Semi-supervised Multi-label Learning for Graph-structured Data
Conference on Information and Knowledge Management(2021)
摘要
ABSTRACTThe semi-supervised multi-label classification problem primarily deals with Euclidean data, such as text with a 1D grid of tokens and images with a 2D grid of pixels. However, the non-Euclidean graph-structured data naturally and constantly appears in semi-supervised multi-label learning tasks from various domains like social networks, citation networks, and protein-protein interaction (PPI) networks. Moreover, the existing popular node embedding methods, like Graph Neural Networks (GNN), focus on graphs with simplex labels and tend to neglect label correlations in the multi-label setting, so the easy adaption proves empirically ineffective. Therefore, graph representation learning for the semi-supervised multi-label learning task is crucial and challenging. In this work, we incorporate the idea of label embedding into our proposed model to capture both network topology and higher-order multi-label correlations. The label embedding is generated along with the node embedding based on the topological structure to serve as the prototype center for each class. Moreover, the similarity of the label embedding and node embedding can be used as a confidence vector to guide the label smoothing process, formulating as a margin ranking optimization problem to learn the second-order relations between labels. Extensive experiments on real-world datasets from various domains demonstrate that our model significantly outperforms the state-of-the-art models for node-level tasks.
更多查看译文
AI 理解论文
溯源树
样例
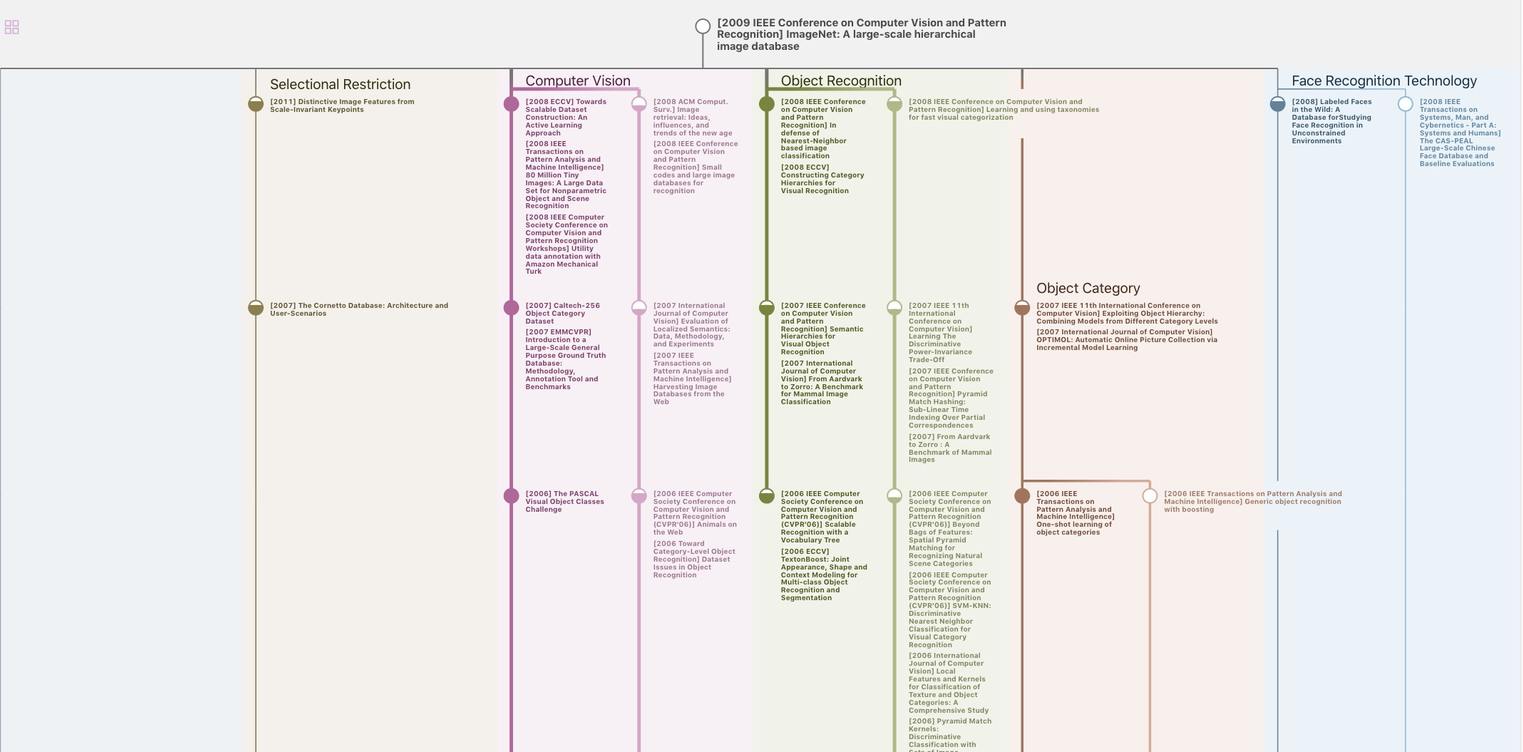
生成溯源树,研究论文发展脉络
Chat Paper
正在生成论文摘要