HPA-Net - Hierarchical and Parallel Aggregation Network for Context Learning in Stereo Matching.
CAIP(2021)
摘要
Accurate disparity estimation with regard to rectified stereo image pairs is essential for many computer vision tasks. Current deep learning-based stereo networks generally construct single-scale cost volume to regularize and regress the disparity. However, these methods do not take advantage of multi-scale context information, leading to the limited performance of disparity prediction in ill-posed regions. In this paper, we propose a novel stereo network named HPA-Net, which provides an efficient representation of context information and lower error rates in ill-posed regions. First, we propose a hierarchical aggregation module to fuse context information from multi-scale cost volumes into an integrated cost volume. Then, we apply the integrated cost volume to the proposed parallel aggregation module, which utilizes several 3D dilated convolutions simultaneously to capture global and local clues of context information for disparity regressions. Experimental results show that our proposed HPA-Net achieves state-of-the-art stereo matching performances on KITTI datasets.
更多查看译文
关键词
stereo,context learning,parallel aggregation network,hpa-net
AI 理解论文
溯源树
样例
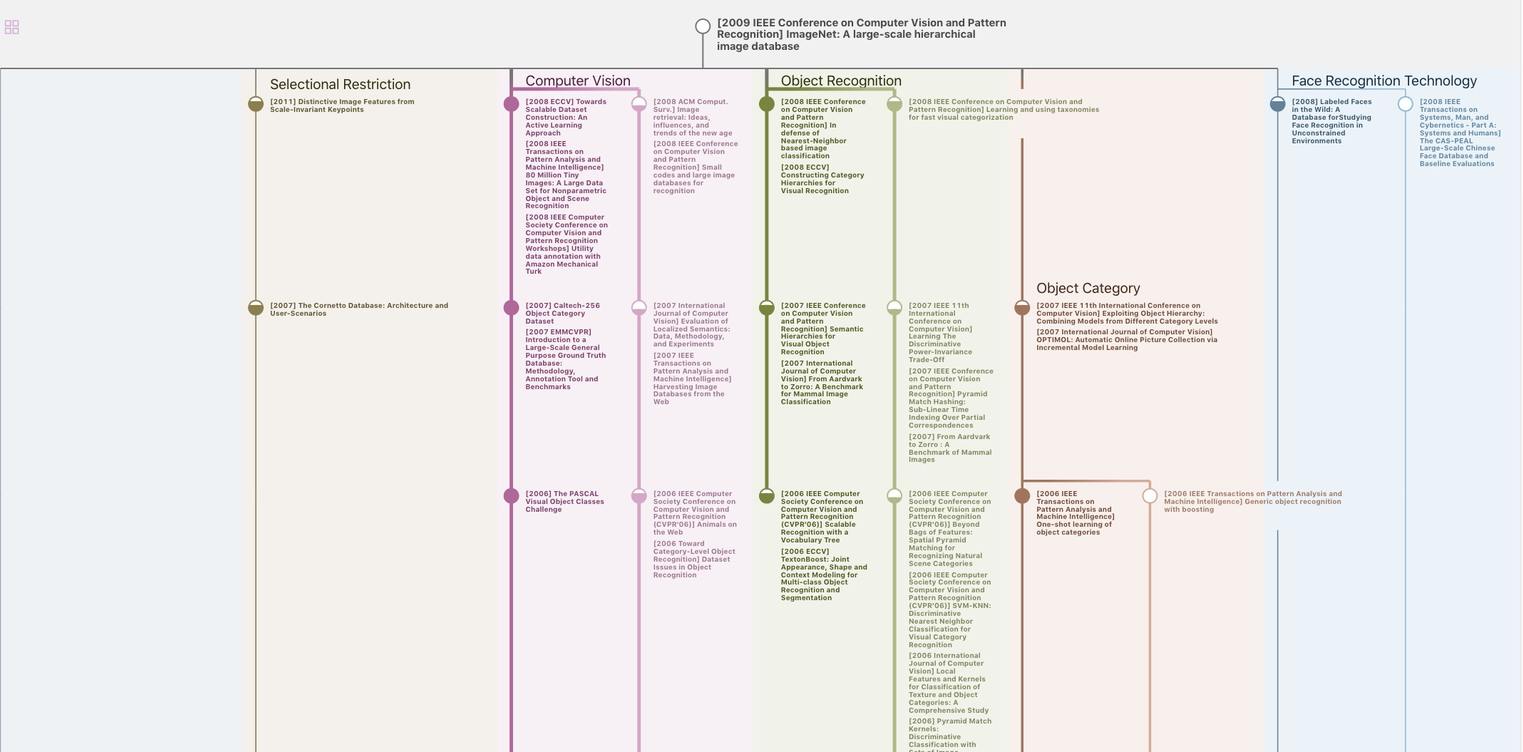
生成溯源树,研究论文发展脉络
Chat Paper
正在生成论文摘要