Augmentations in Graph Contrastive Learning: Current Methodological Flaws & Towards Better Practices
International World Wide Web Conference(2022)
摘要
ABSTRACT Graph classification has a wide range of applications in bioinformatics, social sciences, automated fake news detection, web document classification, and more. In many practical scenarios, including web-scale applications, labels are scarce or hard to obtain. Unsupervised learning is thus a natural paradigm for these settings, but its performance often lags behind that of supervised learning. However, recently contrastive learning (CL) has enabled unsupervised computer vision models to perform comparably to supervised models. Theoretical and empirical works analyzing visual CL frameworks find that leveraging large datasets and task relevant augmentations is essential for CL framework success. Interestingly, graph CL frameworks report high performance while using orders of magnitude smaller data, and employing domain-agnostic graph augmentations (DAGAs) that can corrupt task relevant information. Motivated by these discrepancies, we seek to determine why existing graph CL frameworks continue to perform well, and identify flawed practices in graph data augmentation and popular graph CL evaluation protocols. We find that DAGA can destroy task-relevant information and harm the model’s ability to learn discriminative representations. We also show that on small benchmark datasets, the inductive bias of graph neural networks can significantly compensate for these limitations, while on larger graph classification tasks commonly-used DAGAs perform poorly. Based on our findings, we propose better practices and sanity checks for future research and applications, including adhering to principles in visual CL when designing context-aware graph augmentations. For example, in graph-based document classification, which can be used for better web search, we show task-relevant augmentations improve accuracy by up to 20.
更多查看译文
关键词
Graph Neural Networks, Contrastive Learning, Data Augmentation
AI 理解论文
溯源树
样例
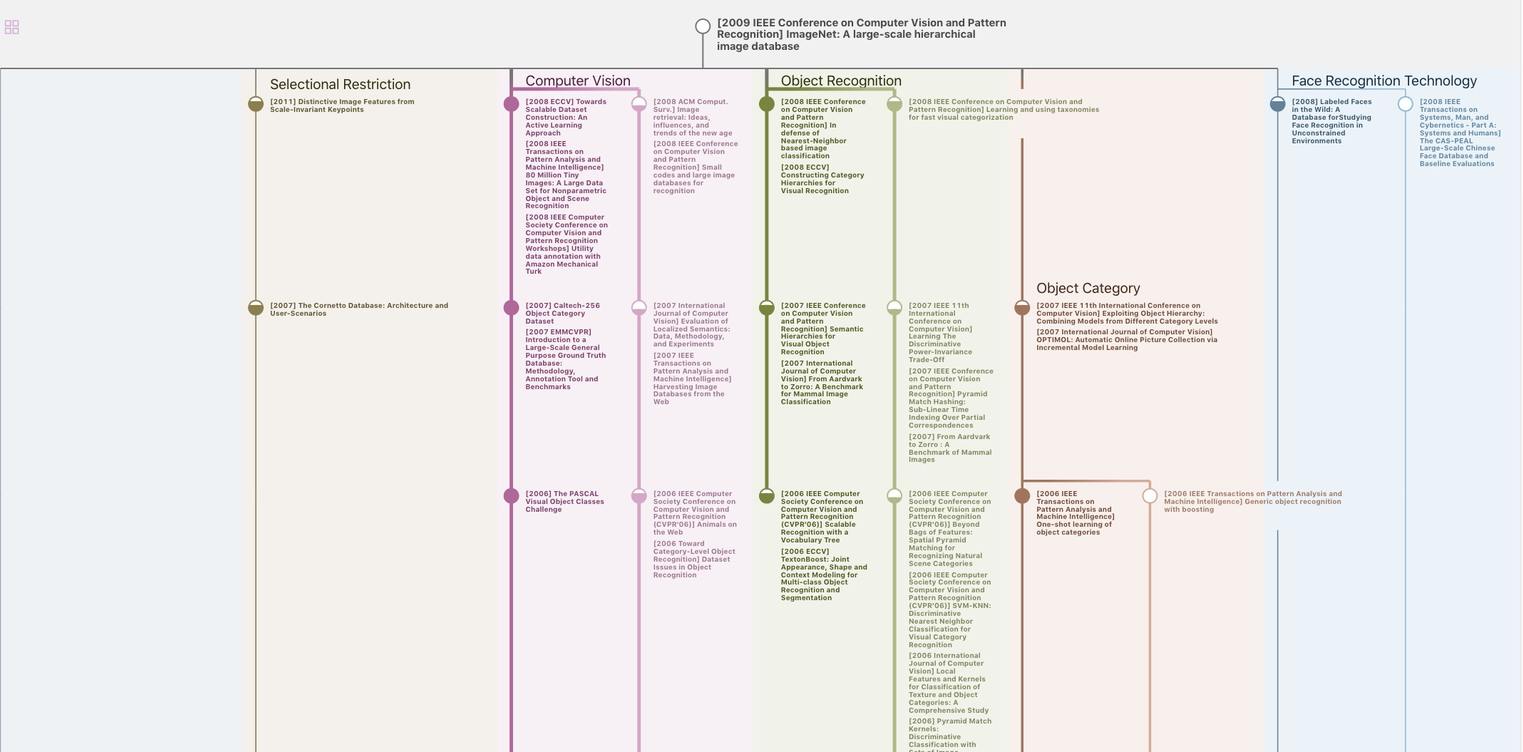
生成溯源树,研究论文发展脉络
Chat Paper
正在生成论文摘要