Covid-19 Chest Radiography Images Analysis Based on Integration of Image Preprocess, Guided Grad-CAM, Machine Learning and Risk Management.
ICMHI(2020)
摘要
COVID19 coronavirus has widely infected more than 10 million people and killed more than 500,000 globally till July 1, 2020. In this paper, we describe a potential methodology, integration of image preprocess, Guided Grad-CAM, machine learning and risk management based on chest radiography images, as one of workable alarm and analysis systems to support clinicians against COVID-19 outbreak threat. We leverage pre-trained CNN models as backbone with further transfer learning to analyze public open datasets composed of 5851 chest radiography images for 4 classes classification, and 15478 images from COVIDx dataset for 3 classes classification, facilitated with steps of ROI and mask, and CNN layer visualization of guided grad-CAM to help CNN focused on critical infection focus in qualitative perspective. In quantitative perspective of 4 classes classification result, accuracy, average sensitivity, average precision, and COVID19 sensitivity of single ResNet50 and our second bagging ensemble model are (77.2%/78.8%/81.9%/100%) and (81.5%/81.4%,86.8%/100%) respectively. Ensemble way of several CNNs and other machine learning methods used here is to contribute about 4% accuracy improvement on top of best single CNN (ResNet50). In our 3 classes classification, those metrics of ensemble model and benchmark are (93.1%/90.1%/89.7%/83%) and (90%/85.9%, 82.4%/77%). We conclude ensemble approach would facilitate weaker classifier more. Beside to accuracy-oriented analysis, a cost minimization approach is suggested here to provide clinicians options of different risk consideration flexibility by trade off among different categories and performance rates.
更多查看译文
AI 理解论文
溯源树
样例
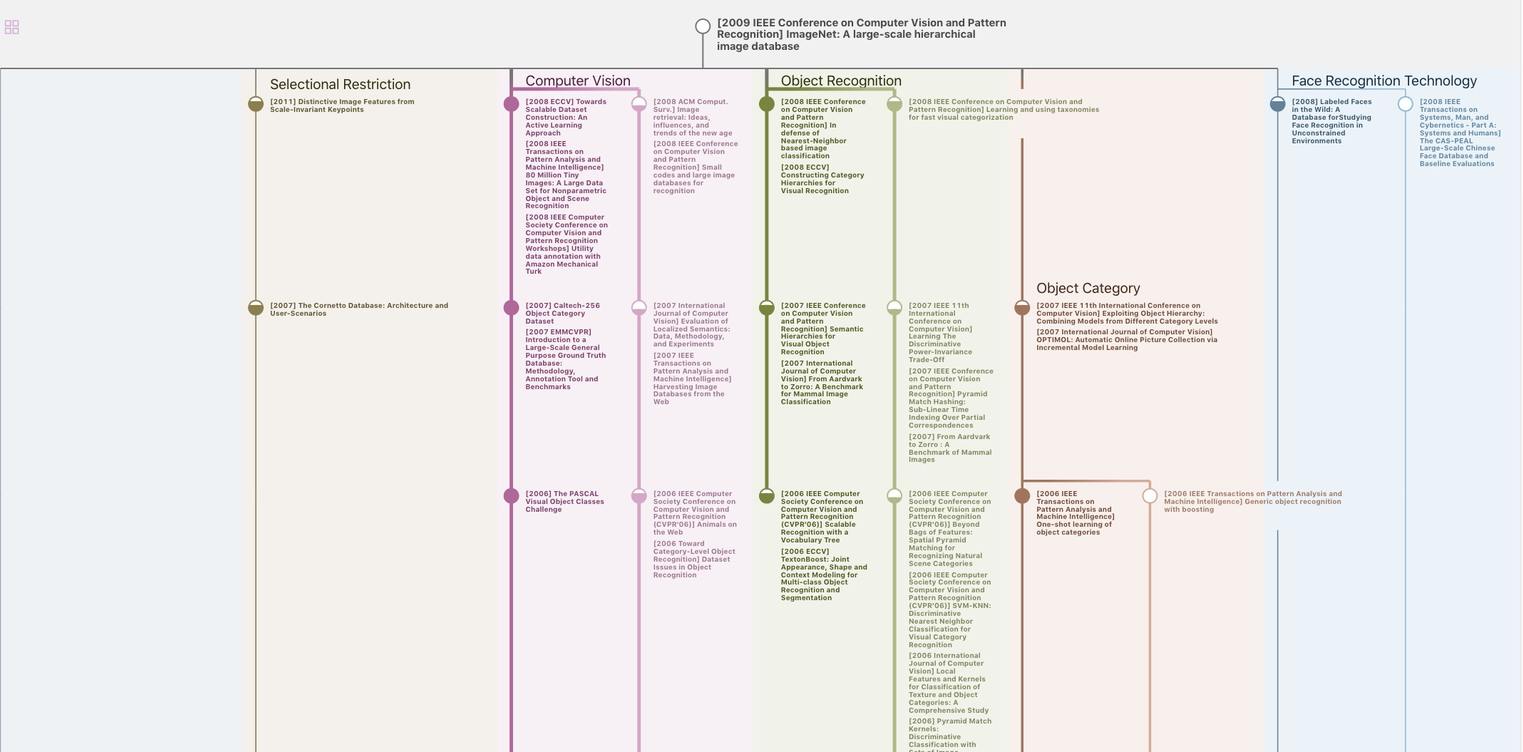
生成溯源树,研究论文发展脉络
Chat Paper
正在生成论文摘要