Moving Target Defense against Adversarial Machine Learning
International Conference on Software Engineering(2021)
摘要
ABSTRACTAs Machine Learning (ML) models are increasingly employed in a number of applications across a multitude of fields, the threat of adversarial attacks against ML models is also increasing. Adversarial samples crafted via specialized attack algorithms have been shown to significantly decrease the performance of ML models. Furthermore, it has also been found that adversarial samples generated for a particular model can transfer across other models, and decrease accuracy and other performance metrics for a model they were not originally crafted for. In recent research, many different defense approaches have been proposed for making ML models robust, ranging from adversarial input re-training to defensive distillation, among others. While these approaches operate at the model-level, we propose an alternate approach to defending ML models against adversarial attacks, using Moving Target Defense (MTD). We formulate the problem and provide preliminary results to showcase the validity of the proposed approach.
更多查看译文
AI 理解论文
溯源树
样例
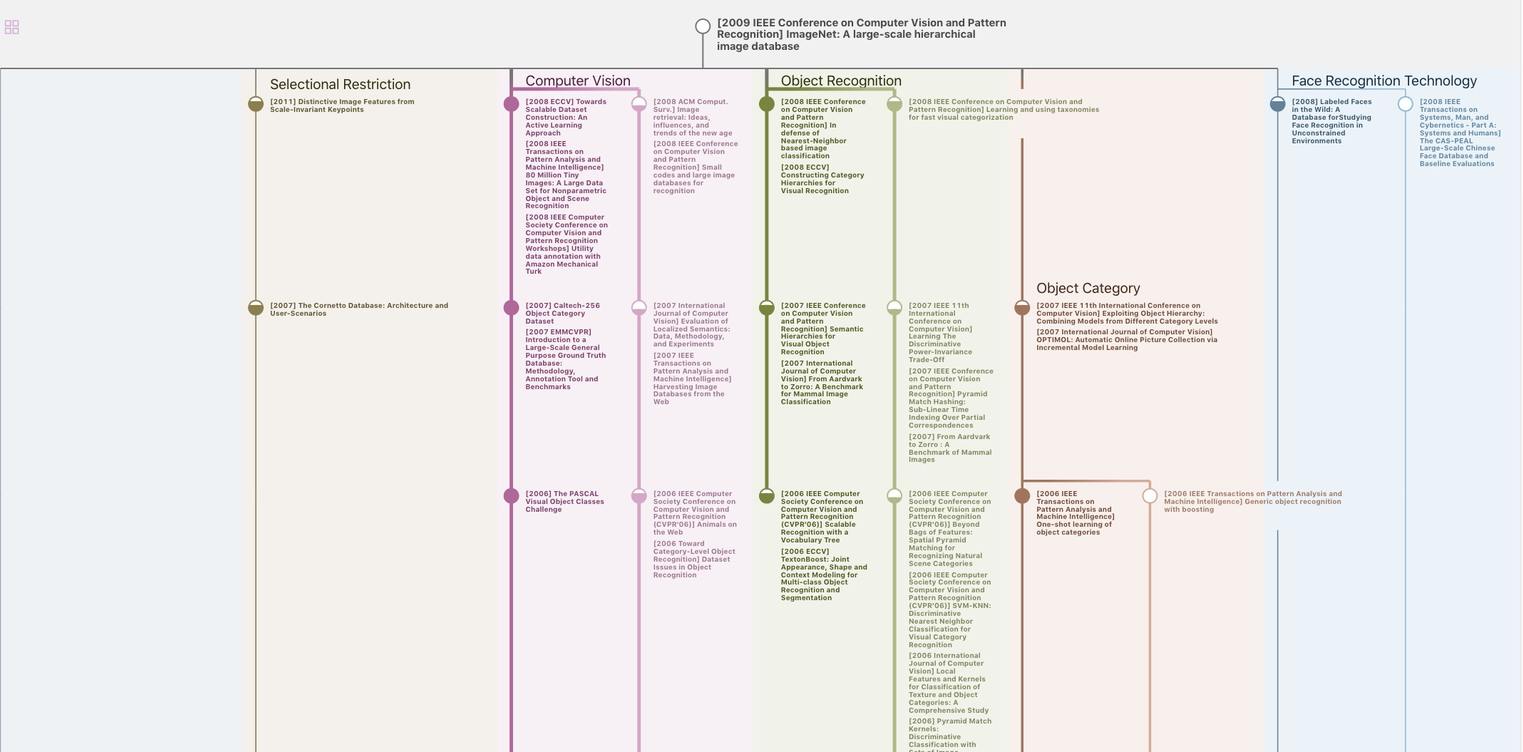
生成溯源树,研究论文发展脉络
Chat Paper
正在生成论文摘要