Projective Ranking: A Transferable Evasion Attack Method on Graph Neural Networks
Conference on Information and Knowledge Management(2021)
摘要
BSTRACTGraph Neural Networks (GNNs) have emerged as a series of effective learning methods for graph-related tasks. However, GNNs are shown vulnerable to adversarial attacks, where attackers can fool GNNs into making wrong predictions on adversarial samples with well-designed perturbations. Specifically, we observe that the current evasion attacks suffer from two limitations: (1) the attack strategy based on the reinforcement learning method might not be transferable when the attack budget changes; (2) the greedy mechanism in the vanilla gradient-based method ignores the long-term benefits of each perturbation operation. In this paper, we propose a new attack method named projective ranking to overcome the above limitations. Our idea is to learn a powerful attack strategy considering the long-term benefits of perturbations, then adjust it as little as possible to generate adversarial samples under different budgets. We further employ mutual information to measure the long-term benefits of each perturbation and rank them accordingly, so the learned attack strategy has better attack performance. Our method dramatically reduces the adaptation cost of learning a new attack strategy by projecting the attack strategy when the attack budget changes. Our preliminary evaluation results in synthesized and real-world datasets demonstrate that our method owns powerful attack performance and effective transferability.
更多查看译文
AI 理解论文
溯源树
样例
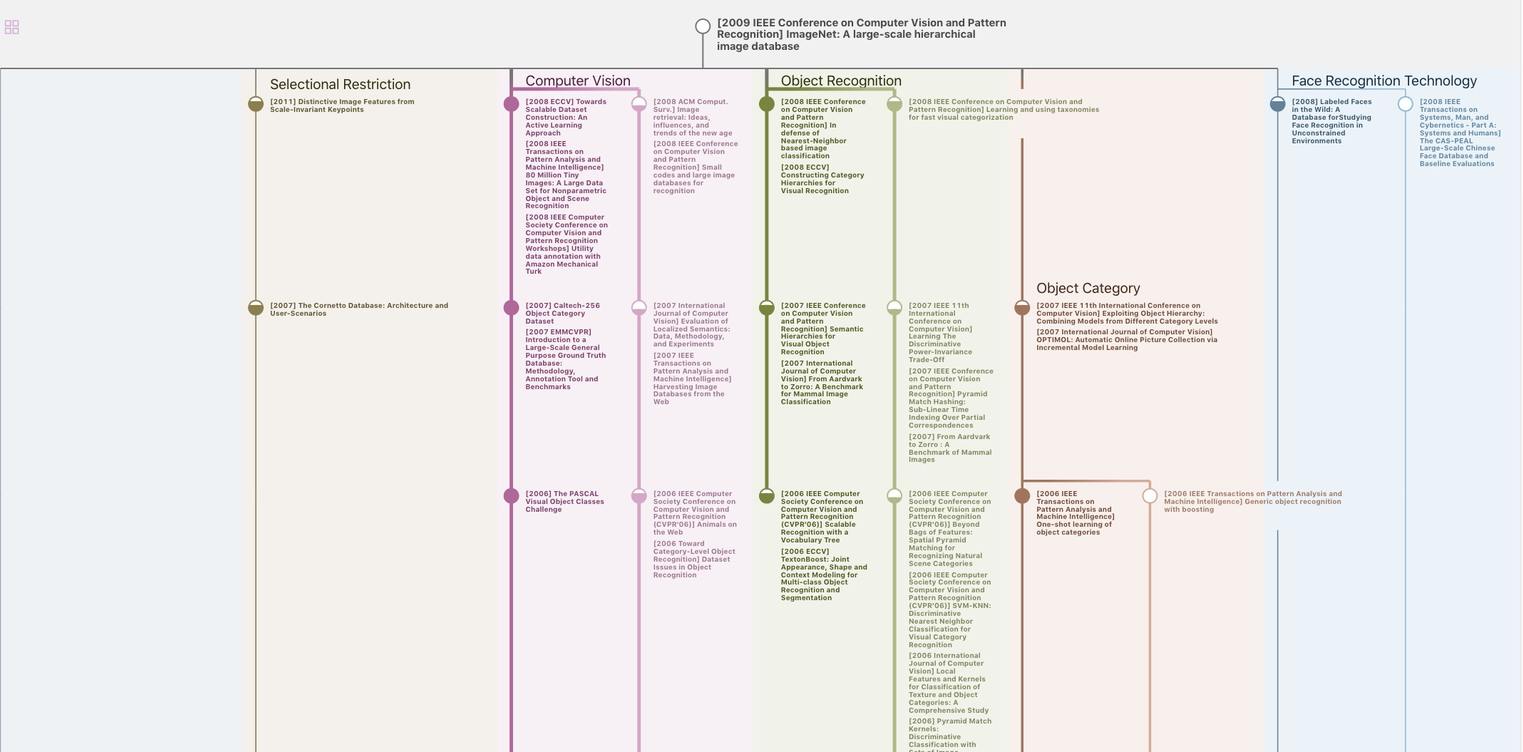
生成溯源树,研究论文发展脉络
Chat Paper
正在生成论文摘要