Zero-Shot Information Extraction as a Unified Text-to-Triple Translation.
EMNLP(2021)
摘要
We cast a suite of information extraction tasks into a text-to-triple translation framework. Instead of solving each task relying on task-specific datasets and models, we formalize the task as a translation between task-specific input text and output triples. By taking the task-specific input, we enable a task-agnostic translation by leveraging the latent knowledge that a pre-trained language model has about the task. We further demonstrate that a simple pre-training task of predicting which relational information corresponds to which input text is an effective way to produce task-specific outputs. This enables the zero-shot transfer of our framework to downstream tasks. We study the zero-shot performance of this framework on open information extraction (OIE2016, NYT, WEB, PENN), relation classification (FewRel and TACRED), and factual probe (Google-RE and T-REx). The model transfers non-trivially to most tasks and is often competitive with a fully supervised method without the need for any task-specific training. For instance, we significantly outperform the F1 score of the supervised open information extraction without needing to use its training set.
更多查看译文
关键词
Information extraction,Language model,Task (project management),F1 score,Natural language processing,Computer science,Translation (geometry),Suite,Zero (linguistics),Simple (abstract algebra),Artificial intelligence
AI 理解论文
溯源树
样例
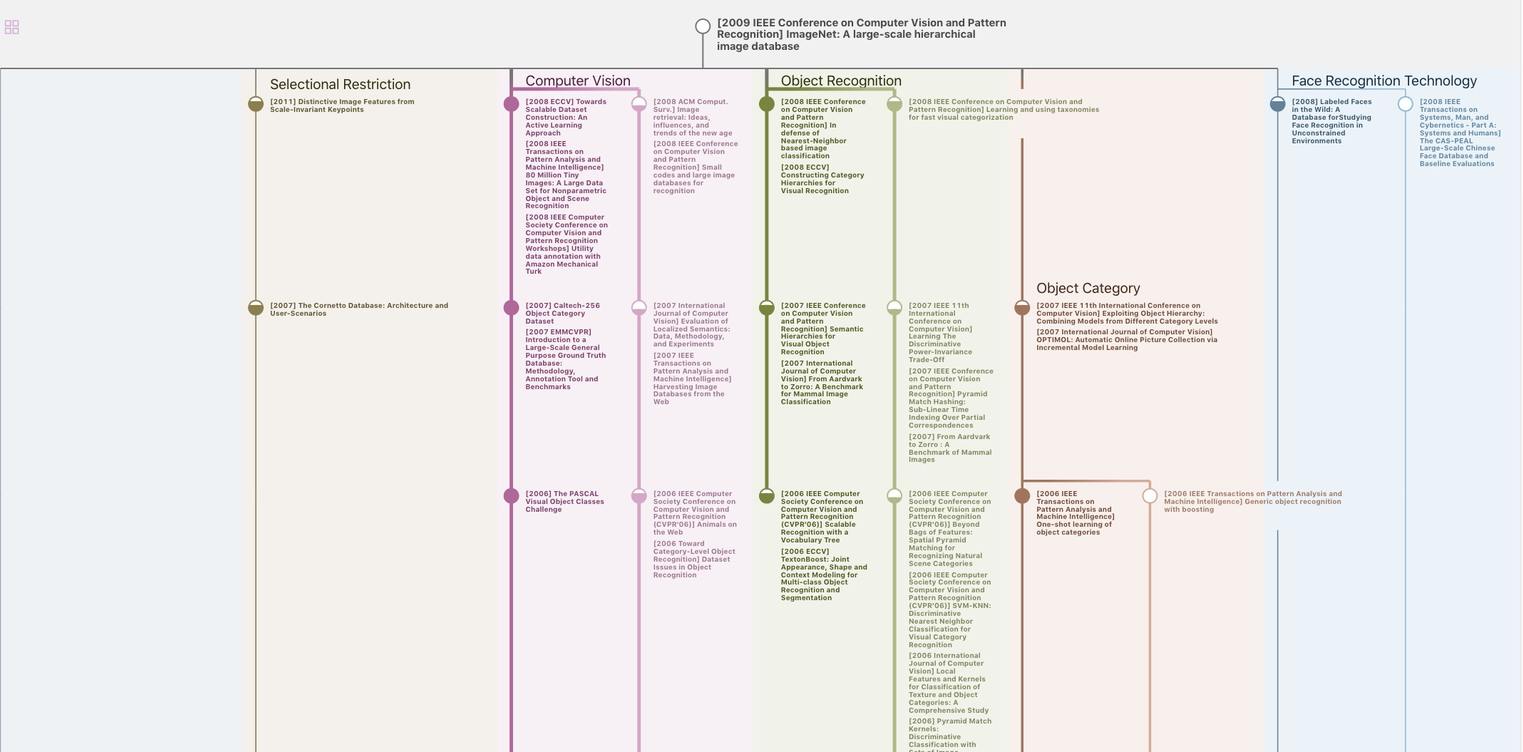
生成溯源树,研究论文发展脉络
Chat Paper
正在生成论文摘要