Understanding Layer-wise Contributions in Deep Neural Networks through Spectral Analysis
arxiv(2021)
摘要
Spectral analysis is a powerful tool, decomposing any function into simpler parts. In machine learning, Mercer's theorem generalizes this idea, providing for any kernel and input distribution a natural basis of functions of increasing frequency. More recently, several works have extended this analysis to deep neural networks through the framework of Neural Tangent Kernel. In this work, we analyze the layer-wise spectral bias of Deep Neural Networks and relate it to the contributions of different layers in the reduction of generalization error for a given target function. We utilize the properties of Hermite polynomials and spherical harmonics to prove that initial layers exhibit a larger bias towards high-frequency functions defined on the unit sphere. We further provide empirical results validating our theory in high dimensional datasets for Deep Neural Networks.
更多查看译文
关键词
deep neural networks,spectral analysis,neural networks,layer-wise
AI 理解论文
溯源树
样例
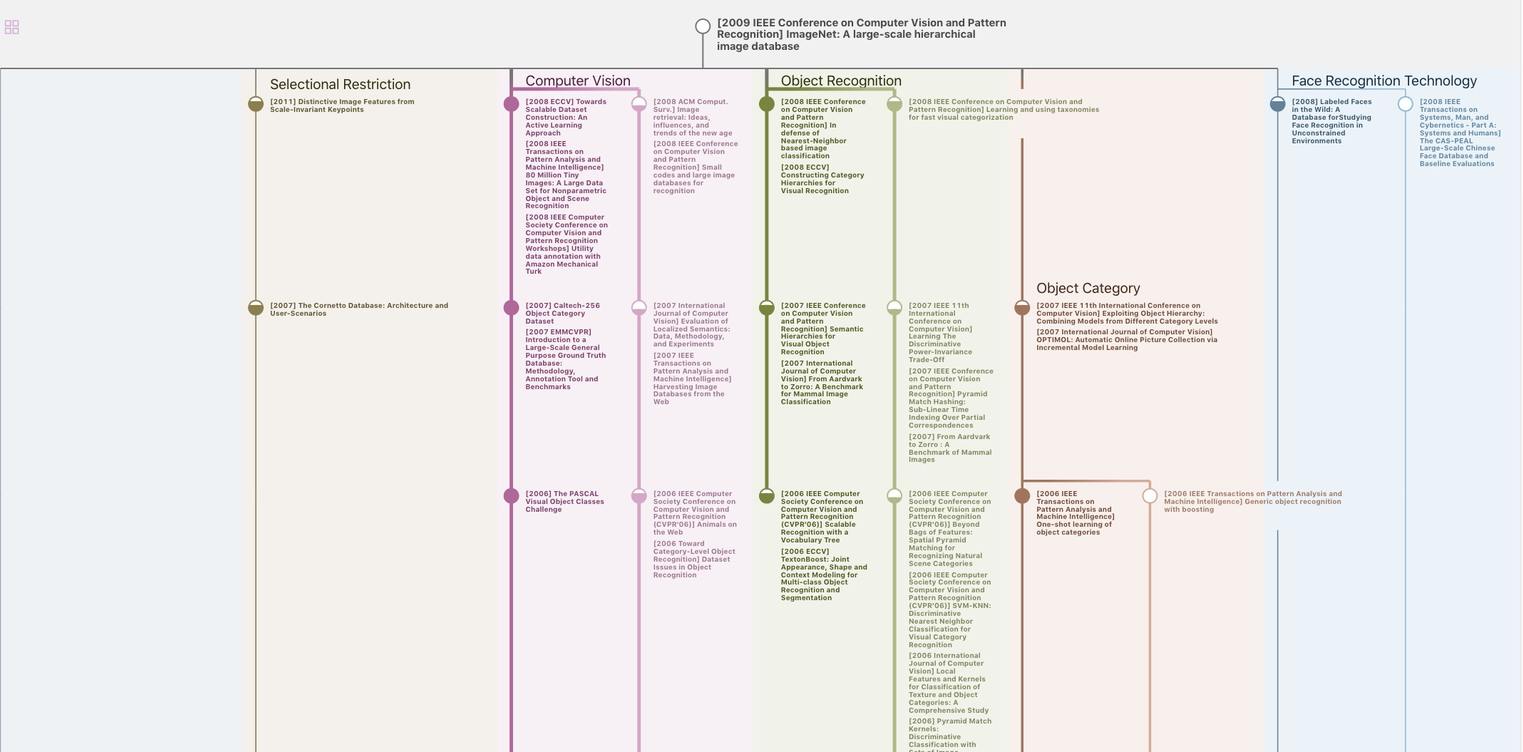
生成溯源树,研究论文发展脉络
Chat Paper
正在生成论文摘要