Trajectory-Based Clustering of Real-World Urban Driving Sequences with Multiple Traffic Objects
ITSC(2021)
摘要
The validation of Advanced Driver Assistance Systems (ADAS) and Automated Driving Systems (ADS) is a major challenge for the automotive industry. Scenario based testing is a promising validation approach, but relies on a scenario database with a high coverage of possible scenarios. Especially in urban areas, the presence of different traffic objects and vulnerable road users (VRU) in combination with semi-structured environments leads to a huge number of possible scenarios. In this paper, a clustering method for grouping real-world driving sequences into semantically similar sequences is introduced. The method allows to deal with the complexity due to the varying number of traffic objects and different sequence lengths and can provide insights over realworld probabilities of driving scenarios. For comparison of different driving sequences, dynamic time warping (DTW) is leveraged. The method is applied to the vehicle trajectories of four urban intersections from the inD dataset and tested with different evaluations. Results show, that an analysis of raw trajectories provides useful insights and is an important component on the path towards a sufficient scenario database.
更多查看译文
关键词
trajectory-based clustering,real-world urban Driving sequences,multiple traffic objects,Advanced Driver Assistance Systems,Automated Driving Systems,automotive industry,scenario based testing,promising validation approach,high coverage,urban areas,different traffic objects,vulnerable road users,semistructured environments,clustering method,real-world driving sequences,semantically similar sequences,different sequence lengths,driving scenarios,different driving sequences,vehicle trajectories,urban intersections,different evaluations,raw trajectories,sufficient scenario database
AI 理解论文
溯源树
样例
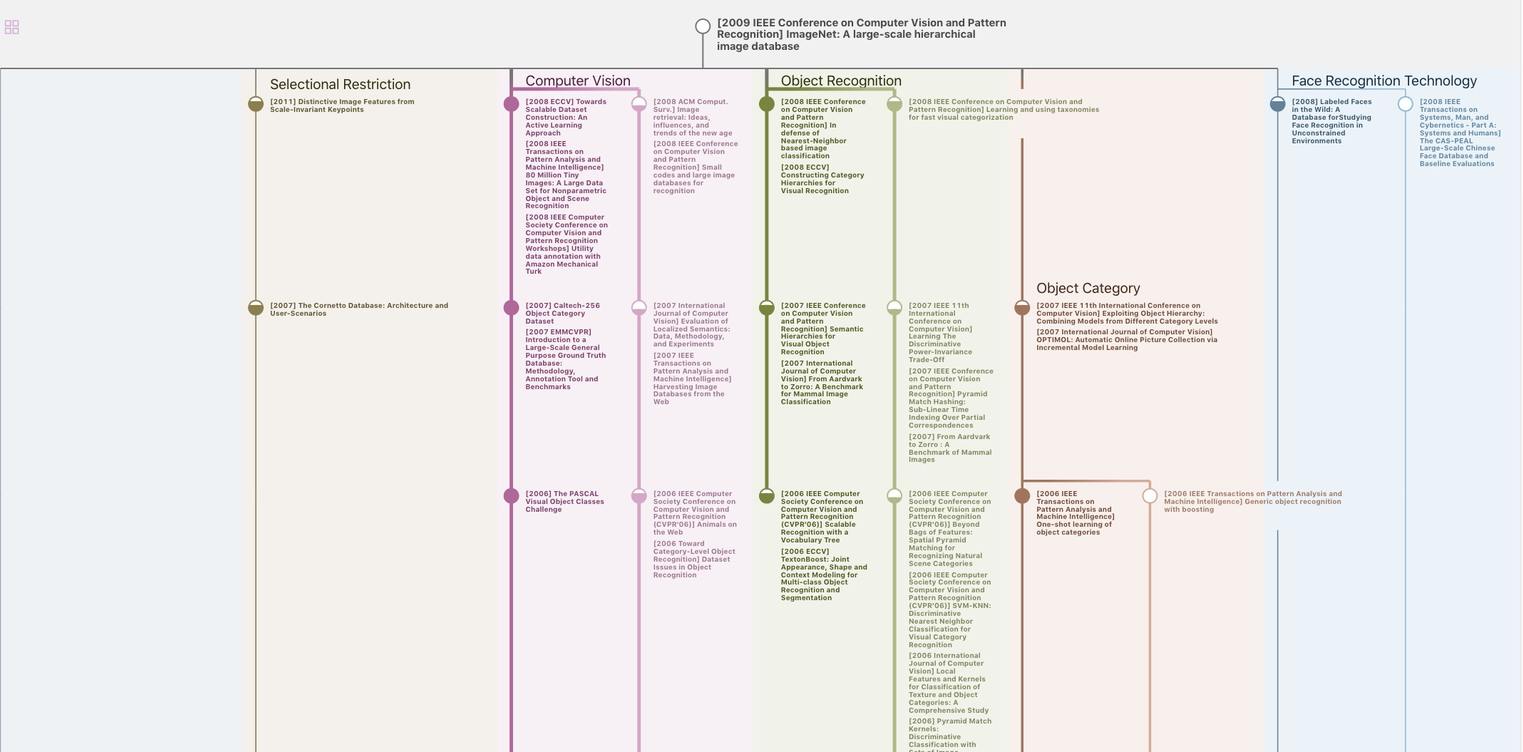
生成溯源树,研究论文发展脉络
Chat Paper
正在生成论文摘要