Improving Spatiotemporal Traffic Prediction in Adversary Weather Conditions Using Hierarchical Bayesian State Space Modeling.
ITSC(2021)
摘要
The significant advancements of the Intelligent Transportation System (ITS) have contributed to the increased development of traffic modeling. These advancements include analytical and prediction models that analyze and predict traffic on highway roads, urban congested networks, as well as estimating travel time delay and road capacity. This study proposes a novel approach for smart traffic prediction models that leverages a Hierarchical Bayesian state-space model framework and incorporates a spatial component to capture the correlation between different dependent road junctions. The model also incorporates weather events as covariates to improve overall predictive accuracy during harsh weather conditions. The proposed model is an extension to the Bayesian hierarchical state-space model for multivariate count series. We derive efficient approximate prediction results where the developed model successfully predicts the peak and trough of traffic congestion index every 15 minutes. Performance evaluation shows that incorporating weather events as covariates in the model provides a significant improvement in the predictive accuracy measured in terms of dynamic time warping distance.
更多查看译文
关键词
Bayesian Networks,Hierarchical Bayesian,Spatiotemporal,Weather events,Traffic Congestion
AI 理解论文
溯源树
样例
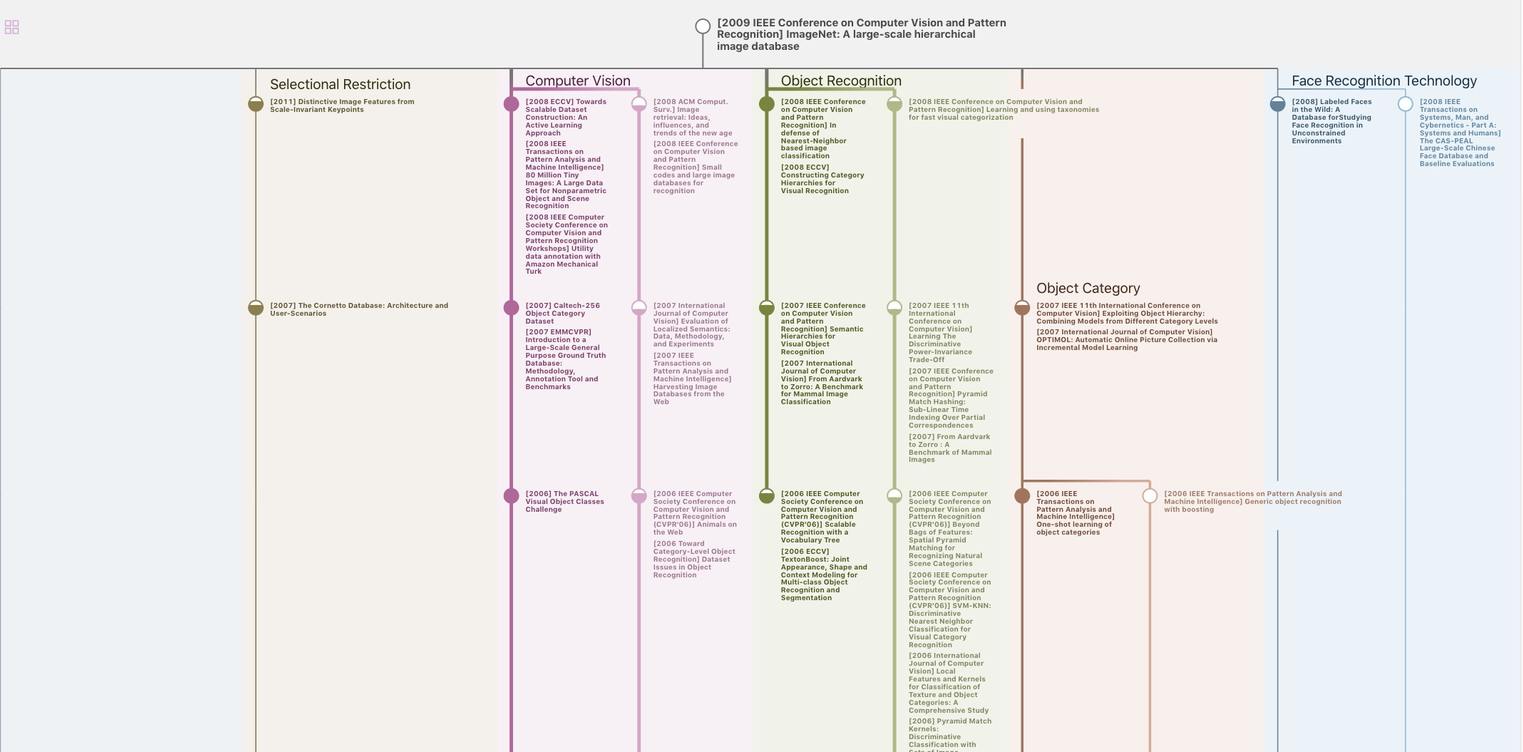
生成溯源树,研究论文发展脉络
Chat Paper
正在生成论文摘要