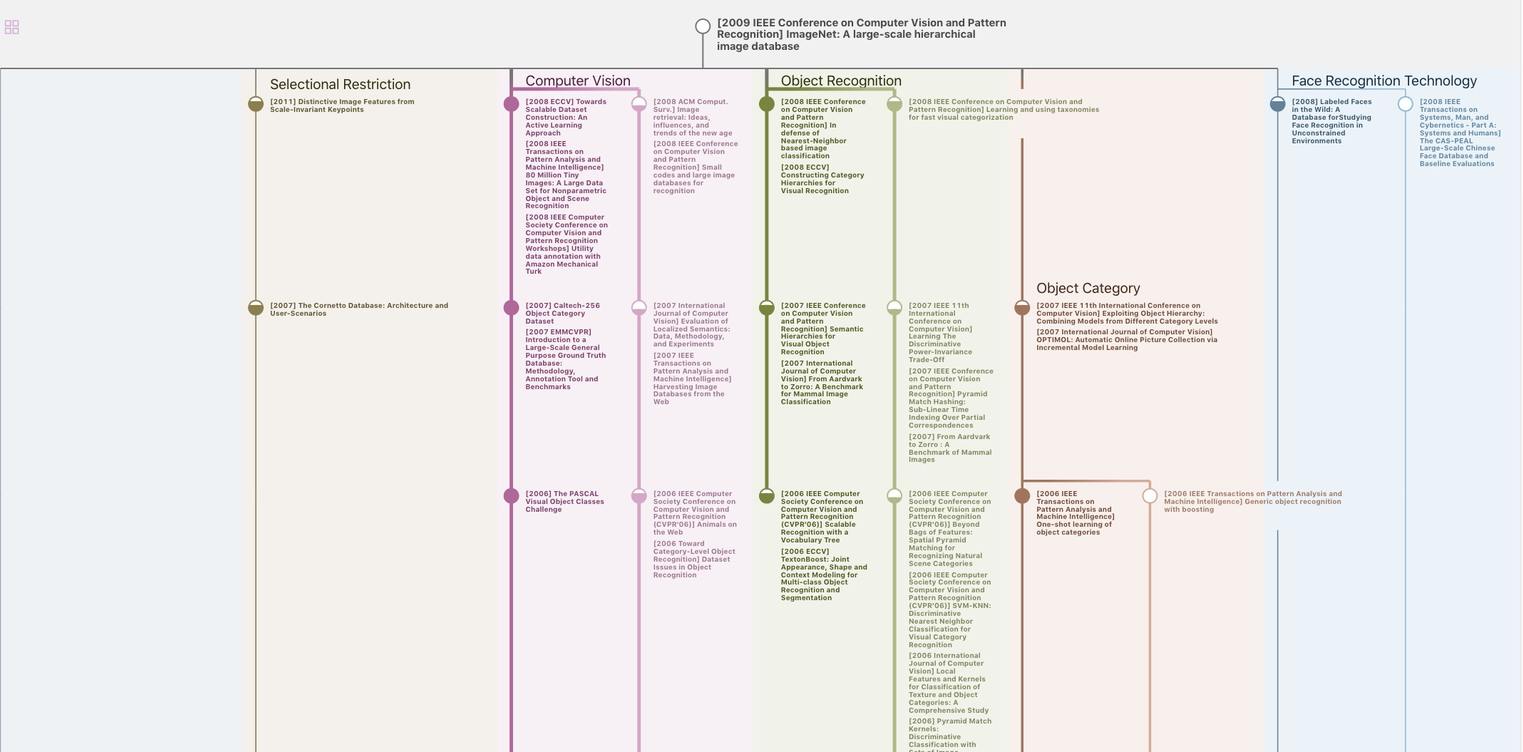
生成溯源树,研究论文发展脉络
Chat Paper
正在生成论文摘要