Tabular Data Concept Type Detection Using Star-Transformers
Conference on Information and Knowledge Management(2021)
摘要
BSTRACTTabular data is an invaluable information resource for search, in-formation extraction and question answering about the world. It is critical to understand the semantic concept types for table columns in order to fully exploit the information in tabular data. In this paper, we focus on learning-based approaches for column concept type detection without relying on any metadata or queries to existing knowledge bases. We propose a model that employs both statistical and semantic features of table columns, and use Star-Transformers to gather and scatter information across the whole table to boost the performance on individual columns. We apply distant supervision to construct a tabular dataset with columns annotated with DBpedia classes. Our experiment results show that our model achieves 93.57 accuracy on the dataset, exceeding that of the state-of-the-art baselines.
更多查看译文
AI 理解论文
溯源树
样例
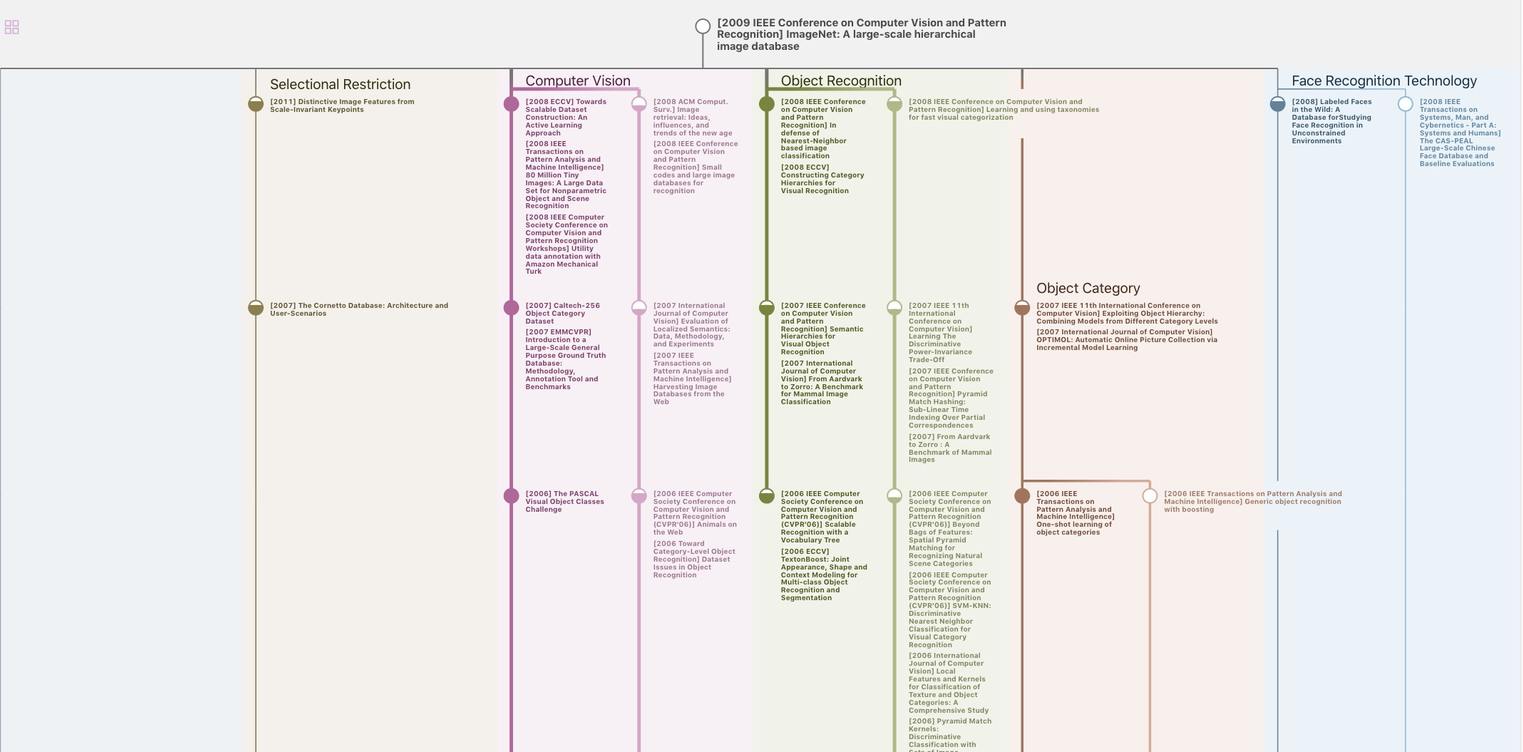
生成溯源树,研究论文发展脉络
Chat Paper
正在生成论文摘要