Open-Set Crowdsourcing using Multiple-Source Transfer Learning
arxiv(2021)
摘要
We raise and define a new crowdsourcing scenario, open set crowdsourcing, where we only know the general theme of an unfamiliar crowdsourcing project, and we don't know its label space, that is, the set of possible labels. This is still a task annotating problem, but the unfamiliarity with the tasks and the label space hampers the modelling of the task and of workers, and also the truth inference. We propose an intuitive solution, OSCrowd. First, OSCrowd integrates crowd theme related datasets into a large source domain to facilitate partial transfer learning to approximate the label space inference of these tasks. Next, it assigns weights to each source domain based on category correlation. After this, it uses multiple-source open set transfer learning to model crowd tasks and assign possible annotations. The label space and annotations given by transfer learning will be used to guide and standardize crowd workers' annotations. We validate OSCrowd in an online scenario, and prove that OSCrowd solves the open set crowdsourcing problem, works better than related crowdsourcing solutions.
更多查看译文
关键词
learning,transfer,open-set,multiple-source
AI 理解论文
溯源树
样例
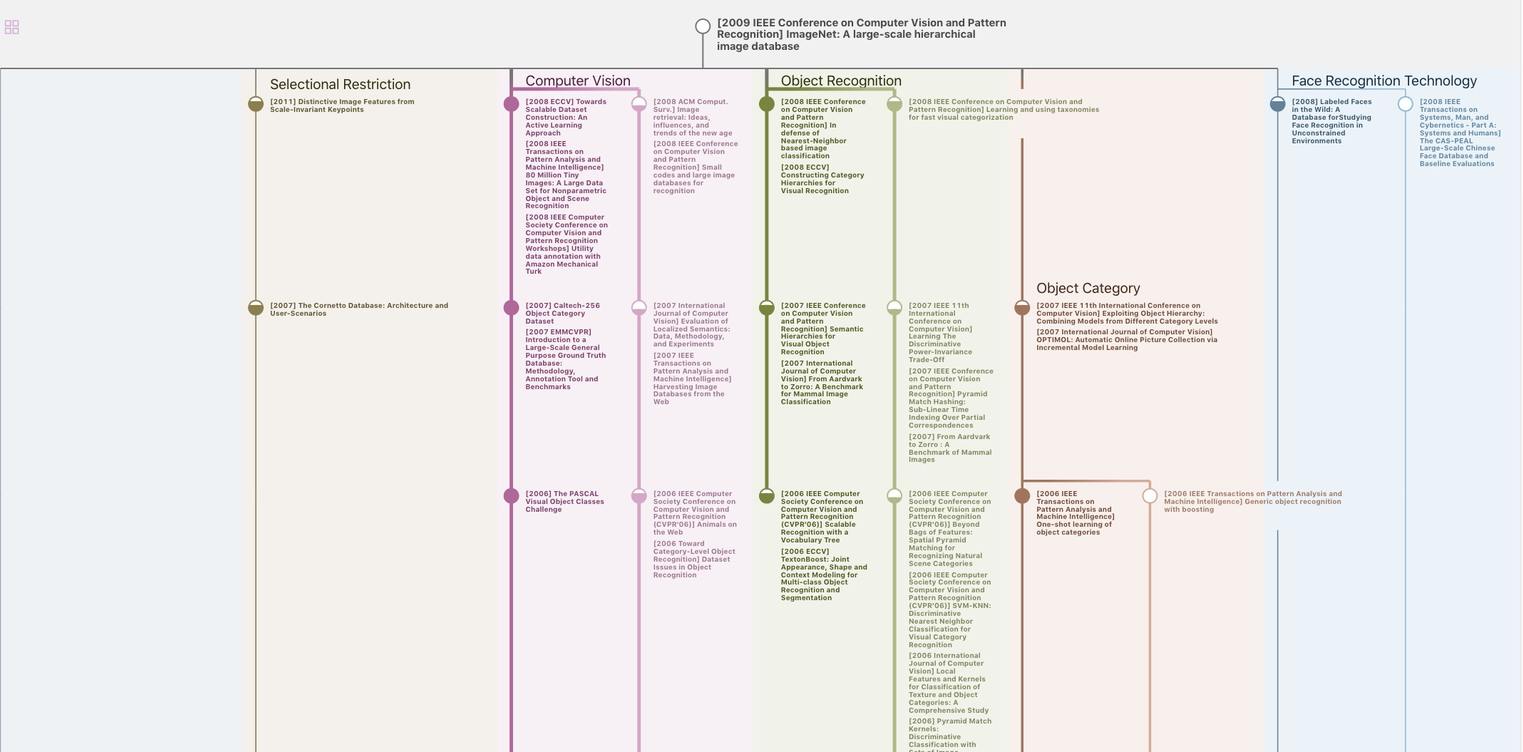
生成溯源树,研究论文发展脉络
Chat Paper
正在生成论文摘要