Rawboost: A Raw Data Boosting and Augmentation Method Applied to Automatic Speaker Verification Anti-Spoofing
IEEE International Conference on Acoustics, Speech, and Signal Processing (ICASSP)(2022)
摘要
This paper introduces RawBoost, a data boosting and augmentation method for the design of more reliable spoofing detection solutions which operate directly upon raw waveform inputs. While RawBoost requires no additional data sources, e.g. noise recordings or impulse responses and is data, application and model agnostic, it is designed for telephony scenarios. Based upon the combination of linear and non-linear convolutive noise, impulsive signal-dependent additive noise and stationary signal-independent additive noise, RawBoost models nuisance variability stemming from, e.g., encoding, transmission, microphones and amplifiers, and both linear and non-linear distortion. Experiments performed using the ASVspoof 2021 logical access database show that RawBoost improves the performance of a state-of-the-art raw end-to-end baseline system by 27% relative and is only outperformed by solutions that either depend on external data or that require additional intervention at the model level.
更多查看译文
关键词
spoofing,presentation attack detection,automatic speaker verification,data augmentation
AI 理解论文
溯源树
样例
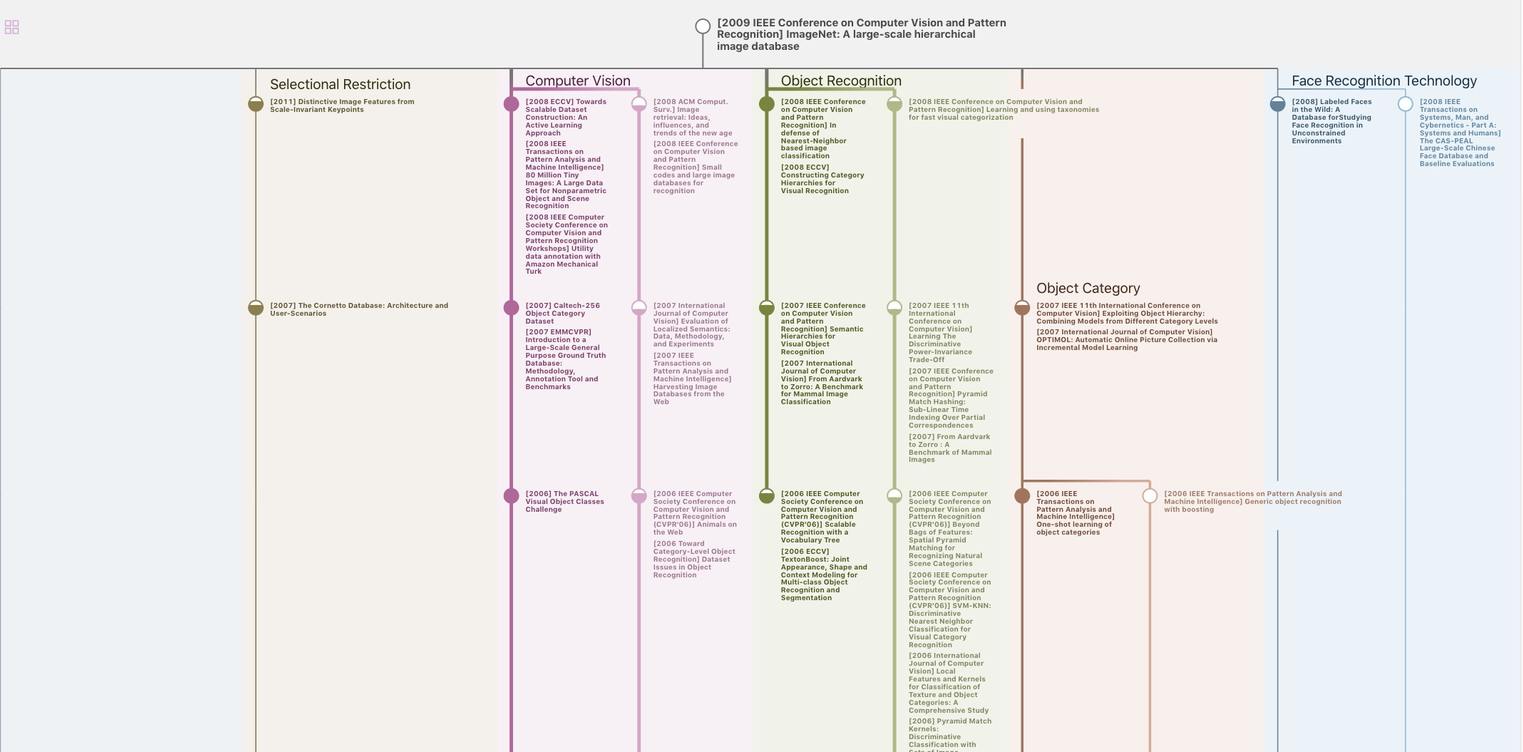
生成溯源树,研究论文发展脉络
Chat Paper
正在生成论文摘要