Image Super-Resolution With Parallel Convolution Attention Network
CONCURRENCY AND COMPUTATION-PRACTICE & EXPERIENCE(2021)
摘要
In recent years, deep convolutional neural networks (CNNs) have achieved a lot of outstanding results in super-resolution with superior ability. However, the majority of CNNs only use a series of convolution kernels with the same size to extract features. This will cause limited receptive fields. In this work, we propose a parallel convolution attention network (PCAN) to extract features in an effective way. Specifically, a pair of parallel convolutions (PCs) with different kernel sizes is used in one layer in our network, which can extract features within different receptive fields, thereby making full use of the multiscale information. Meanwhile, we apply a channel-spatial attention (CSA) module in each parallel convolution block to calculate and fuse channel attention and spatial attention. The obtained attention maps emphasize useful features. Experimental results demonstrate the superiority of our PCAN in comparison with the state-of-the-art methods.
更多查看译文
关键词
attention, parallel convolution, super‐, resolution
AI 理解论文
溯源树
样例
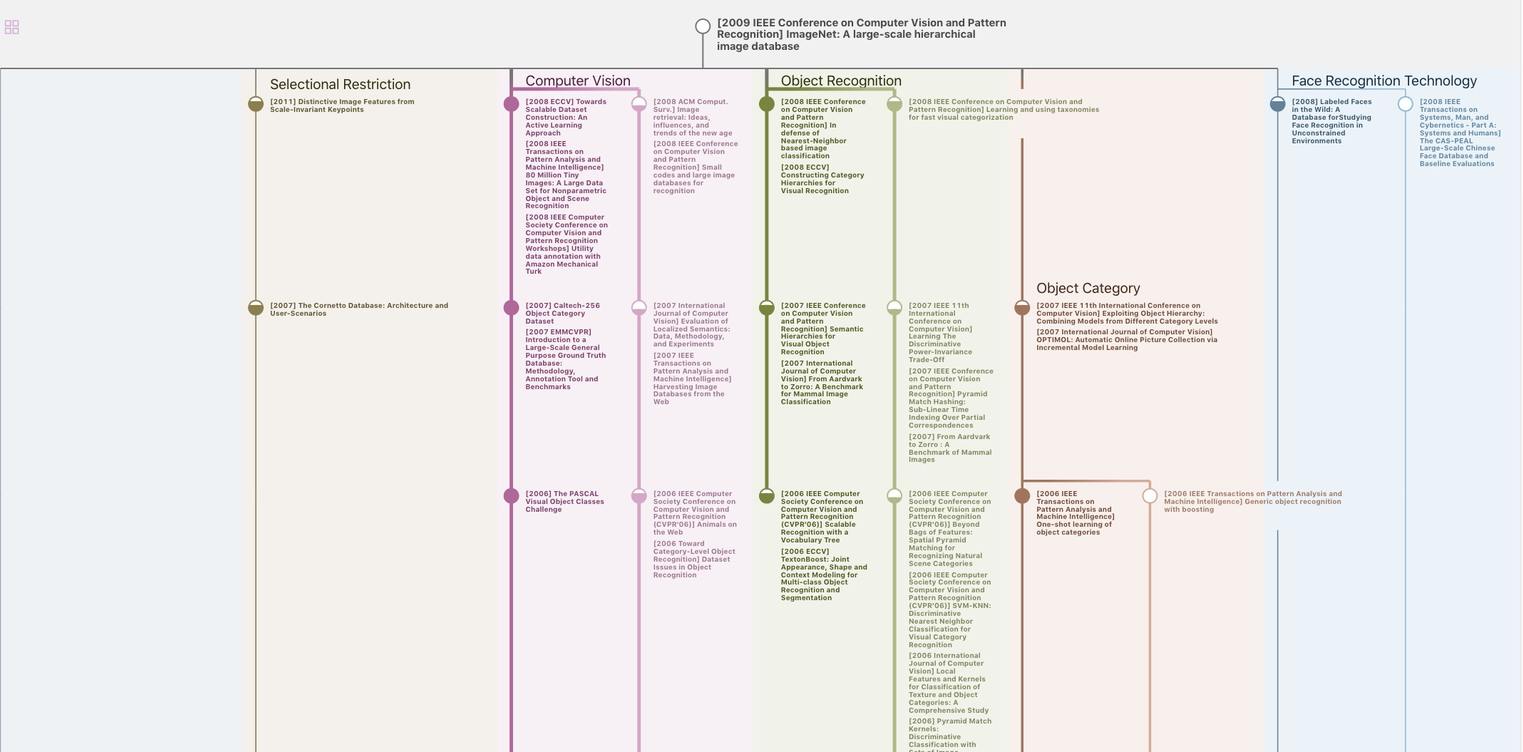
生成溯源树,研究论文发展脉络
Chat Paper
正在生成论文摘要