Ensemble-Based Semi-Supervised Learning to Improve Noisy Soiling Annotations in Autonomous Driving.
ITSC(2021)
摘要
Manual annotation of soiling on surround view cameras is a very challenging and expensive task. The unclear boundary for various soiling categories like water drops or mud particles usually results in a large variance in the annotation quality. As a result, the models trained on such poorly annotated data are far from being optimal. In this paper, we focus on handling such noisy annotations via pseudo-label driven ensemble model which allow us to quickly spot problematic annotations and in most cases also sufficiently fixing them. We train a soiling segmentation model on both noisy and refined labels and demonstrate significant improvements using the refined annotations. It also illustrates that it is possible to effectively refine lower cost coarse annotations.
更多查看译文
关键词
ensemble-based semisupervised,noisy soiling annotations,autonomous driving,surround view cameras,challenging task,expensive task,unclear boundary,soiling categories,water drops,mud particles,annotation quality,poorly annotated data,noisy annotations,pseudolabel driven ensemble model,problematic annotations,soiling segmentation model,noisy labels,refined labels,refined annotations,lower cost coarse annotations
AI 理解论文
溯源树
样例
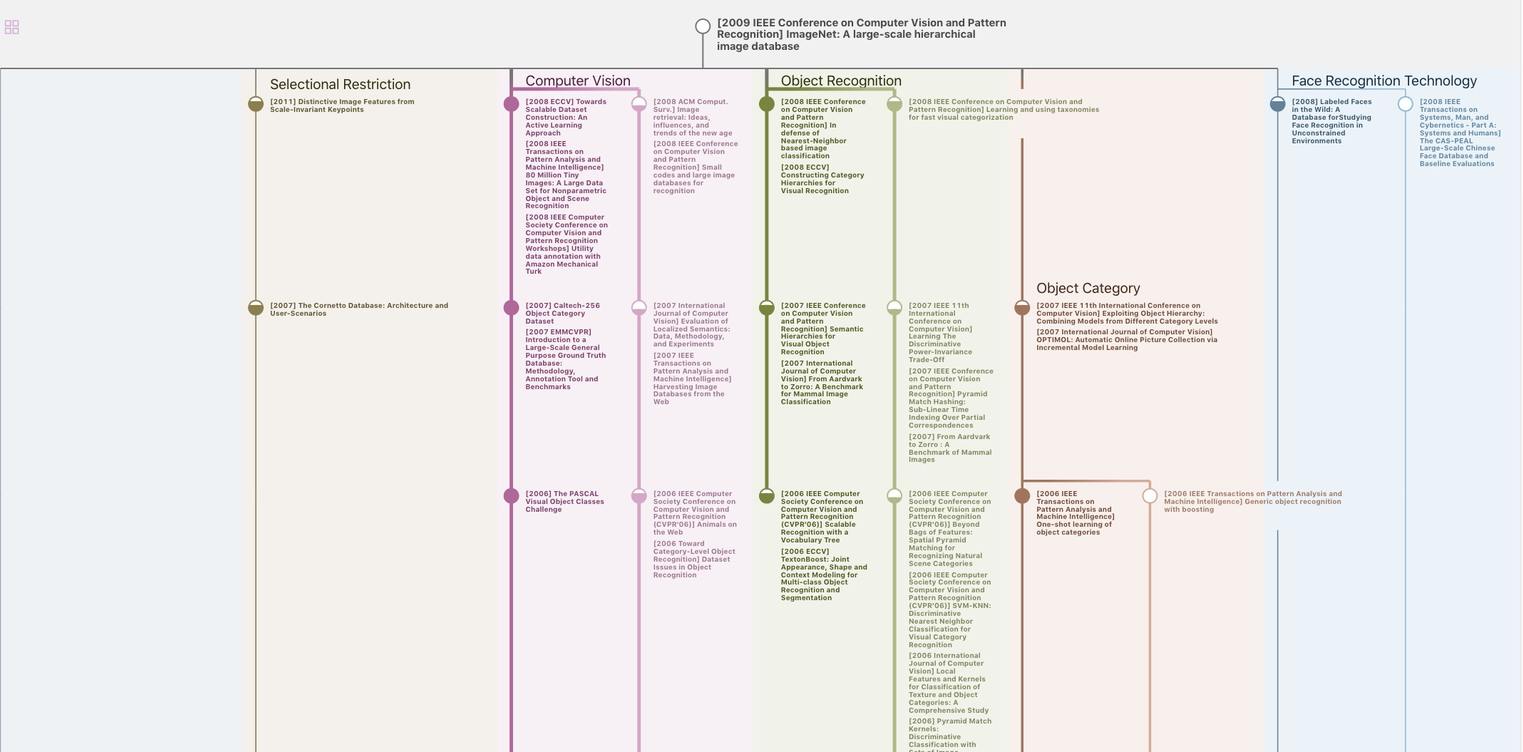
生成溯源树,研究论文发展脉络
Chat Paper
正在生成论文摘要