Relevance-Based Wireless Resource Allocation for a Machine Learning-Based Centralized Control System
2021 IEEE 32ND ANNUAL INTERNATIONAL SYMPOSIUM ON PERSONAL, INDOOR AND MOBILE RADIO COMMUNICATIONS (PIMRC)(2021)
摘要
Machine learning (ML) grows to be an inseparable part of future networks and an enabler for internet of things use-cases. Input components of machine learning units (MLU) have different levels of relevancy for determining accurate output. Assuming that attributes of each MLU are transmitted from multiple terminals, we revisit the resource allocation problem for a ML-based centralized control system, considering relevancy in order to provide best effort MLU outcome as key performance indicator. To achieve this goal, we propose a heuristic greedy resource allocation algorithm in combination with lookup tables of various payload requirements. These tables are generated by a Kullback-Leibler divergence (KLD)-based approach for each MLU. The KLD assigns number of quantization bits for input attributes of each terminal considering their relevancy. The proposed resource allocation is applied to a network of inverted pendulums on carts with MLUs as controllers. Simulation results demonstrate significant gains in MLU performance and efficiency in resource utilization when employing the proposed technique comparing with two benchmarks: the greedy maximum sum rate (GMSR) algorithm allocating resources once with equal payload requirements, and once KLD lookup table.
更多查看译文
关键词
Resource allocation, distributed quantization, correlated multiple source, Kullback-Leibler divergence, relevant information, machine learning
AI 理解论文
溯源树
样例
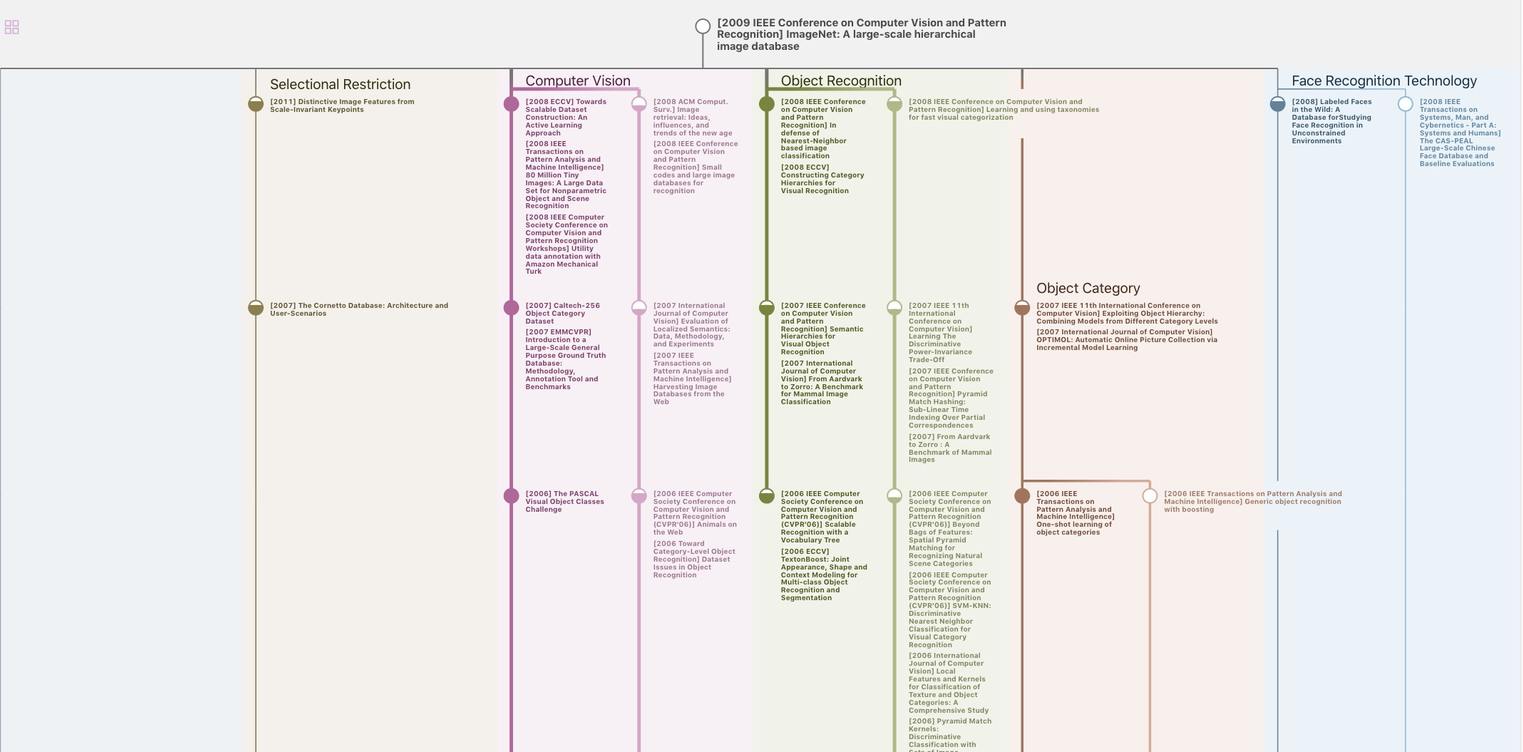
生成溯源树,研究论文发展脉络
Chat Paper
正在生成论文摘要