Cycle or Minkowski: Which is More Appropriate for Knowledge Graph Embedding?
Conference on Information and Knowledge Management(2021)
摘要
ABSTRACTKnowledge graph (KG) embedding aims to encode entities and relations into low-dimensional vector spaces, in turn, can support various machine learning models on KG related tasks with good performance. However, existing methods for knowledge graph embedding fail to consider the influence of the embedding space, which makes them still unsatisfactory in practical applications. In this study, we try to improve the expressiveness of the embedding space from the perspective of the metric. Specifically, we first point out the implications of Minkowski metric used in KG embedding and then make a quantitative analysis. To solve the limitations, we introduce a new metric, named Cycle metric, based on the oscillation property of the periodic function. Furthermore, we find that the function period has a significant influence on the expressiveness of the embedding space. Given a fully trained model, the smaller the period, the better the expressive ability. Finally, to validate the findings, we propose a new model, named CyclE by combining Cycle Metric and the popular KG embeddings models. Comprehensive experimental results show that Cycle is more appropriate than Minkowski for KG embedding.
更多查看译文
关键词
cycle,minkowski
AI 理解论文
溯源树
样例
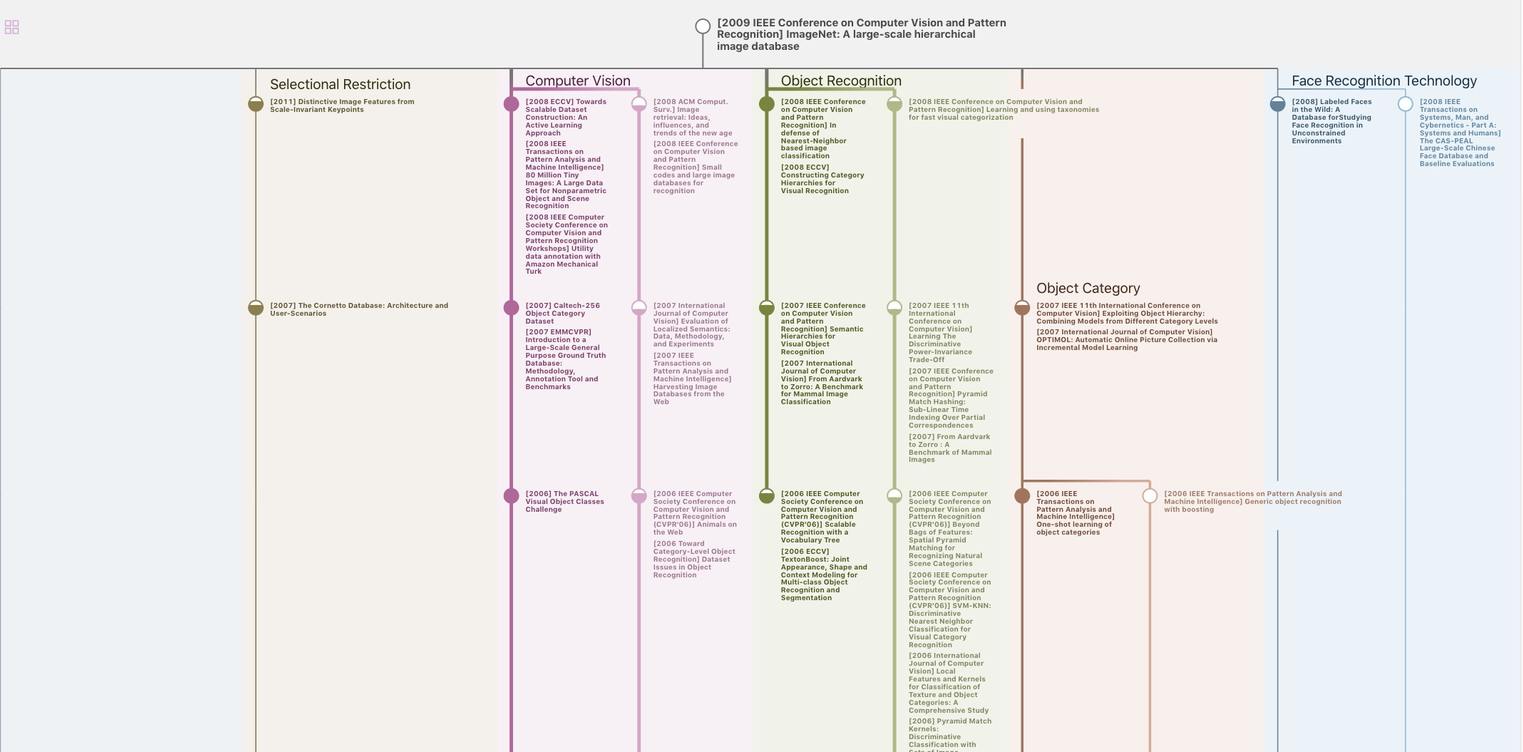
生成溯源树,研究论文发展脉络
Chat Paper
正在生成论文摘要