GDFM: Gene Vectors Embodied Deep Attentional Factorization Machines for Interaction prediction
Conference on Information and Knowledge Management(2021)
摘要
BSTRACTGene Network Graphs (GNGs) are comprised of biomedical data. Deriving structural information from these graphs remains a prime area of research in the domain of biomedical and health informatics. In this paper, we propose Gene Vectors Embodied Deep Attentional Factorization Machines (GDFMs) for the gene to gene interaction prediction. We first initialize GDFM with vector embeddings learned from gene locality configuration and an expression equivalence criterion that preserves their innate similar traits. GDFM uses an attention-based mechanism that manipulates different positions, to learn the representation of sequence, before calculating the pairwise factorized interactions. We further use hidden layers, batch normalization, and dropout to stabilize the performance of our deep structured architecture. An extensive comparison with several state-of-the-art approaches, using Ecoli and Yeast datasets for gene-gene interaction prediction shows the significance of our proposed framework.
更多查看译文
AI 理解论文
溯源树
样例
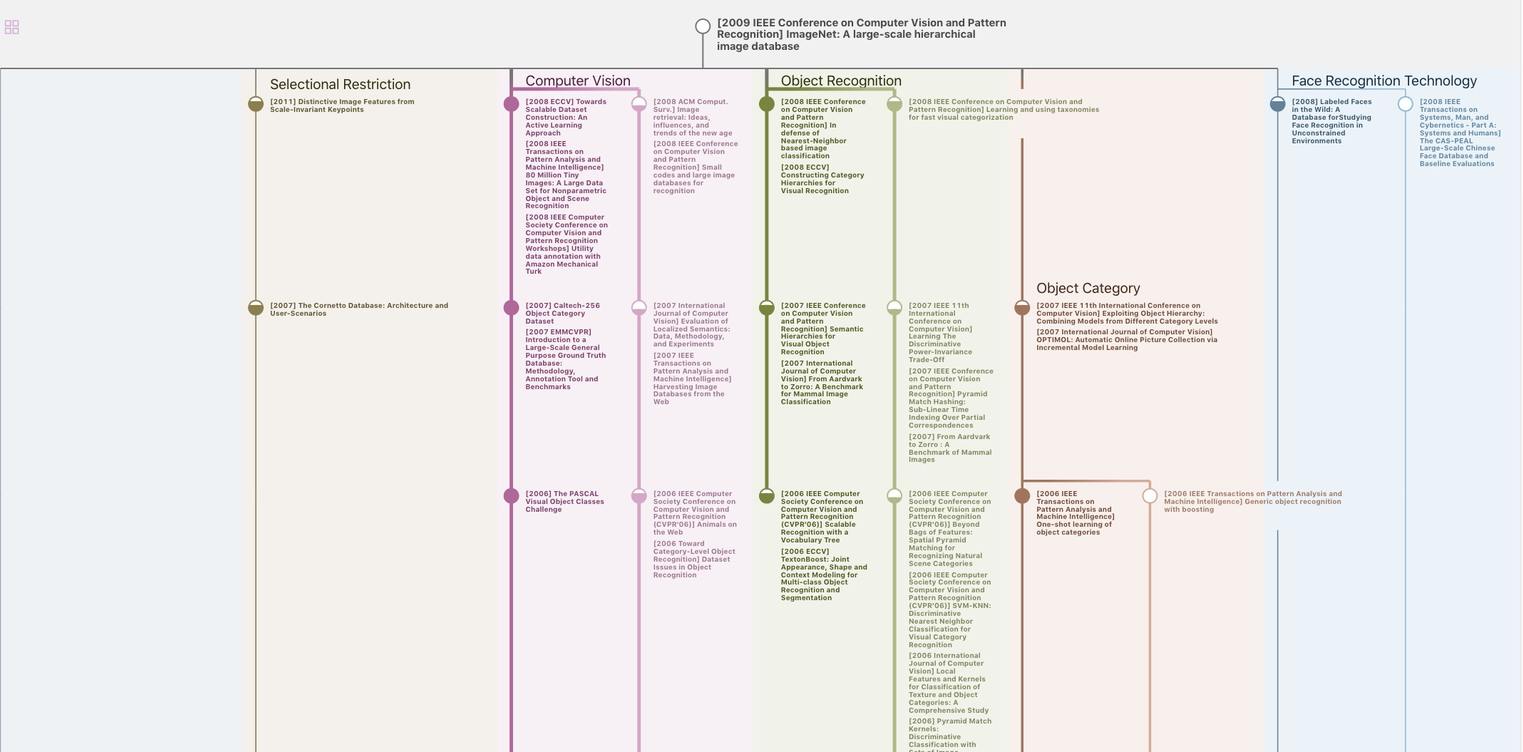
生成溯源树,研究论文发展脉络
Chat Paper
正在生成论文摘要